Self-Weighted Anchor Graph Learning for Multi-View Clustering
IEEE Transactions on Multimedia(2023)
摘要
Graph-based multi-view clustering method has attracted considerable attention in multi-media data analyse community due to its good clustering performance and efficiency in characterizing the relationship between data. But the existing graph-based clustering methods still have many shortcomings. Firstly, they have high computational complexity due to the eigenvalue decomposition. Secondly, the complementary information and spatial structure embedded in different views can affect the clustering performance. However, some existing graph-based clustering methods do not consider these two points. In this article, we use the anchor graphs of different views as input, which effectively reduces the computational complexity. And then we explicitly consider the complementary information and spatial structure between anchor graphs of different views by minimizing the tensor Schatten
$p$
-norm, aiming to achieve a better tensor with low-rank approximation. Finally, we learn the view-consensus anchor graph with connectivity constraints, which can directly indicate clusters by self-weighted strategy. An efficient alternating algorithm is then derived to optimize the proposed multi-view special clustering model. Furthermore, the constructed sequence was proved to converge to the stationary KKT point. Experiments show that our proposed method not only reduces the time cost, but also outperforms the most advanced methods.
更多查看译文
关键词
Anchor graph learning,connectivity constraint,multi-view clustering
AI 理解论文
溯源树
样例
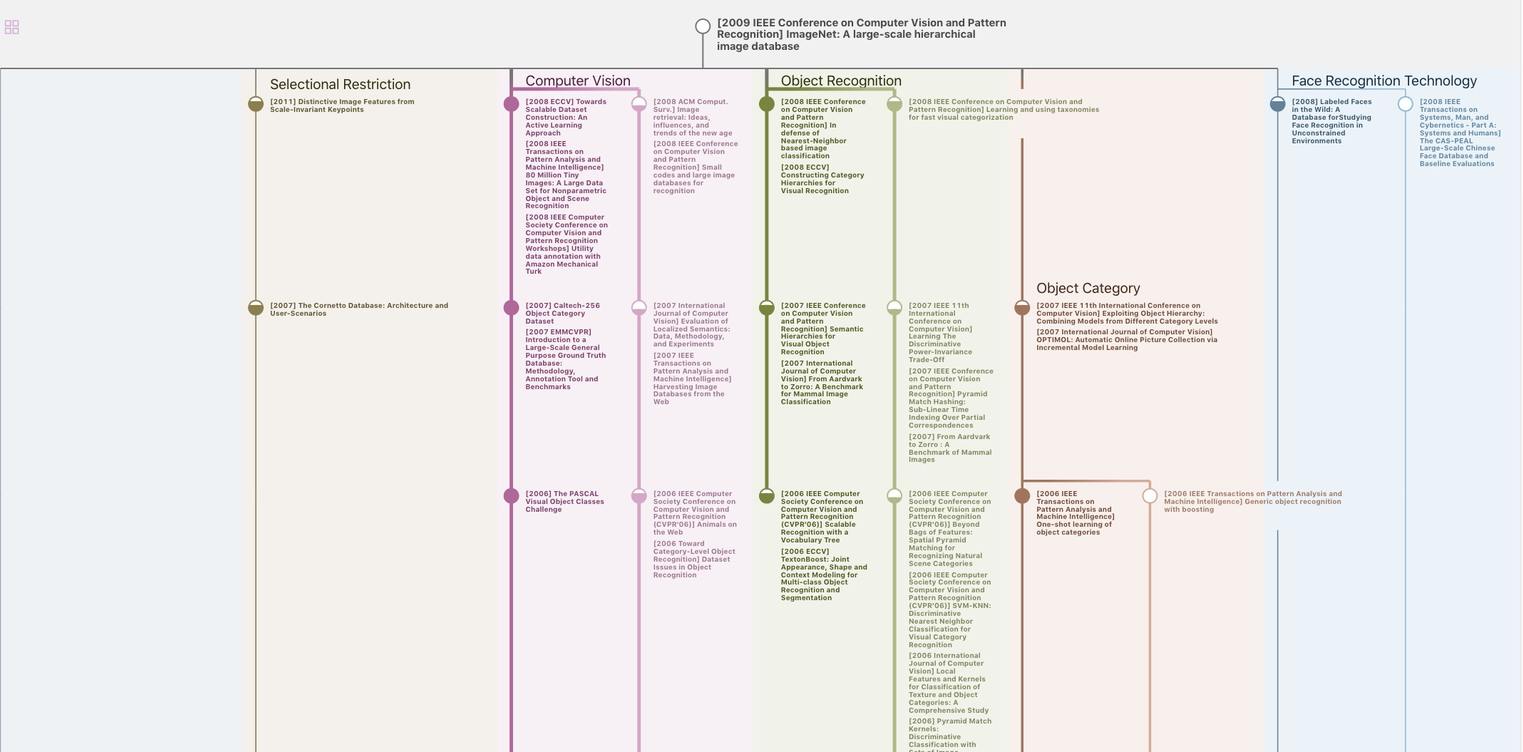
生成溯源树,研究论文发展脉络
Chat Paper
正在生成论文摘要