Feature Selection Assists BLSTM for the Ultrasensitive Detection of Bioflavonoids in Different Biological Matrices Based on the 3D Fluorescence Spectra of Gold Nanoclusters
ANALYTICAL CHEMISTRY(2022)
AI 理解论文
溯源树
样例
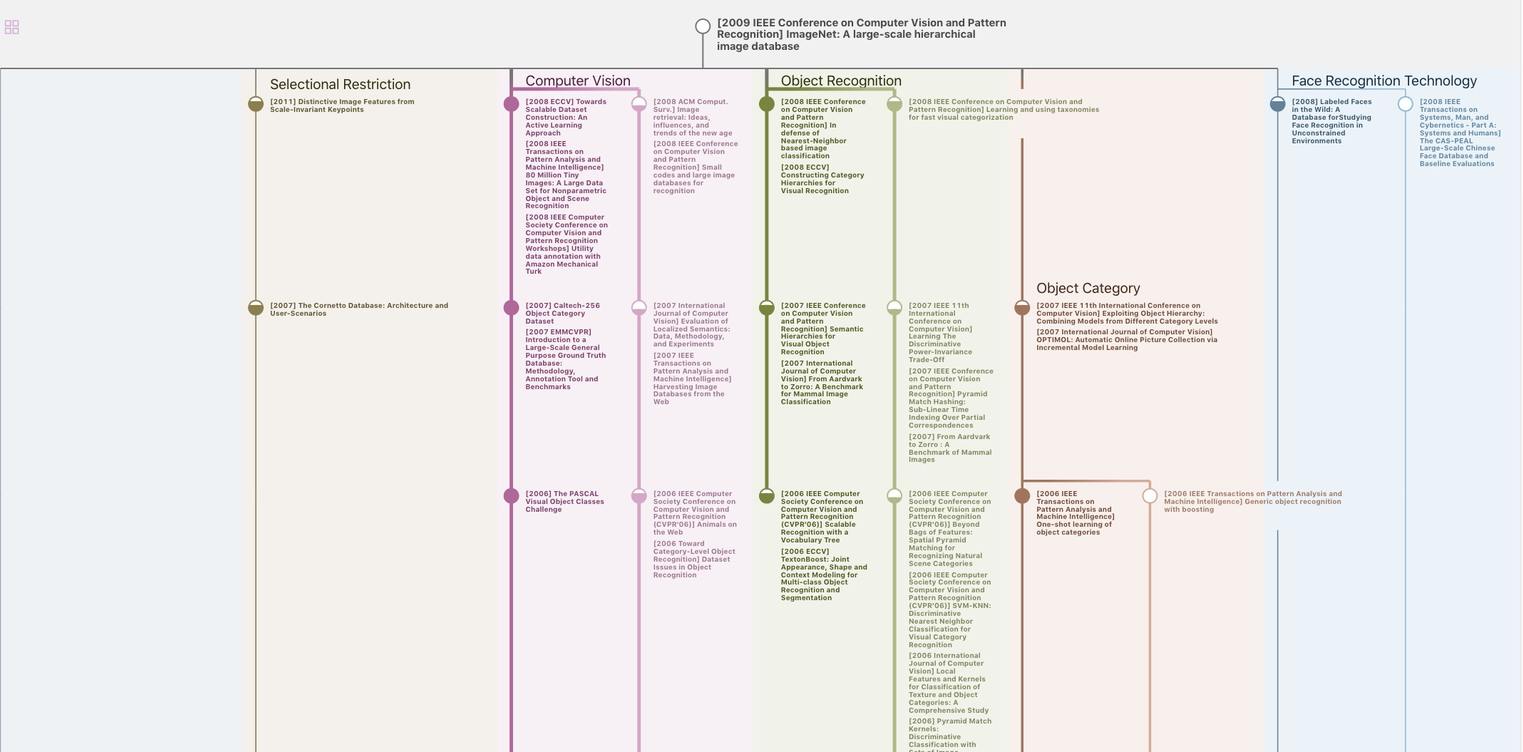
生成溯源树,研究论文发展脉络
Chat Paper
正在生成论文摘要
ANALYTICAL CHEMISTRY(2022)