Rediscovering Reliable Components Analysis: An Application to Executive Function Skills in Early Childhood
Psychological assessment(2023)
摘要
Executive function (EF) assessments often involve the administration of multiple tasks. Although factor analytic methods are routinely used to summarize performance across multiple tasks, they may not be optimal for this purpose. We introduce reliable component analysis (RCA) as a strategy for summarizing EF task performance and demonstrate how it compares to traditional methods. Participants included 259 children (M = 4.5, SD = 0.6 years old; 55% female; 41% White, 35% Black, 14% Hispanic, 1% Asian, 1% American Indian, and 8% of more than one race) from the Kids Activity and Learning Study. Data collection occurred in center-based preschools and involved direct child assessments of EF, motor, and math skills. Principal components analysis (PCA), principal axis factor analysis (FA), and RCA methods were used to summarize children's performance across a battery of six EF tasks. Whereas PCA and FA indicated that a single composite or factor provided the best representation of EF task data, RCA indicated that three composites were justifiable. RCA composites were moderately to strongly correlated with PCA and FA scores (rs = .39-.79). Regression models indicated that all three approaches for combining EF task scores explained the same proportion of variance in motor and math skills outcomes, though the contributions of individual composite and factor scores varied. Results are discussed with respect to how RCA differs from more commonly used tools for data reduction. Public Significance Statement Executive function (EF) assessments involve the administration of multiple tasks to children or adults. It is not yet clear how best to summarize performance across tasks. We present a new statistical method for doing so.
更多查看译文
关键词
principal components analysis,factor analysis,reliable components analysis,executive function
AI 理解论文
溯源树
样例
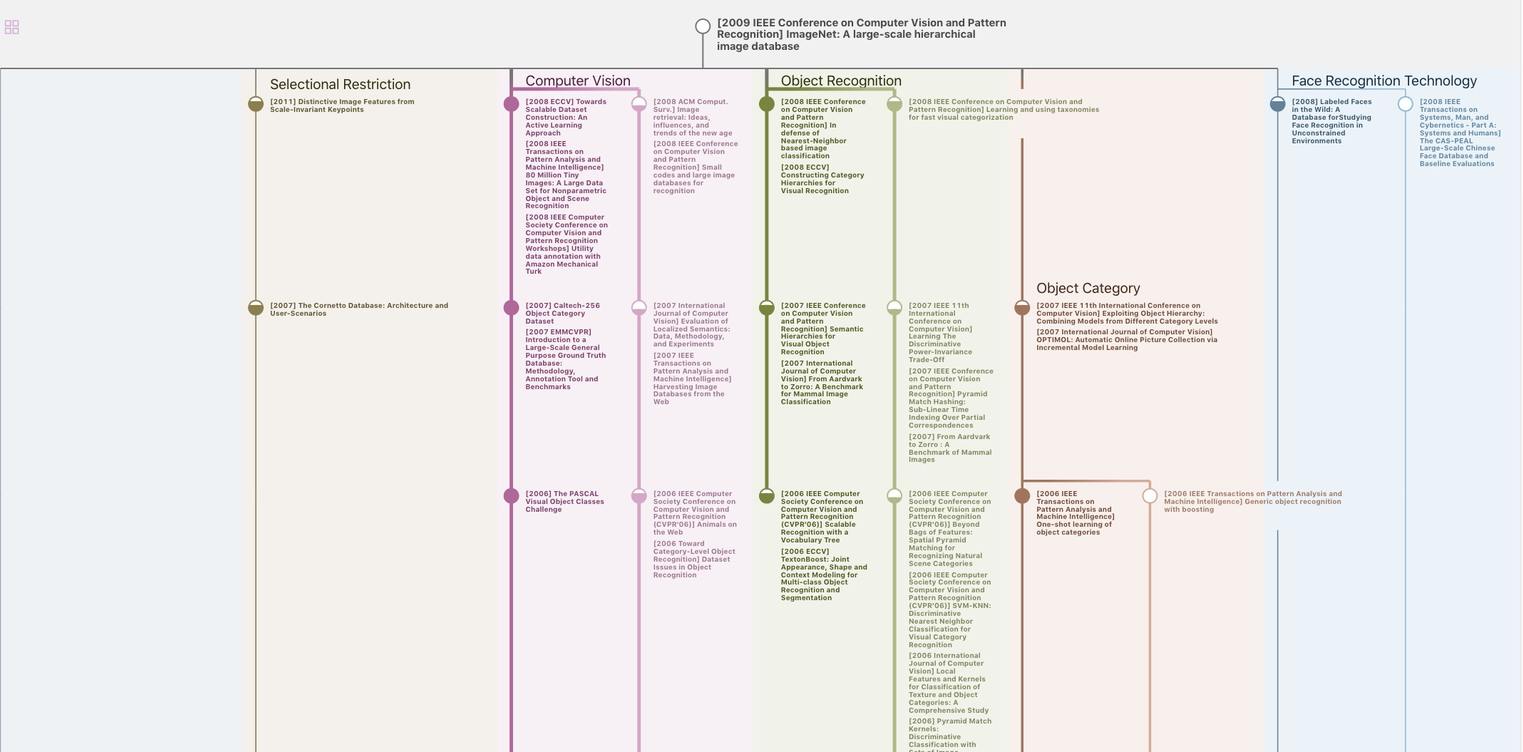
生成溯源树,研究论文发展脉络
Chat Paper
正在生成论文摘要