Space-Based Mapping of Mangrove Canopy Height with Multi-Sensor Observations and Deep Learning Techniques
IEEE International Geoscience and Remote Sensing Symposium (IGARSS)(2022)
摘要
This study maps canopy height (CH) of mangrove forests using multi-sensor remote-sensing observations with deep learning methods. The multi-sensor data include satellite observations of Synthetic Aperture Radar (SAR) and Landsat-8 optical, and airborne Light Detection and Ranging (LiDAR) data. The study focuses on the south Florida Everglades mangrove forests, which experienced severe damage caused by Hurricane Irma in September 2017. We developed two innovative neural network models to predict CH from SAR and optical data. The models mapped mangrove CH the year following Hurricane Irma with an RMSE of about 3 m. The innovative deep learning methods achieves better accuracy than other traditional machine learning models.
更多查看译文
关键词
multi-sensor, neural network, airborne LiDAR, SAR, optical data, mangrove, deep learning
AI 理解论文
溯源树
样例
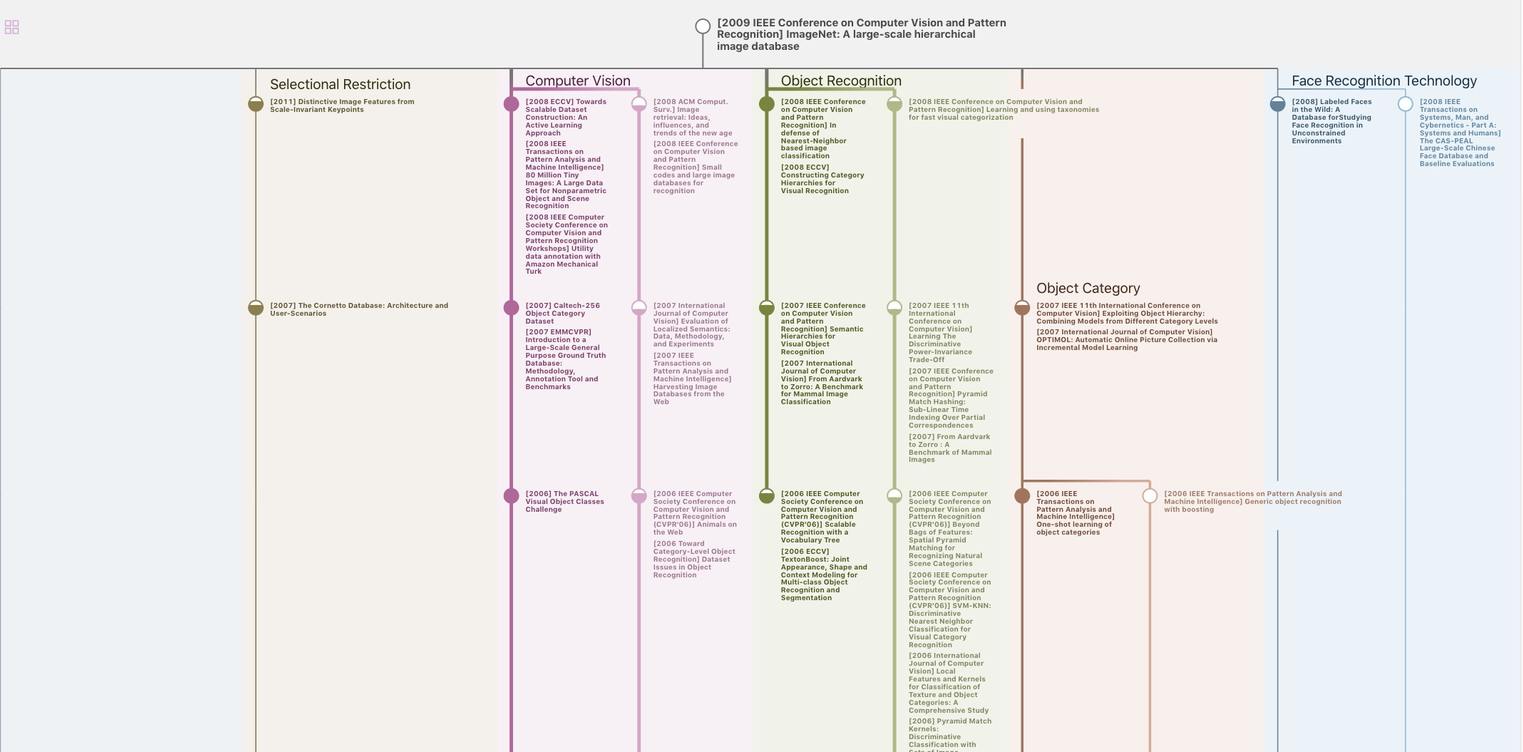
生成溯源树,研究论文发展脉络
Chat Paper
正在生成论文摘要