Towards Accurate Reconstruction of 3D Scene Shape from A Single Monocular Image
IEEE TRANSACTIONS ON PATTERN ANALYSIS AND MACHINE INTELLIGENCE(2023)
Key words
Monocular Depth Estimation,Depth Estimation,3D Shape Measurement,Scene Reconstruction,Camera Calibration
AI Read Science
Must-Reading Tree
Example
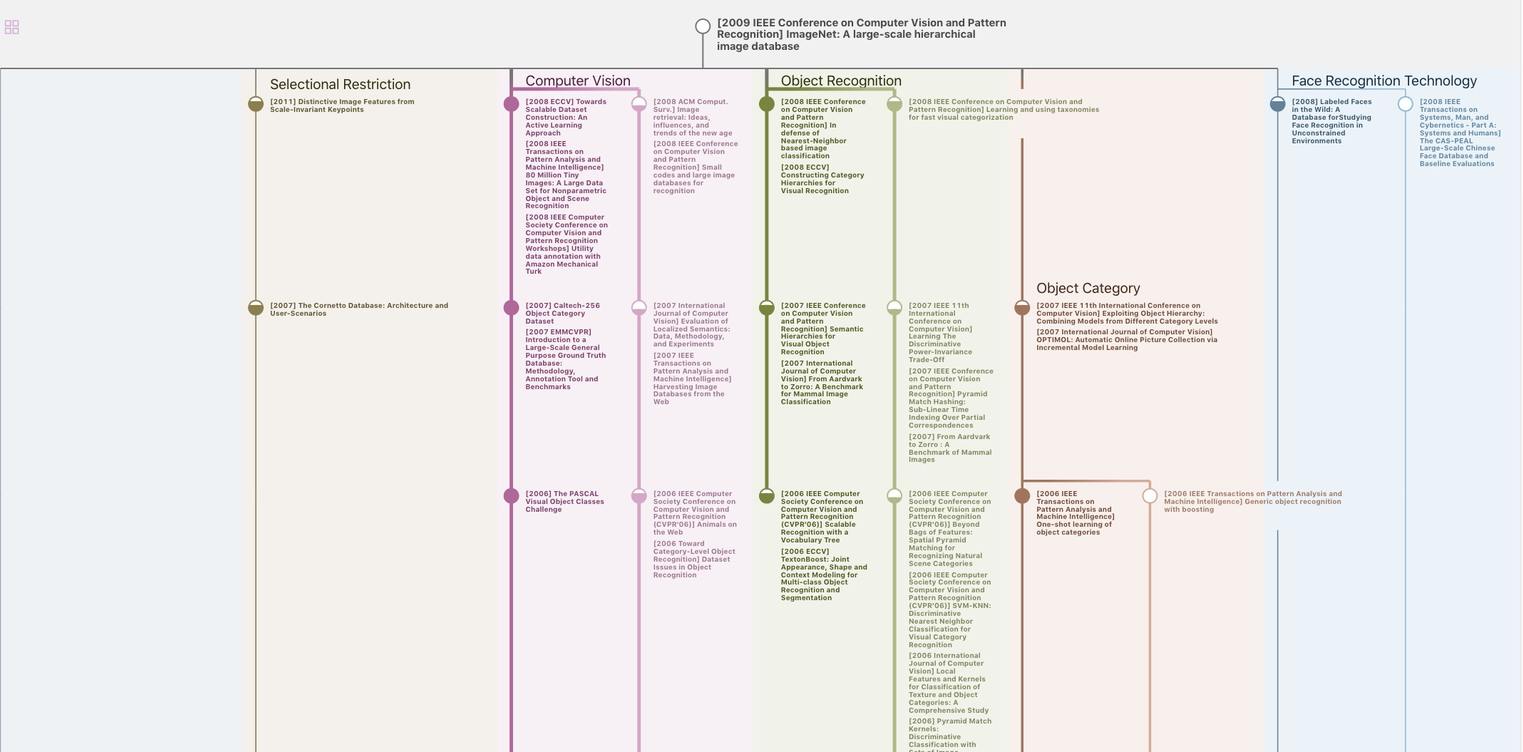
Generate MRT to find the research sequence of this paper
Chat Paper
Summary is being generated by the instructions you defined