Hyper-differential Sensitivity Analysis in the Context of Bayesian Inference Applied to Ice-Sheet Problems
INTERNATIONAL JOURNAL FOR UNCERTAINTY QUANTIFICATION(2024)
关键词
hyperdifferential sensitivity analysis,Bayesian inverse problems,inversion
AI 理解论文
溯源树
样例
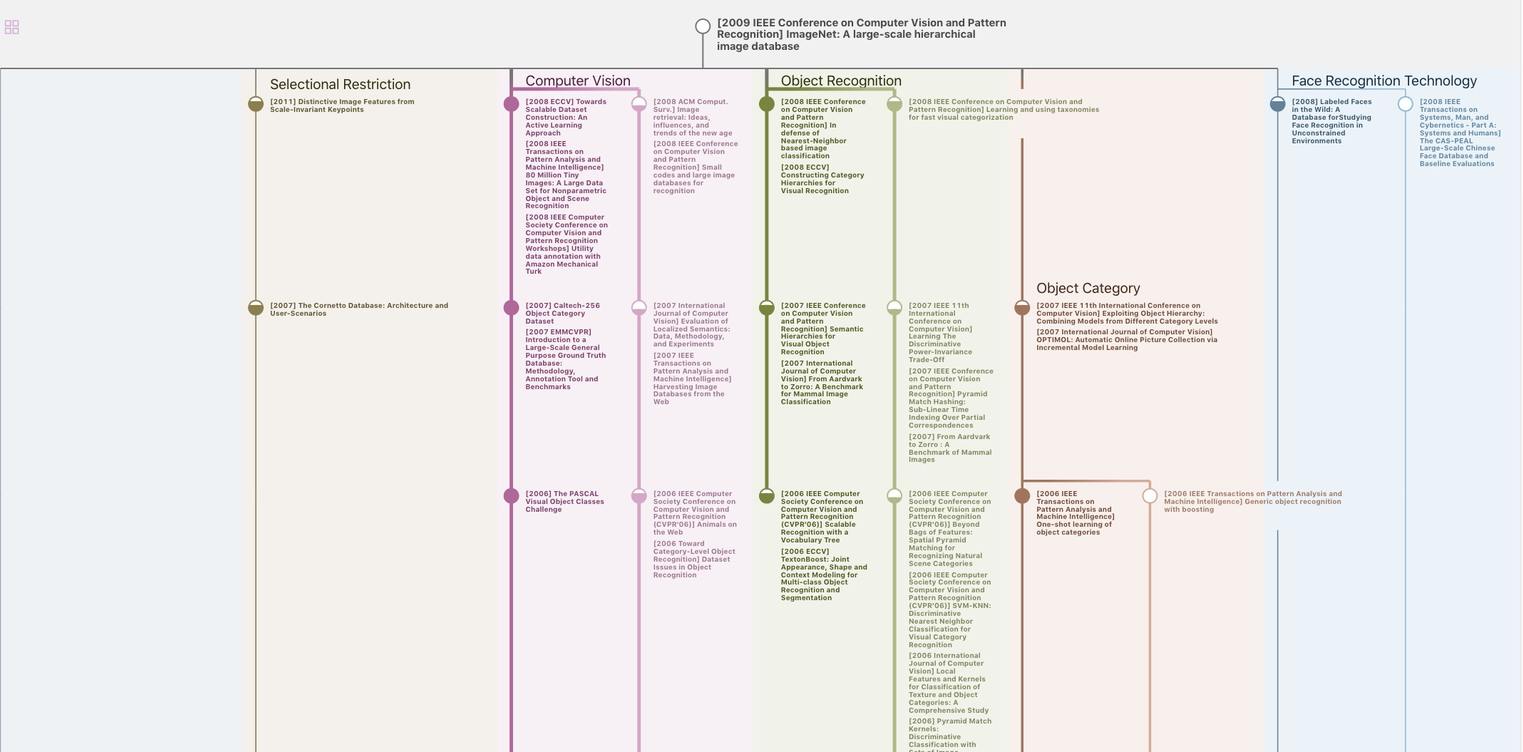
生成溯源树,研究论文发展脉络
Chat Paper
正在生成论文摘要