A deep-CNN based low-cost, multi-modal sensing system for efficient walking activity identification
Multimedia Tools and Applications(2022)
摘要
The misclassification of human activity information by IoT-based smart, health monitoring devices raises concerns about their reliability. For instance, the identification of walking activity by wrist-worn, hand-held, or pocketed devices is often error-prone because tracking of actual leg movement is necessary for proper identification of a walk. In contrast, wearable systems on the waist recognize the bodily movement as a whole and not limb-specific movement. In this paper, we consider the novel problem of distinguishing the walking activity defined as a sequence of repeated leg-swing actions from repetitive leg-swing activities in sitting state. For this purpose, a heterogeneous sensor system is implemented that acquires novel multi-modal data from low-cost leg-worn IMU sensors (m-module) and finger-tip based pulse sensors (p-module). This dataset is then processed to extract features for a supervised learning framework. A 1-D Deep Convolutional Neural Network (DCNN) model is used for system performance evaluation, that prevents misclassification of walking activity with an average accuracy of 97% and maximum accuracy of 99%. With the fusion of features, the DCNN model performs with 2% more accuracy than the performance of the system using only IMU data. For performance comparison with the DCNN model, 4 other supervised learning algorithms have been used, namely Support Vector Machine (SVM), k-Nearest Neighbors (kNN), Decision Tree (DT), and Gaussian Naive Bayes (GNB). The proposed DCNN model outperforms these algorithms by using the m-module features individually and in unison with the p-module features. The performance is also comparable to the state-of-the-art works on intelligent walking activity detection.
更多查看译文
关键词
Multimodal sensors,Human activity recognition,Sensor fusion,Deep CNN,Supervised learning,Walking activity
AI 理解论文
溯源树
样例
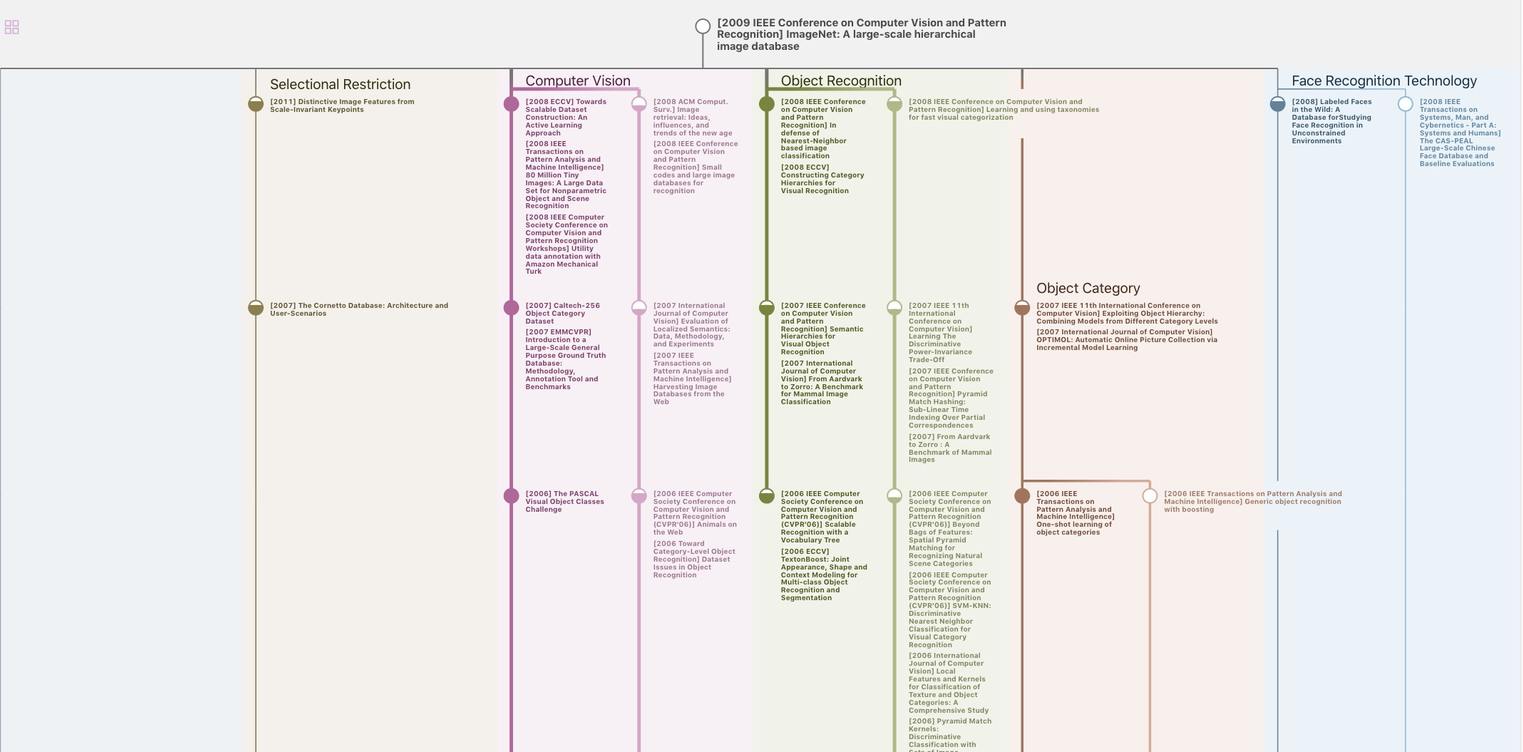
生成溯源树,研究论文发展脉络
Chat Paper
正在生成论文摘要