Multi-Objective Personalized Product Retrieval in Taobao Search
arxiv(2022)
摘要
In large-scale e-commerce platforms like Taobao, it is a big challenge to retrieve products that satisfy users from billions of candidates. This has been a common concern of academia and industry. Recently, plenty of works in this domain have achieved significant improvements by enhancing embedding-based retrieval (EBR) methods, including the Multi-Grained Deep Semantic Product Retrieval (MGDSPR) model [16] in Taobao search engine. However, we find that MGDSPR still has problems of poor relevance and weak personalization compared to other retrieval methods in our online system, such as lexical matching and collaborative filtering. These problems promote us to further strengthen the capabilities of our EBR model in both relevance estimation and personalized retrieval. In this paper, we propose a novel Multi-Objective Personalized Product Retrieval (MOPPR) model with four hierarchical optimization objectives: relevance, exposure, click and purchase. We construct entire-space multi-positive samples to train MOPPR, rather than the single-positive samples for existing EBR models.We adopt a modified softmax loss for optimizing multiple objectives. Results of extensive offline and online experiments show that MOPPR outperforms the baseline MGDSPR on evaluation metrics of relevance estimation and personalized retrieval. MOPPR achieves 0.96% transaction and 1.29% GMV improvements in a 28-day online A/B test. Since the Double-11 shopping festival of 2021, MOPPR has been fully deployed in mobile Taobao search, replacing the previous MGDSPR. Finally, we discuss several advanced topics of our deeper explorations on multi-objective retrieval and ranking to contribute to the community.
更多查看译文
关键词
personalized,search,product,multi-objective
AI 理解论文
溯源树
样例
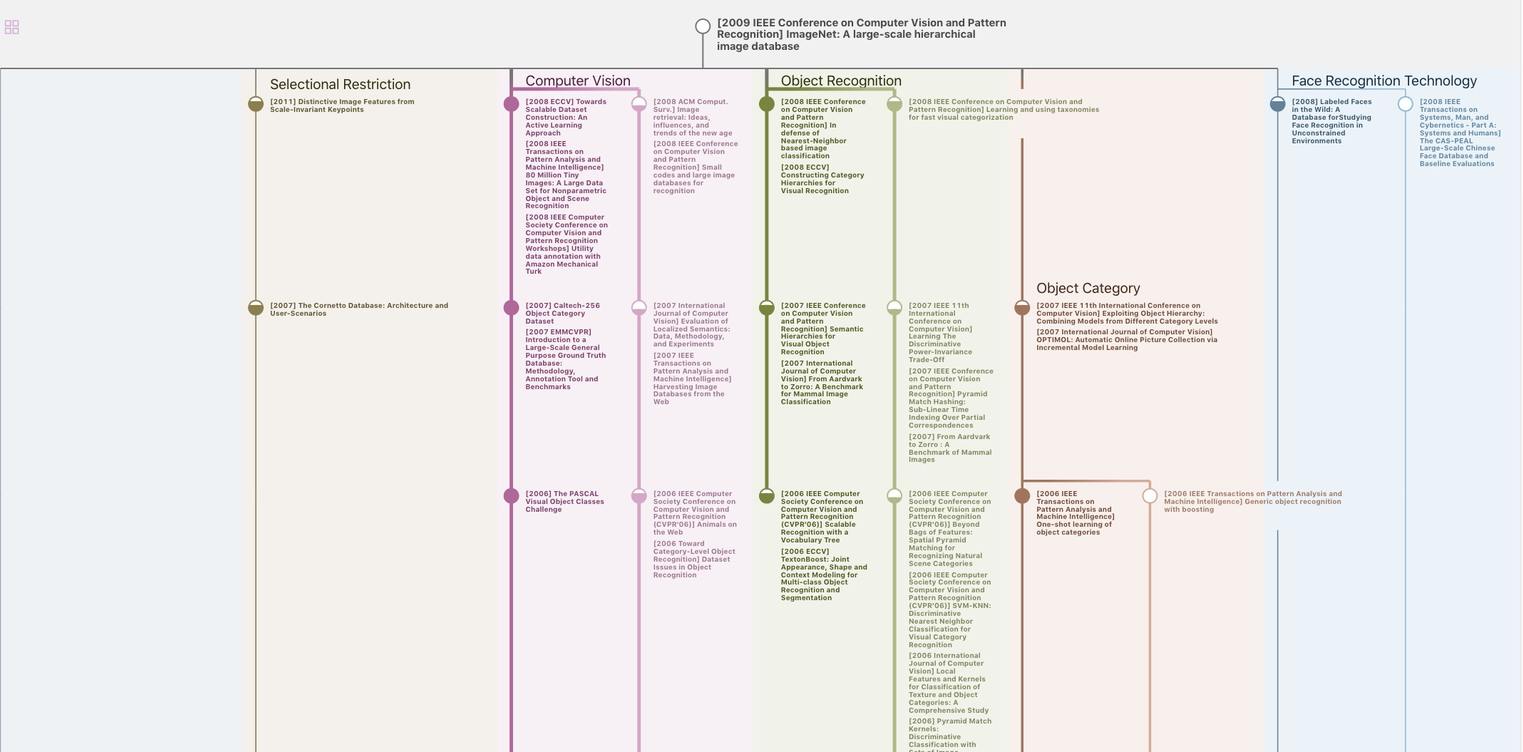
生成溯源树,研究论文发展脉络
Chat Paper
正在生成论文摘要