Data-Driven Inference of Synthesis Guidelines for High-Performance Zeolite-Based Selective Catalytic Reduction Catalysts at Low Temperatures
CHEMISTRY OF MATERIALS
摘要
Numerous zeolite-based selective catalytic reduc-tion (SCR) catalysts have been investigated to improve nitrogen oxide (NOx) removal efficiency at low temperatures of 25-200 degrees C in diesel vehicles. However, the majority of these studies examined only one of each feature's effects. The catalysis mechanism consists of complex reactions, and the various features interact, making it difficult to predict their combinatorial effects on the catalytic activity. Recently, machine learning-based models have been widely employed in catalysis science to infer hidden information about catalysts without knowledge of the underlying physical principles. Interpretable machine learning models are particularly useful for catalyst research because they can explain the causal relationship between characteristics and catalytic performance. In this study, we construct a machine learning model utilizing a decision tree, one of the representative interpretable machine learning models. Using this model, we evaluate the causal relationship between features and the NOx removal efficiency of zeolite-based SCR catalysts at low temperatures, which is difficult to deduce due to the high number of features. Additionally, we extract several synthesis guidelines for catalysts that show superior NOx removal performance at low temperatures. New catalysts were synthesized using the proposed rules, and their performance was validated experimentally.
更多查看译文
关键词
selective catalytic reduction catalysts,synthesis guidelines,data-driven,high-performance,zeolite-based
AI 理解论文
溯源树
样例
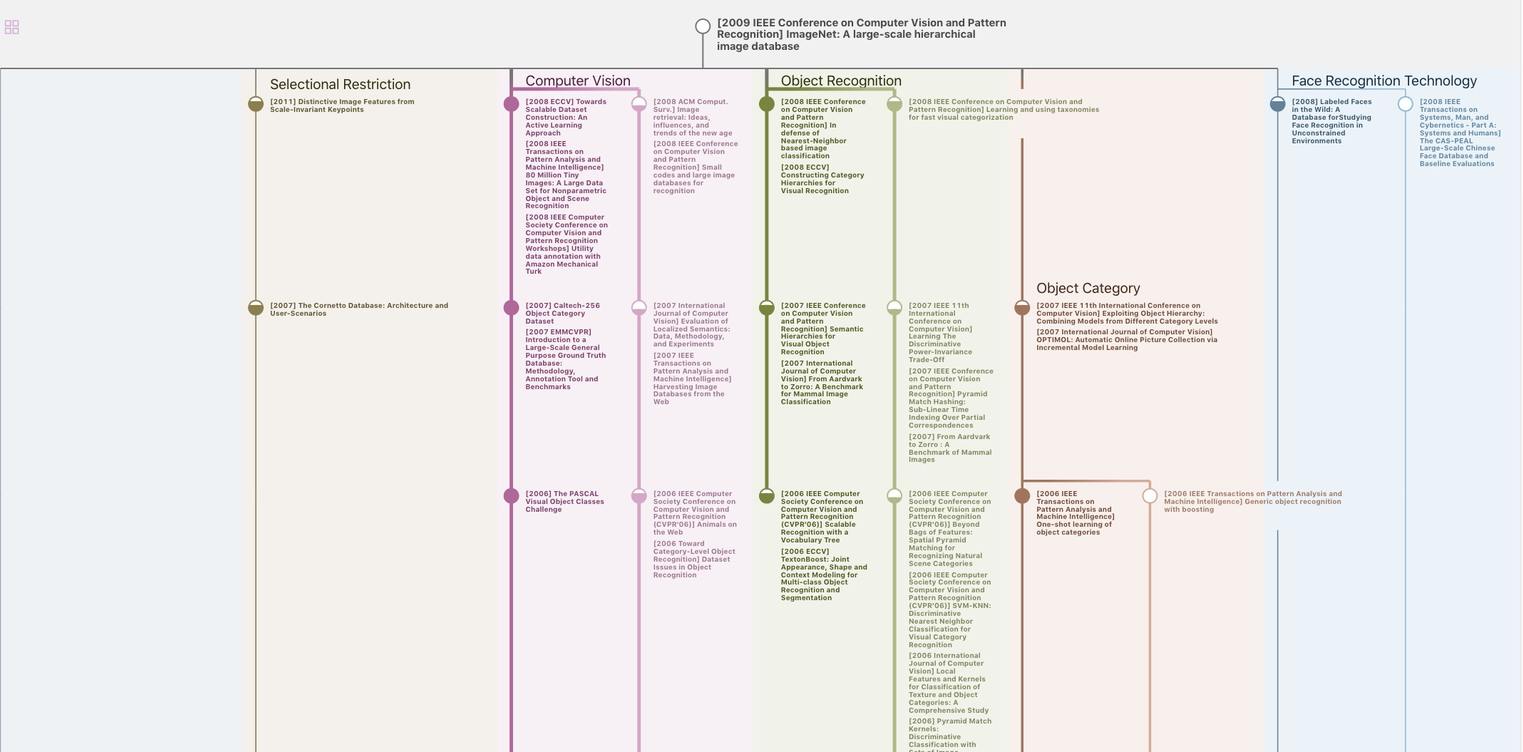
生成溯源树,研究论文发展脉络
Chat Paper
正在生成论文摘要