Terrain Traversal Cost Learning with Knowledge Transfer Between Multi-legged Walking Robot Gaits
International Conference on Autonomous Robot Systems and Competitions (ICARSC)(2022)
Key words
terrain traversal cost learning,knowledge transfer,multilegged walking robot gaits,terrain traversal abilities,walking patterns,low-set gaits,cautious gaits,highset gaits,suitable gait,prior experience,particular terrain type,novel gait-terrain interactions,unsampled gait-terrain interactions,gaitterrain cost models,gait-to-gait models,different gaits,unknown terrains,cost prediction,appropriate gait,specific terrains
AI Read Science
Must-Reading Tree
Example
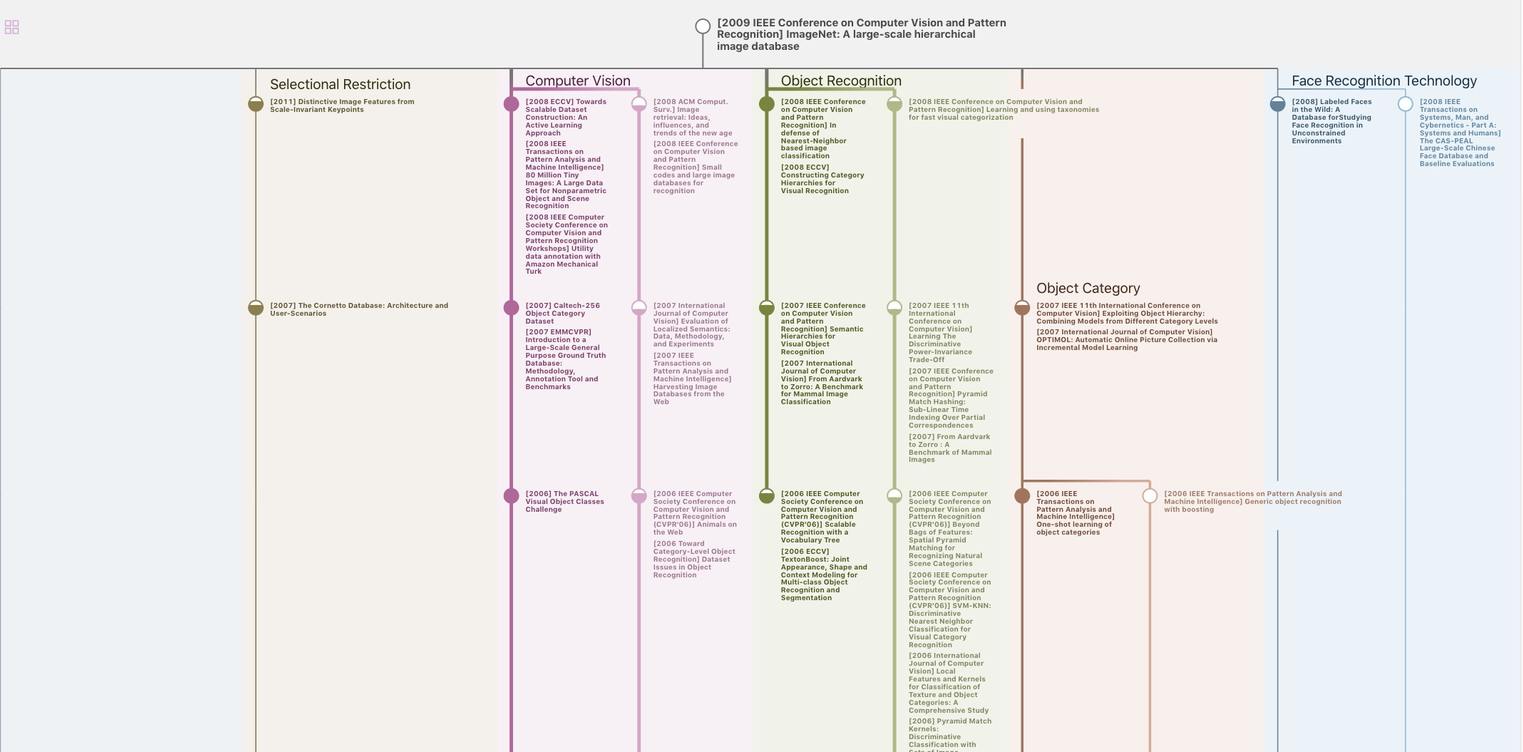
Generate MRT to find the research sequence of this paper
Chat Paper
Summary is being generated by the instructions you defined