Grouped Spatial Autoregressive Model
COMPUTATIONAL STATISTICS & DATA ANALYSIS(2023)
关键词
Conditional least-squares estimation,Network autocorrelation heterogeneity,Large-scale network,Naive least-squares estimation,Spatial autoregressive panel model
AI 理解论文
溯源树
样例
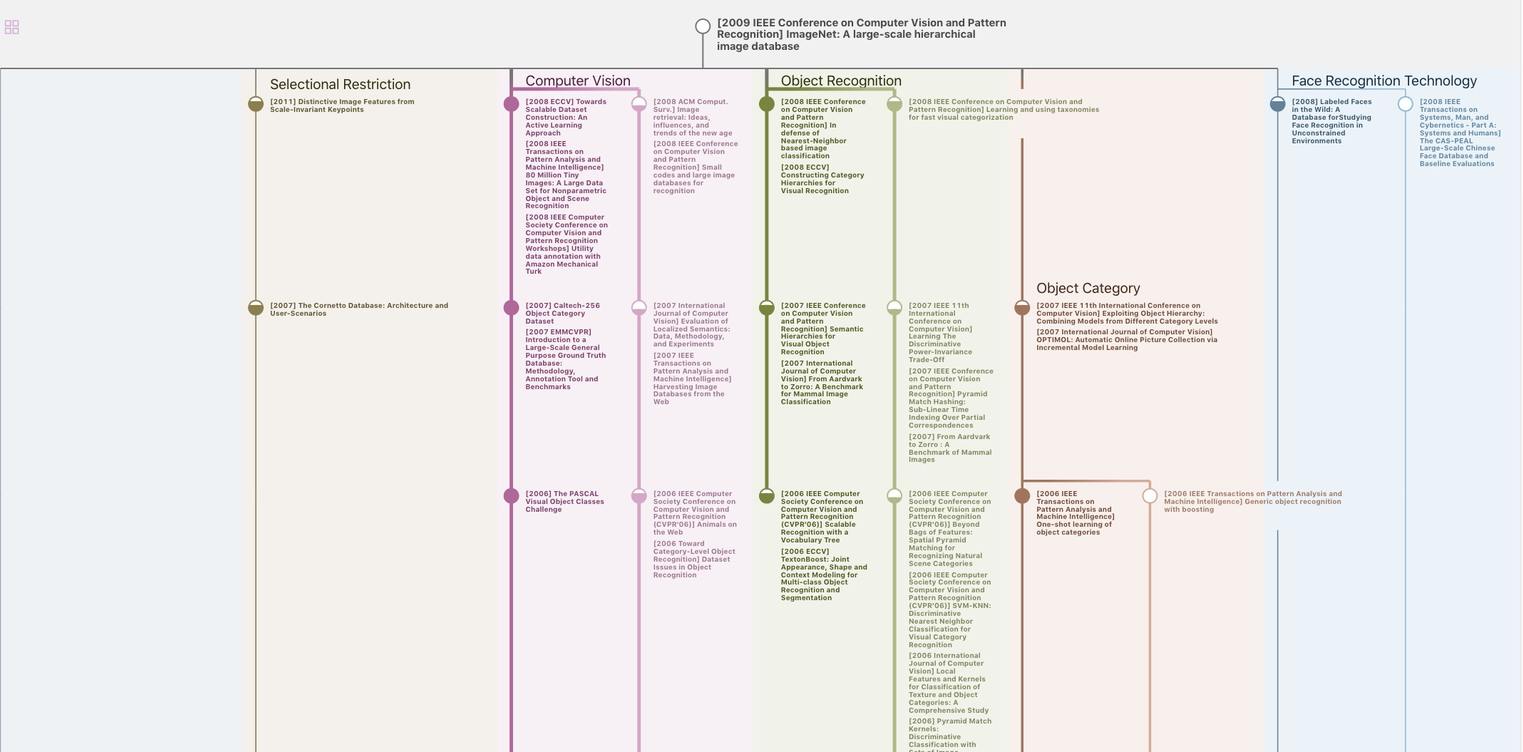
生成溯源树,研究论文发展脉络
Chat Paper
正在生成论文摘要