A New Method for Building Single Feedforward Neural Network Models for Multivariate Static Regression Problems: a Combined Weight Initialization and Constructive Algorithm
Evolutionary Intelligence(2022)
关键词
Regression,Single feedforward neural network,Constructive algorithm,Weight initialization,Linearization
AI 理解论文
溯源树
样例
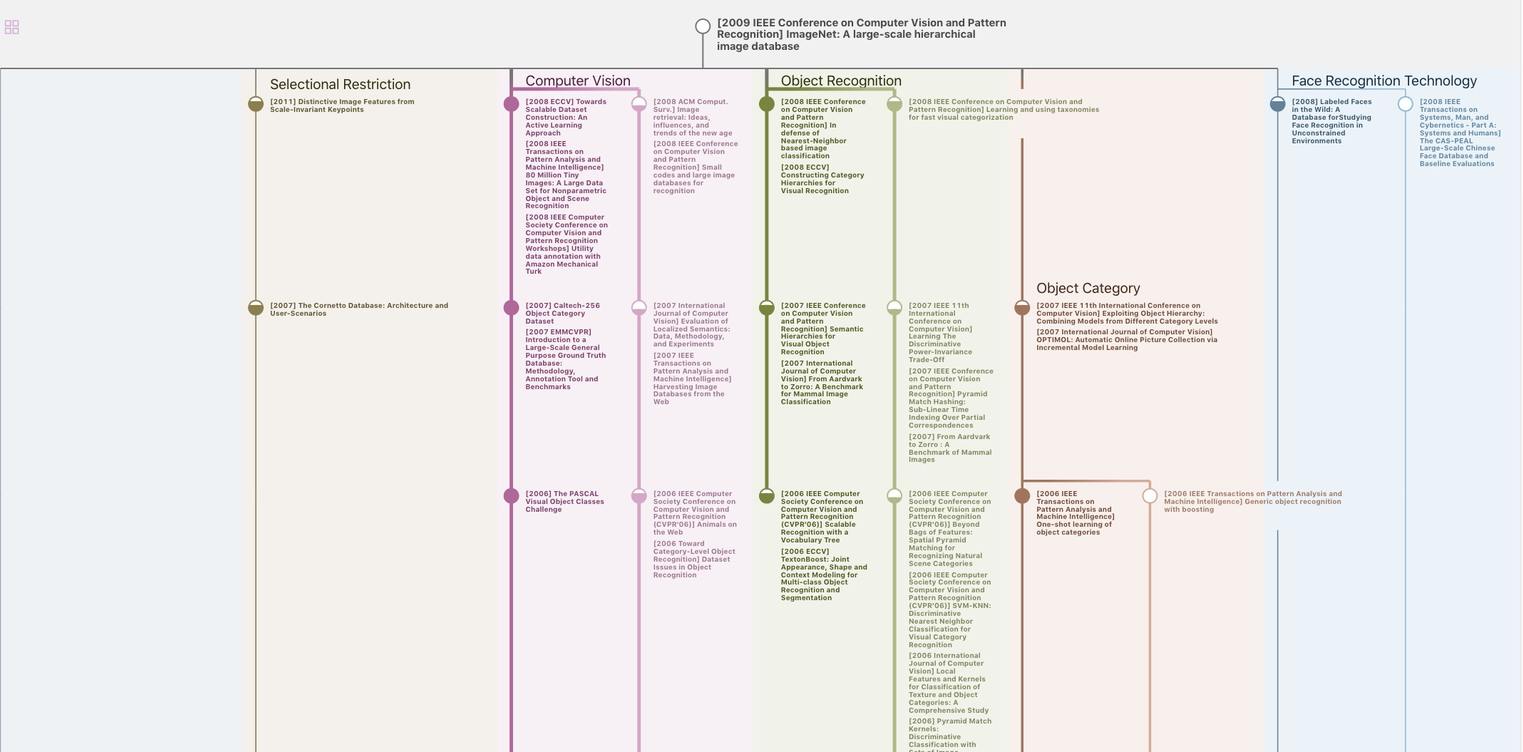
生成溯源树,研究论文发展脉络
Chat Paper
正在生成论文摘要