3D Reconstruction and Semantic Segmentation Method Combining PointNet and 3D-Lmnet from Single Image
LASER & OPTOELECTRONICS PROGRESS(2022)
关键词
deep learning,single image,joint optimization,3D reconstruction,semantic segmentation
AI 理解论文
溯源树
样例
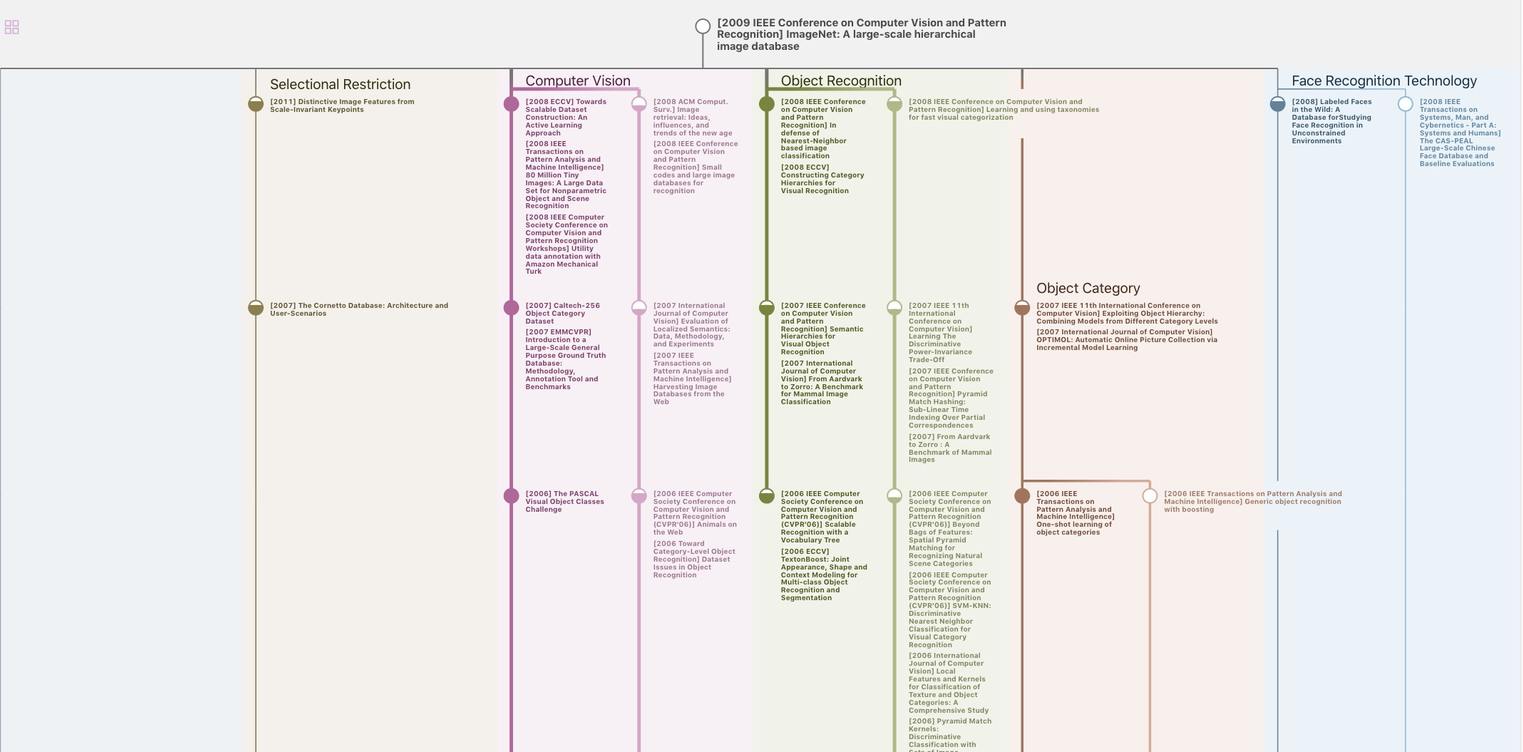
生成溯源树,研究论文发展脉络
Chat Paper
正在生成论文摘要