Multiscale Neural Operator: Learning Fast and Grid-independent PDE Solvers
ICLR 2023(2023)
Key words
physics-informed machine learning,pinns,scientific machine learning,neural ODEs,neural operators,machine learning,neural networks,Matryoshka,multiphysics,multiscale,parametrizations,closure,subgrid,superstructures,partial differential equations,PDEs,differential equations,numerical solvers,physics,hpc,surrogate,reduced order modeling,model reduction,uncertainty quantification,climate,fluid dynamics,physics,computational physics
AI Read Science
Must-Reading Tree
Example
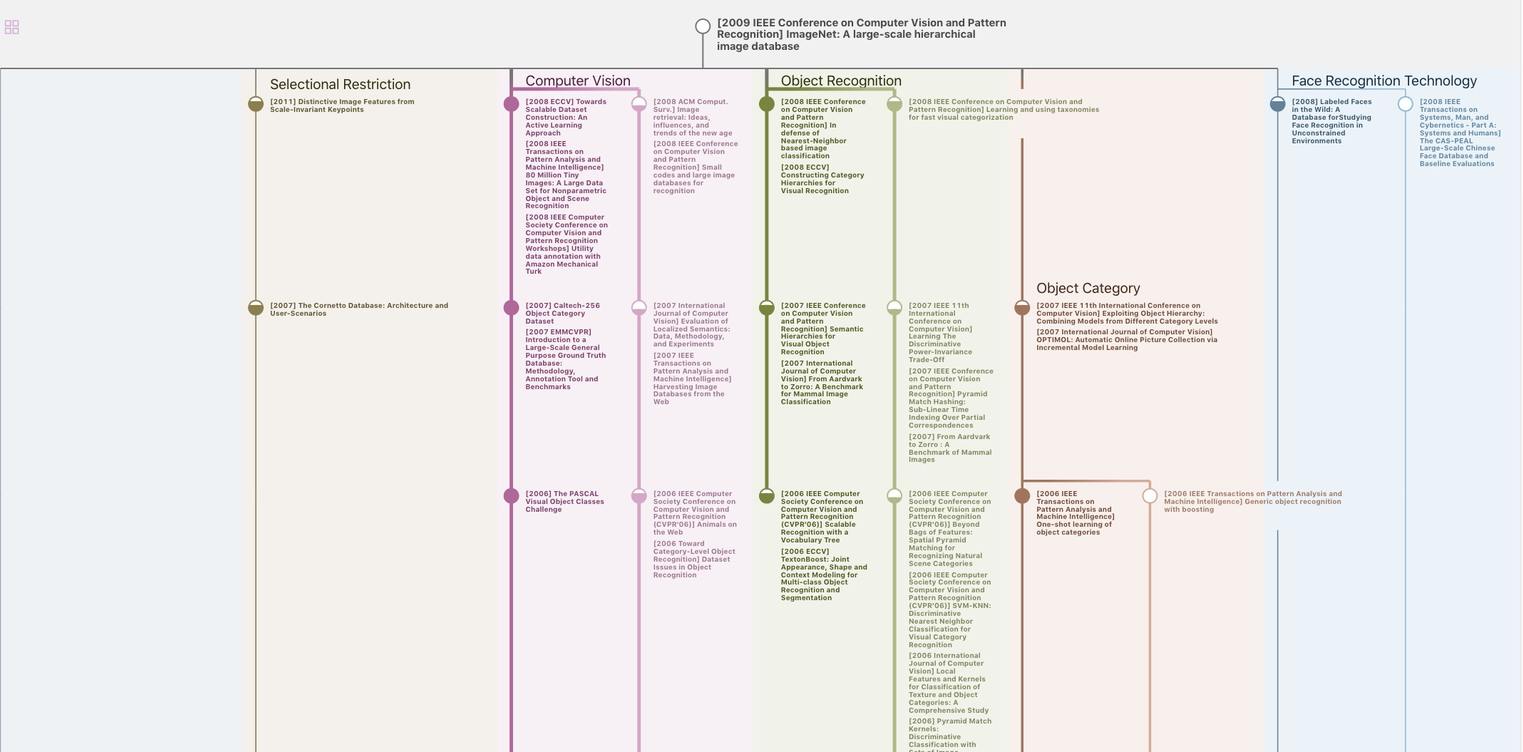
Generate MRT to find the research sequence of this paper
Chat Paper
Summary is being generated by the instructions you defined