Analysis on Norms of Word Embedding and Hidden Vectors in Neural Conversational Model Based on Encoder-Decoder RNN
IEICE Transactions on Information and Systems(2022)
摘要
A neural conversational model (NCM) based on an encoder-decoder recurrent neural network (RNN) with an attention mech-anism learns different sequence-to-sequence mappings from what neural machine translation (NMT) learns even when based on the same technique. In the NCM, we confirmed that target-word-to-source-word mappings cap-tured by the attention mechanism are not as clear and stationary as those for NMT. Considering that vector norms indicate a magnitude of information in the processing, we analyzed the inner workings of an encoder-decoder GRU-based NCM focusing on the norms of word embedding vectors and hidden vectors. First, we conducted correlation analyses on the norms of word embedding vectors with frequencies in the training set and with con-ditional entropies of a bi-gram language model to understand what is cor-related with the norms in the encoder and decoder. Second, we conducted correlation analyses on norms of change in the hidden vector of the recur-rent layer with their input vectors for the encoder and decoder, respectively. These analyses were done to understand how the magnitude of information propagates through the network. The analytical results suggested that the norms of the word embedding vectors are associated with their semantic in-formation in the encoder, while those are associated with the predictability as a language model in the decoder. The analytical results further revealed how the norms propagate through the recurrent layer in the encoder and decoder.
更多查看译文
关键词
neural conversational model,encoder-decoder recurrent neural network,vector norm,word embedding
AI 理解论文
溯源树
样例
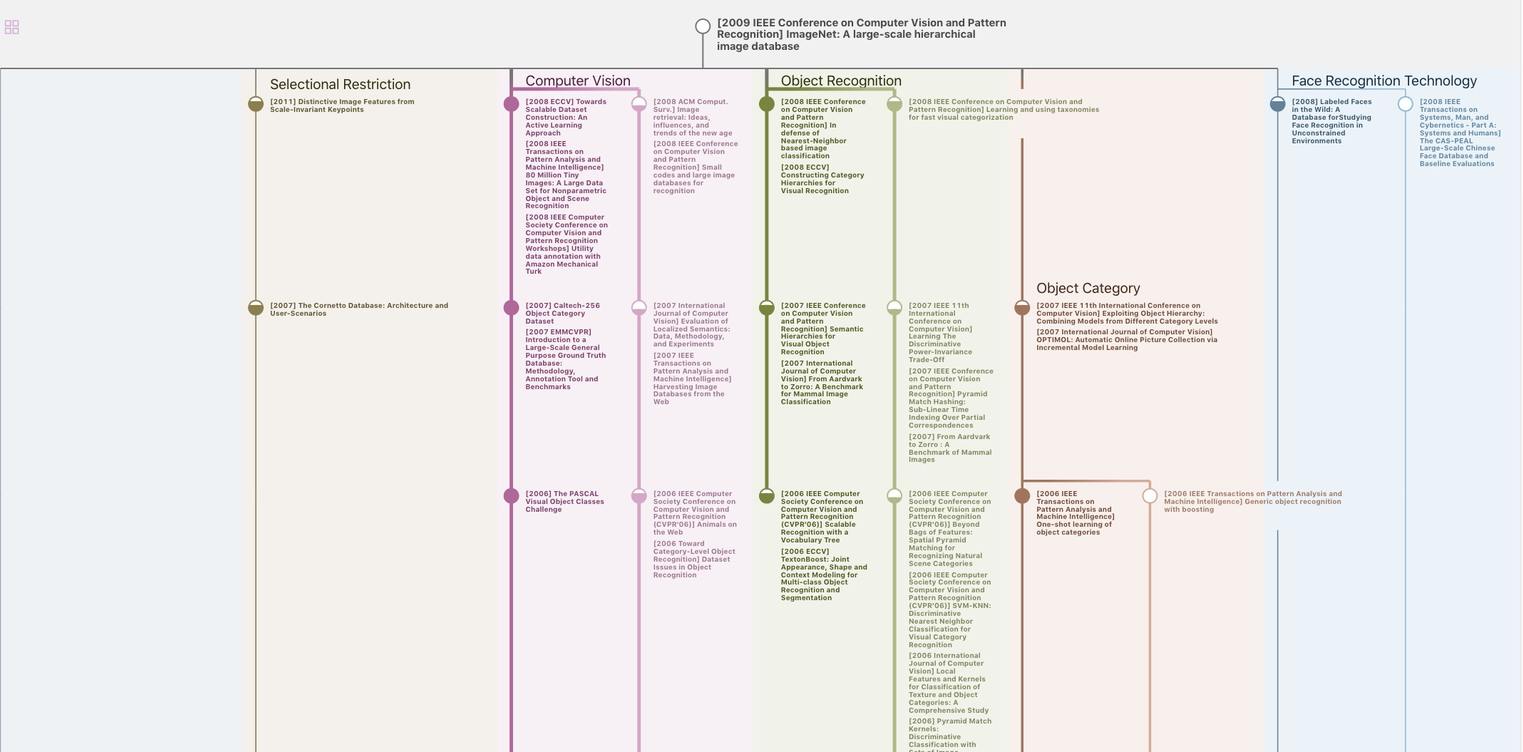
生成溯源树,研究论文发展脉络
Chat Paper
正在生成论文摘要