Driver Drowsiness Detection in Video Sequences Using Hybrid Selection of Deep Features
Knowledge-Based Systems(2022)
关键词
Drowsiness detection,Transfer learning,Feature selection,SVM classifier
AI 理解论文
溯源树
样例
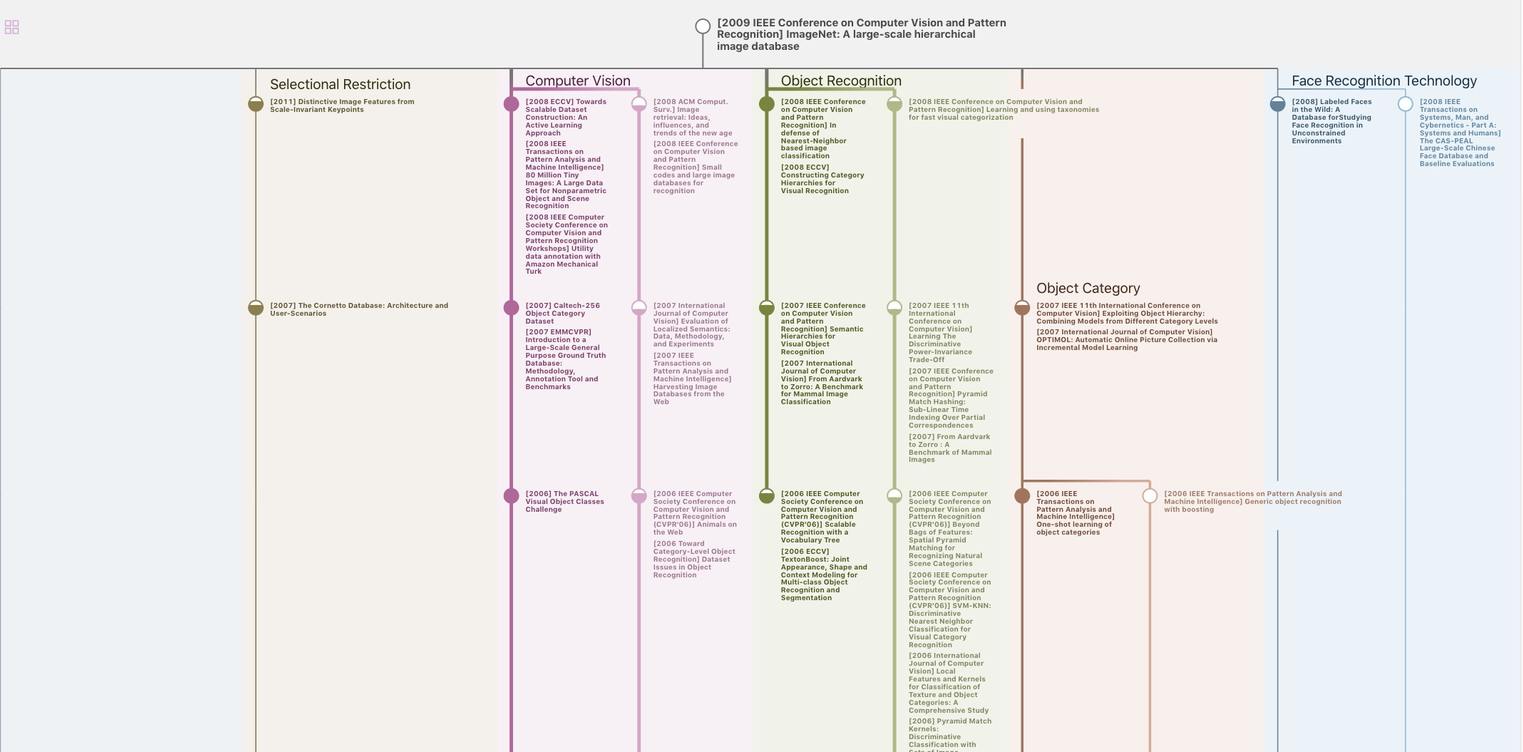
生成溯源树,研究论文发展脉络
Chat Paper
正在生成论文摘要
Knowledge-Based Systems(2022)