CMU-Net: A Strong ConvMixer-based Medical Ultrasound Image Segmentation Network
arxiv(2022)
摘要
U-Net and its extensions have achieved great success in medical image segmentation. However, due to the inherent local characteristics of ordinary convolution operations, U-Net encoder cannot effectively extract global context information. In addition, simple skip connections cannot capture salient features. In this work, we propose a fully convolutional segmentation network (CMU-Net) which incorporates hybrid convolutions and multi-scale attention gate. The ConvMixer module extracts global context information by mixing features at distant spatial locations. Moreover, the multi-scale attention gate emphasizes valuable features and achieves efficient skip connections. We evaluate the proposed method using both breast ultrasound datasets and a thyroid ultrasound image dataset; and CMU-Net achieves average Intersection over Union (IoU) values of 73.27% and 84.75%, and F1 scores of 84.81% and 91.71%. The code is available at https://github.com/FengheTan9/CMU-Net.
更多查看译文
关键词
medical ultrasound image segmentation
AI 理解论文
溯源树
样例
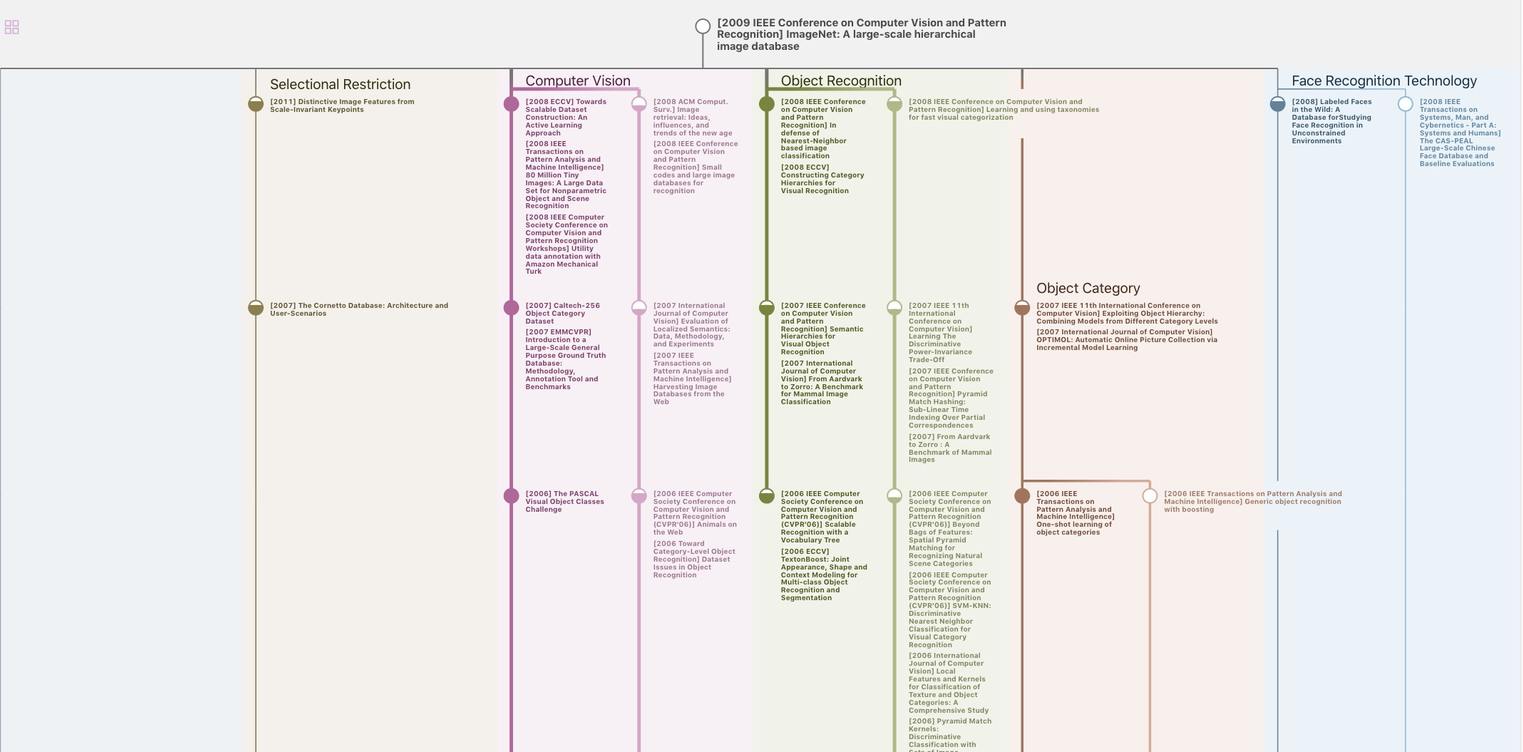
生成溯源树,研究论文发展脉络
Chat Paper
正在生成论文摘要