Intelligent Fault Diagnosis for Inertial Measurement Unit through Deep Residual Convolutional Neural Network and Short-Time Fourier Transform
MACHINES(2022)
摘要
An Inertial Measurement Unit (IMU) is a significant component of a spacecraft, and its fault diagnosis results directly affect the spacecraft's stability and reliability. In recent years, deep learning-based fault diagnosis methods have made great achievements; however, some problems such as how to extract effective fault features and how to promote the training process of deep networks are still to be solved. Therefore, in this study, a novel intelligent fault diagnosis approach combining a deep residual convolutional neural network (CNN) and a data preprocessing algorithm is proposed. Firstly, the short-time Fourier transform (STFT) is adopted to transform the raw time domain data into time-frequency images so the useful information and features can be extracted. Then, the Z-score normalization and data augmentation strategies are both explored and exploited to facilitate the training of the subsequent deep model. Furthermore, a modified CNN-based deep diagnosis model, which utilizes the Parameter Rectified Linear Unit (PReLU) as activation functions and residual blocks, automatically learns fault features and classifies fault types. Finally, the experiment's results indicate that the proposed method has good fault features' extraction ability and performs better than other baseline models in terms of classification accuracy.
更多查看译文
关键词
IMU, deep learning, residual network, fault diagnosis
AI 理解论文
溯源树
样例
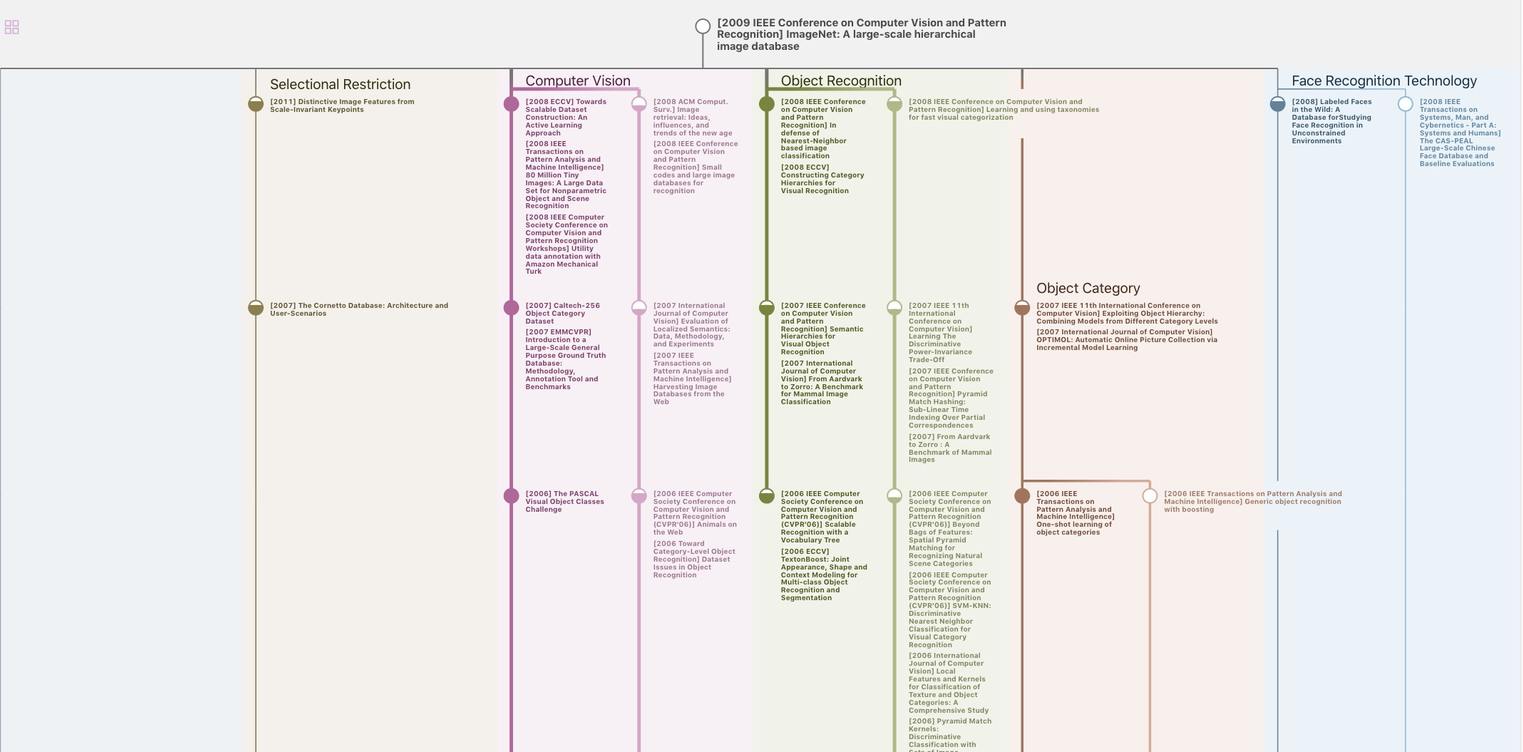
生成溯源树,研究论文发展脉络
Chat Paper
正在生成论文摘要