HiDDEN: a Machine Learning Method for Detection of Disease-Relevant Populations in Case-Control Single-Cell Transcriptomics Data
NATURE COMMUNICATIONS(2024)
Key words
Droplet-based Sequencing,Single-Cell,Cell Heterogeneity
AI Read Science
Must-Reading Tree
Example
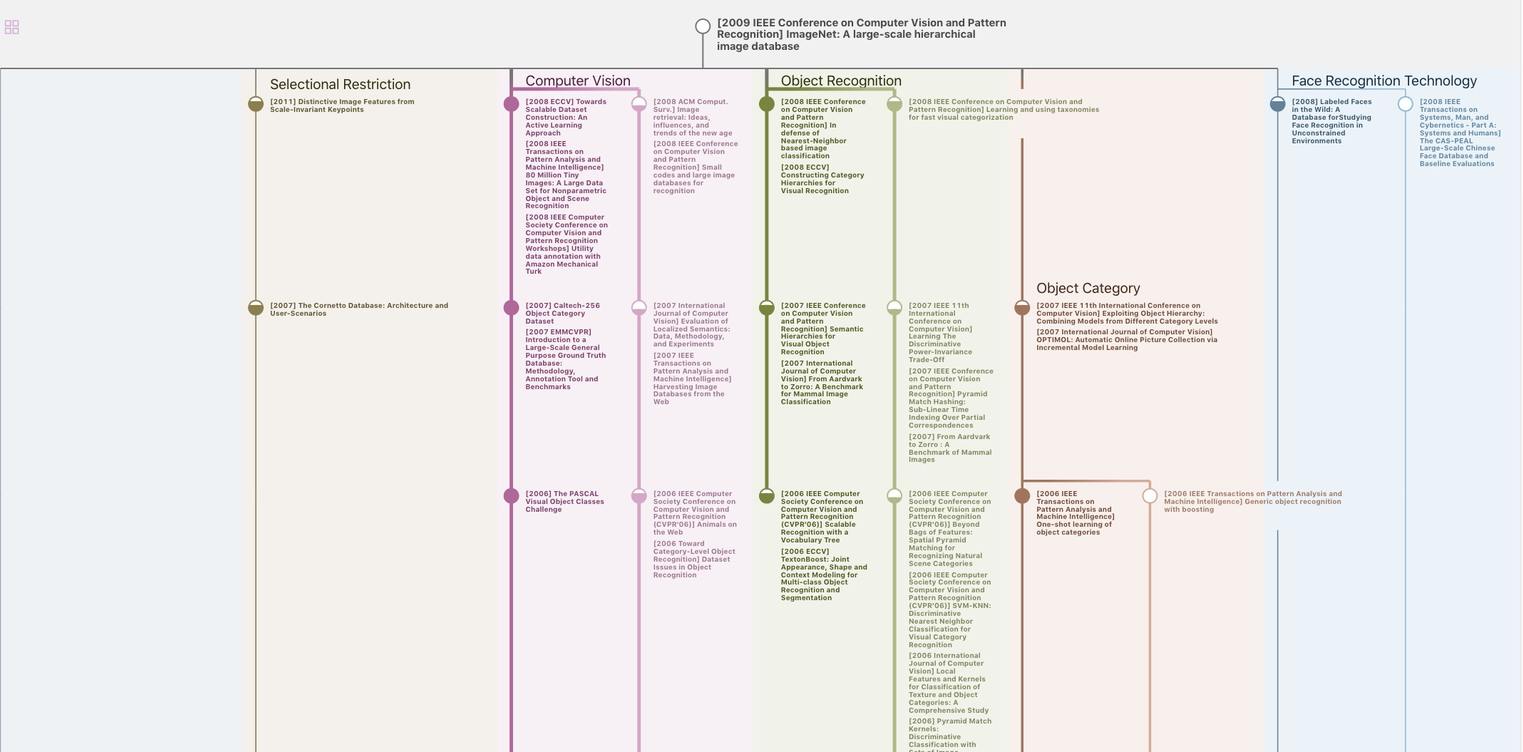
Generate MRT to find the research sequence of this paper
Chat Paper
Summary is being generated by the instructions you defined