Kernel Risk Sensitive Loss-based Echo State Networks for Predicting Therapeutic Peptides with Sparse Learning.
BIBM(2022)
Key words
Therapeutic peptides,Kernel risk-sensitive loss,Sparse learning,Protein function,Biological sequence classification
AI Read Science
Must-Reading Tree
Example
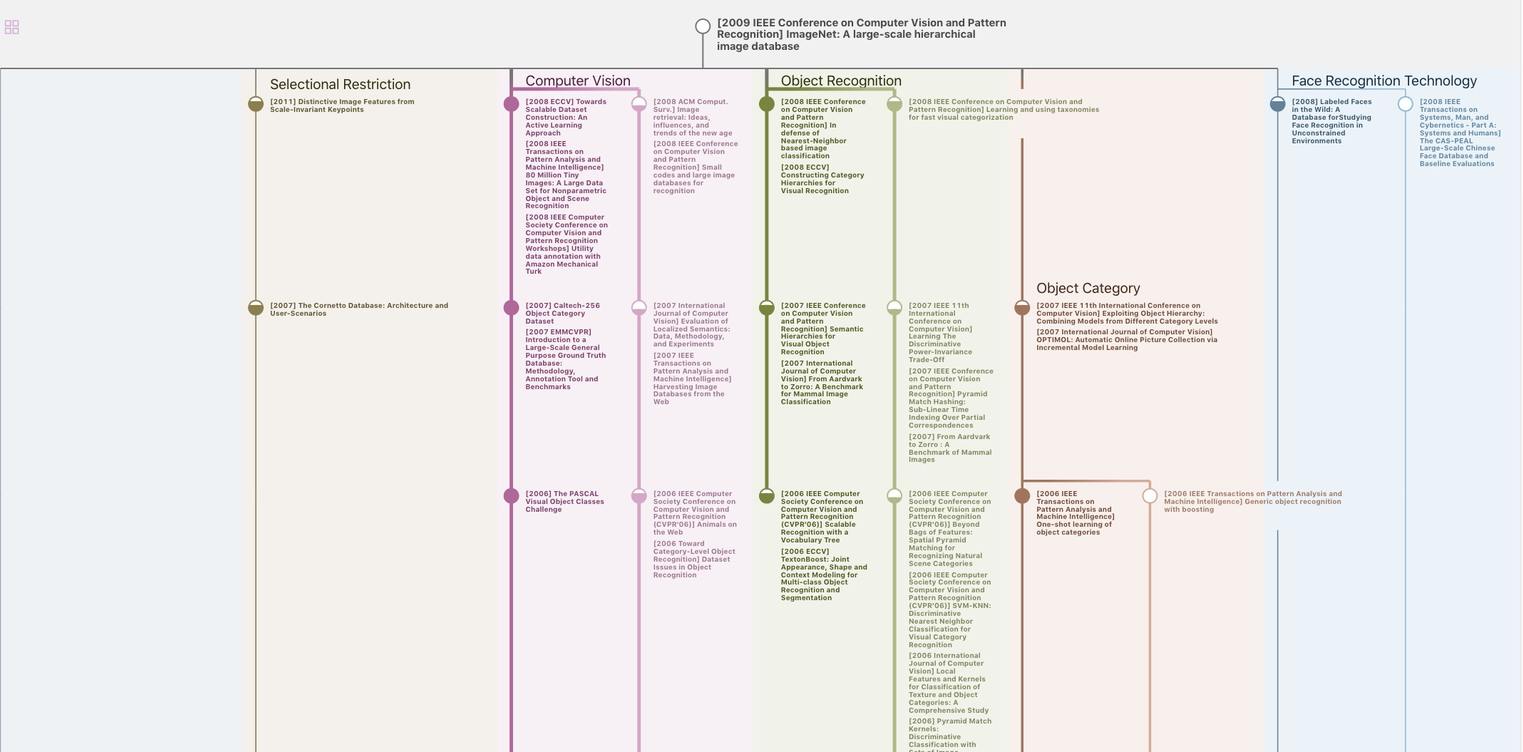
Generate MRT to find the research sequence of this paper
Chat Paper
Summary is being generated by the instructions you defined