Decentralized Natural Policy Gradient with Variance Reduction for Collaborative Multi-Agent Reinforcement Learning
arxiv(2022)
摘要
This paper studies a policy optimization problem arising from collaborative multi-agent reinforcement learning in a decentralized setting where agents communicate with their neighbors over an undirected graph to maximize the sum of their cumulative rewards. A novel decentralized natural policy gradient method, dubbed Momentum-based Decentralized Natural Policy Gradient (MDNPG), is proposed, which incorporates natural gradient, momentum-based variance reduction, and gradient tracking into the decentralized stochastic gradient ascent framework. The $\mathcal{O}(n^{-1}\epsilon^{-3})$ sample complexity for MDNPG to converge to an $\epsilon$-stationary point has been established under standard assumptions, where $n$ is the number of agents. It indicates that MDNPG can achieve the optimal convergence rate for decentralized policy gradient methods and possesses a linear speedup in contrast to centralized optimization methods. Moreover, superior empirical performance of MDNPG over other state-of-the-art algorithms has been demonstrated by extensive numerical experiments.
更多查看译文
关键词
variance reduction,reinforcement,multi-agent
AI 理解论文
溯源树
样例
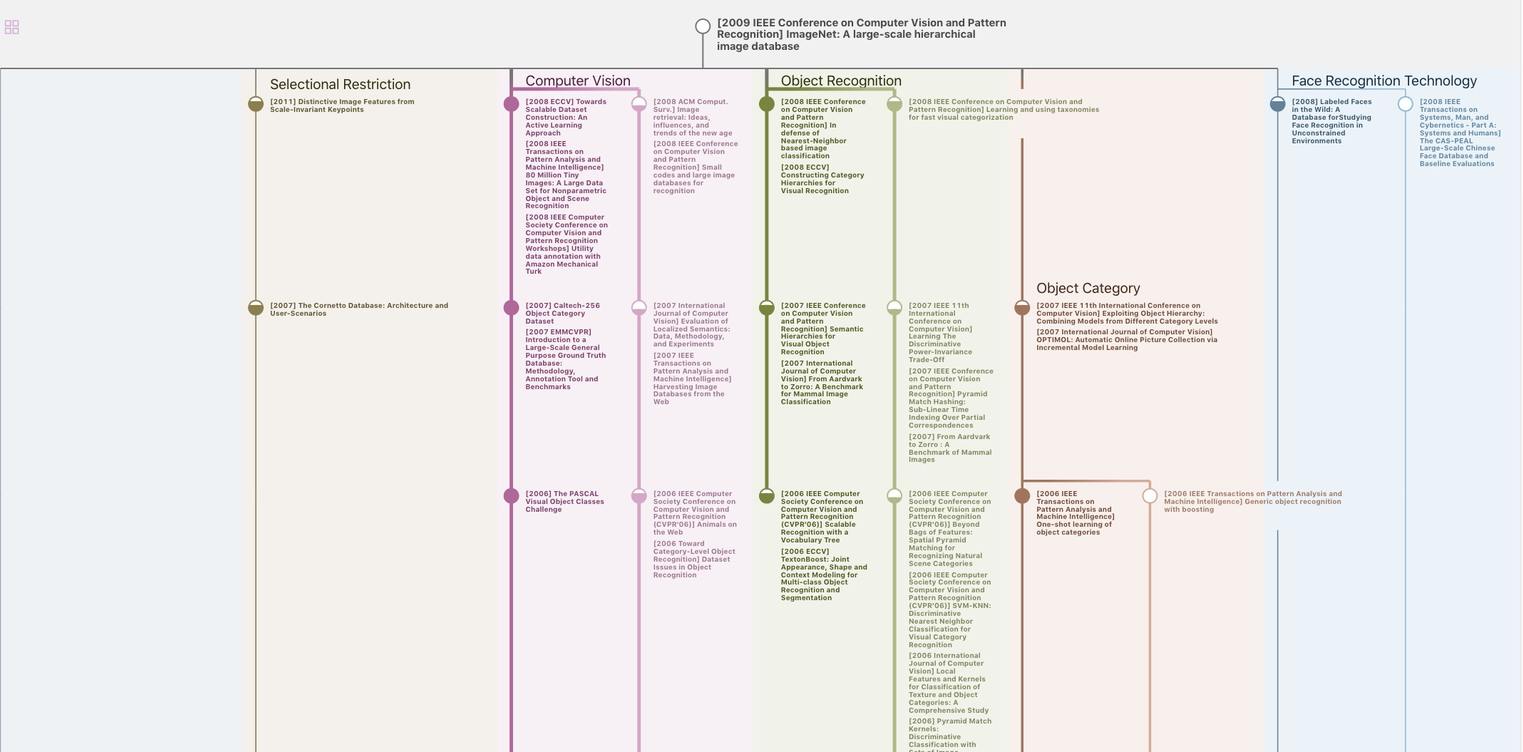
生成溯源树,研究论文发展脉络
Chat Paper
正在生成论文摘要