Machine learning directed multi-objective optimization of mixed variable chemical systems
Chemical Engineering Journal(2023)
摘要
The consideration of discrete variables (e.g. catalyst, ligand, solvent) in experimental self-optimization approaches remains a significant challenge. Herein we report the application of a new mixed variable multi-objective optimization (MVMOO) algorithm for the self-optimization of chemical reactions. Coupling of the MVMOO algorithm with an automated continuous flow platform enabled identification of the trade-off curves for different performance criteria by optimizing the continuous and discrete variables concurrently. This approach utilizes a Bayesian methodology to provide high optimization efficiency, enhances process understanding by considering key interactions between the mixed variables, and requires no prior knowledge of the reaction. Nucleophilic aromatic substitution (SNAr) and palladium catalyzed Sonogashira reactions were investigated, where the effect of solvent and ligand selection on the regioselectivity and process efficiency were determined respectively whilst simultaneously determining the optimum continuous parameters in each case.
更多查看译文
关键词
Automated flow reactor,Mixed variable optimization,Multi-objective,Machine learning,Reaction engineering
AI 理解论文
溯源树
样例
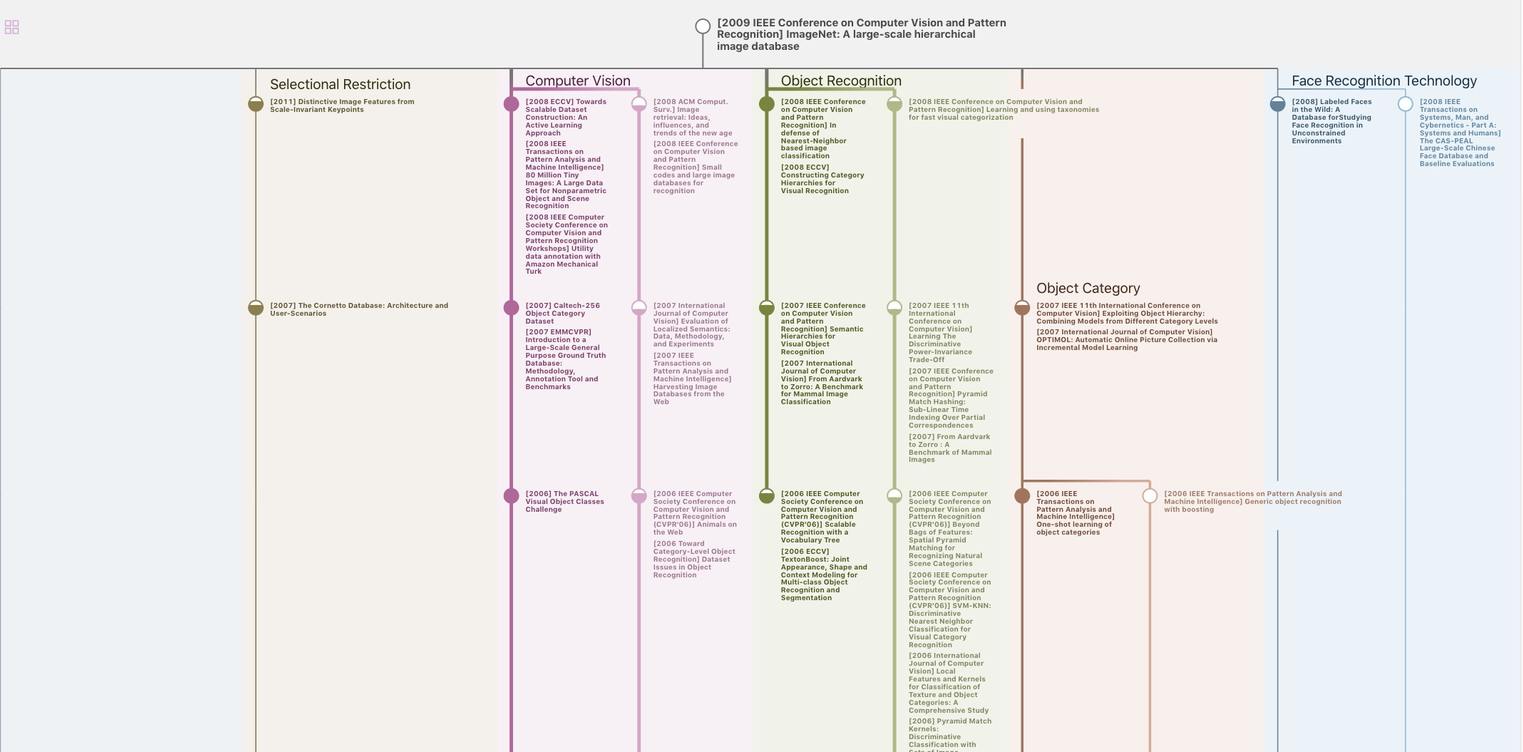
生成溯源树,研究论文发展脉络
Chat Paper
正在生成论文摘要