How Reliable is Distribution of Relaxation Times (DRT) Analysis? A Dual Regression-Classification Perspective on DRT Estimation, Interpretation, and Accuracy
ELECTROCHIMICA ACTA(2023)
Key words
Electrochemical impedance spectroscopy,Distribution of relaxation times,Equivalent circuit,Autonomous,Classification
AI Read Science
Must-Reading Tree
Example
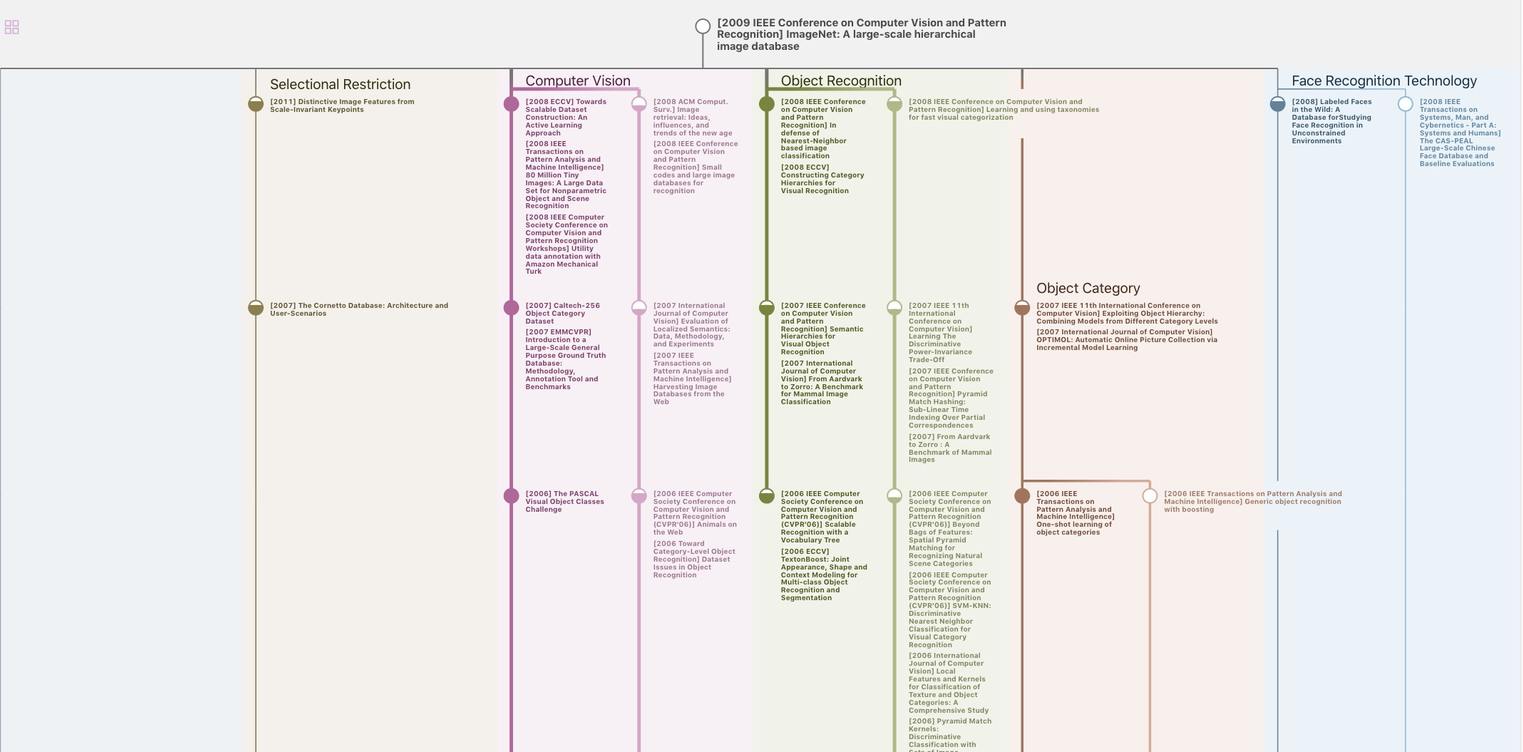
Generate MRT to find the research sequence of this paper
Chat Paper
Summary is being generated by the instructions you defined