Towards Efficient Edge Computing Through Adoption of Reinforcement Learning Strategies: A Review
Data Management, Analytics and Innovation(2022)
摘要
This paper contributes towards the mapping of the variants of Reinforcement Learning (RL) techniques to solve the key challenges of Edge Computing (EC) through broadly addressing task handling and Quality of Service (QoS) parameters. EC has bolstered ever since the advent of Industry 4.0 with computationally reliable heterogeneous mobile secured dynamic edge devices powered by an array of multifarious sensors designed on multi-edge hierarchical architectures found a strong footing on the backbone of ably equipped communication protocols to manifest their growth powered by the advent of 5G technology. However, with millions of such edge devices finding its way in a plethora of EC applications, with each having its own set of domain specific challenges, devising a suitable agent so as to sense the environment and learn from it has driven RL find its way as one of the significant tools to make the EC framework intelligent. Here we lay a good understanding of how RL has achieved noteworthy success to solve some of the pressing EC challenges, given that EC finds its use in settings of autonomous driving, content delivery, smart grid, healthcare applications and so on.
更多查看译文
关键词
Edge computing, Reinforcement learning, Task handling, Quality of service
AI 理解论文
溯源树
样例
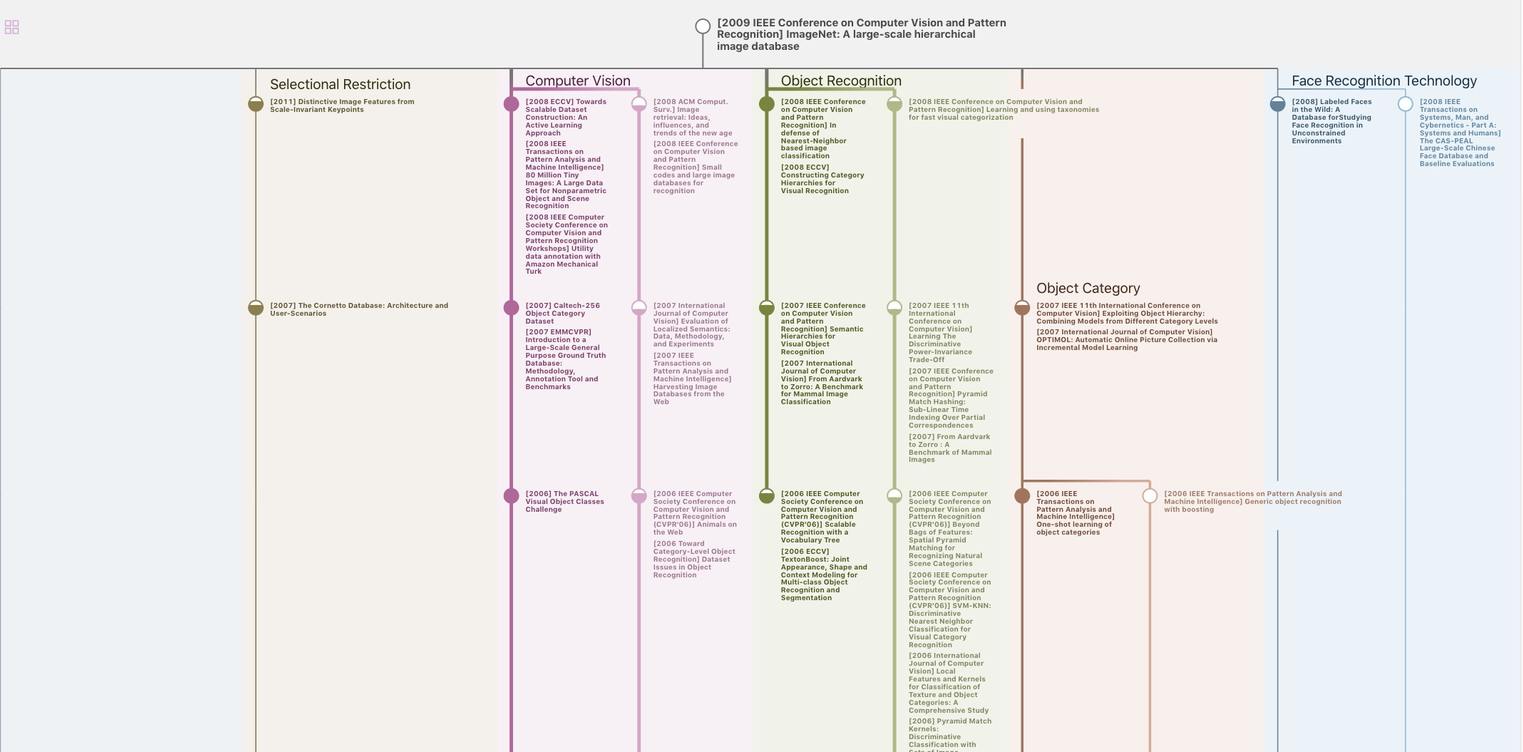
生成溯源树,研究论文发展脉络
Chat Paper
正在生成论文摘要