Assessing the Existence of a Function in a Dataset with the g3 Indicator
2022 IEEE 38th International Conference on Data Engineering (ICDE)(2022)
摘要
Taking domain knowledge into account is a long-standing issue in AI, especially nowadays where huge amounts of data are collected in the hope of delivering new insights and value. Let us consider the following scenario. Let D(y, x(1), ..., x(n)) be a dataset, Alice a data scientist, Bob a domain expert and y = f(x(1), ..., x(n)) a function known by Bob from his background knowledge. We are interested in the following simple yet crucial questions for Alice: how to define the satisfaction of f in D and how difficult is it to measure that satisfaction? It turns out that those problems are related to functional dependencies (FDs) and especially FD measurements used to quantify their satisfaction in a dataset such as the g(3) indicator. In this paper, we examine the computation of g(3) with crisp FDs (aka. exact FDs) and a large class of non-crisp FDs replacing strict equality by more flexible predicates. Interestingly, it is known that the computation of g(3) with crisp FDs is polynomial but turns out to be NP-Hard for non-crisp FDs. In this paper, we propose different exact and approximate solutions for the computation of g(3) for both types. First, for crisp FDs with very large datasets, we propose solutions based on uniform and stratified random sampling. Second, for non-crisp FDs we present a detailed computation pipeline with various computation optimizations, including approximation algorithms and adaptations of recent developments in sublinear algorithms for NP-Hard problems. We also propose an in-depth experimental study of the algorithms presented in terms of time performances and approximation accuracy. All the algorithms are also made available through FASTG3, an open-source Python library designed to be intuitive and efficient thanks to an underlying C++ implementation.
更多查看译文
关键词
domain knowledge,function,functional dependency,crisp,non-crisp,coverage,g3,error,confidence,NP-hardness,computation
AI 理解论文
溯源树
样例
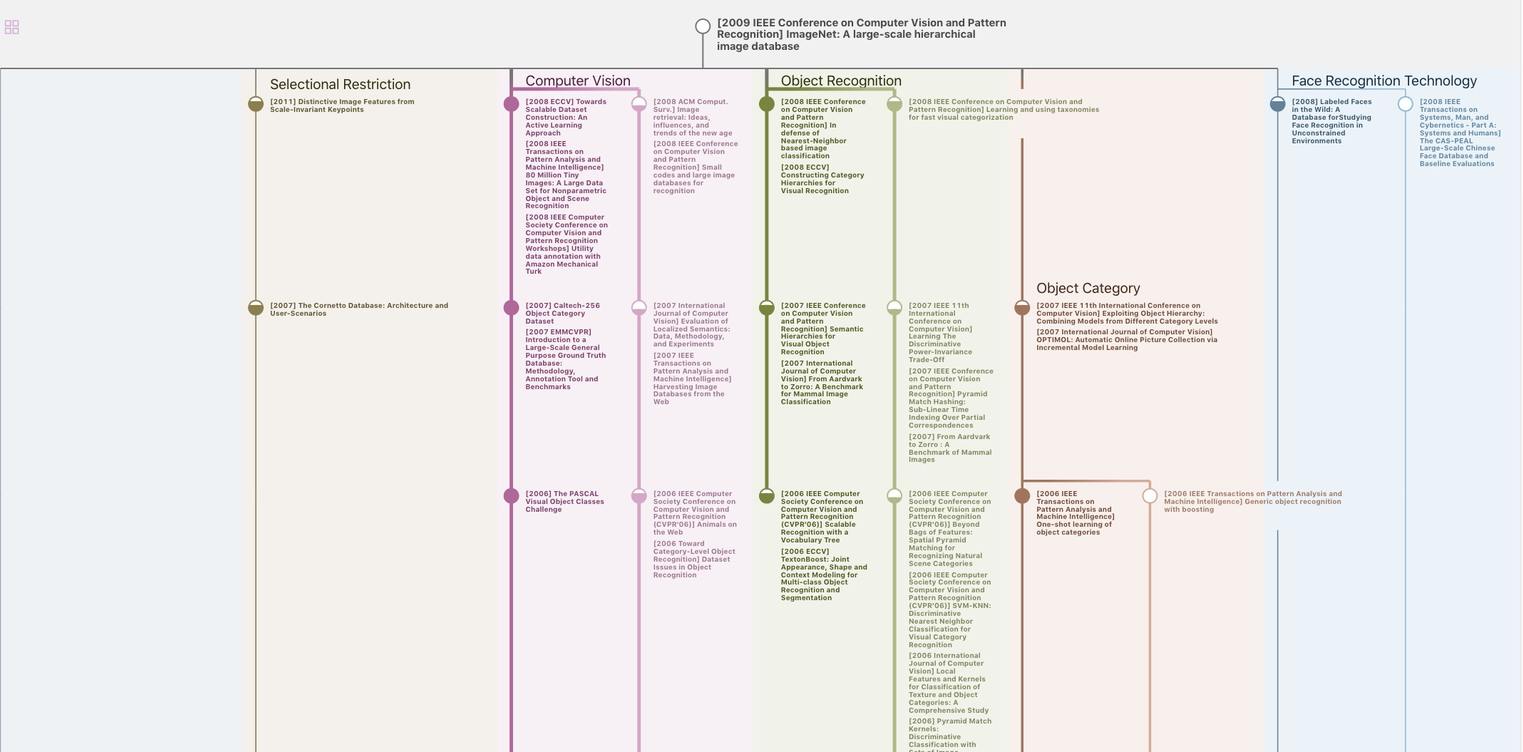
生成溯源树,研究论文发展脉络
Chat Paper
正在生成论文摘要