ASO Visual Abstract: Solid Attenuation Components Attention Deep Learning Model to Predict Micropapillary and Solid Patterns in Lung Adenocarcinomas on Computed Tomography
Annals of Surgical Oncology(2022)
关键词
Tumor Staging
AI 理解论文
溯源树
样例
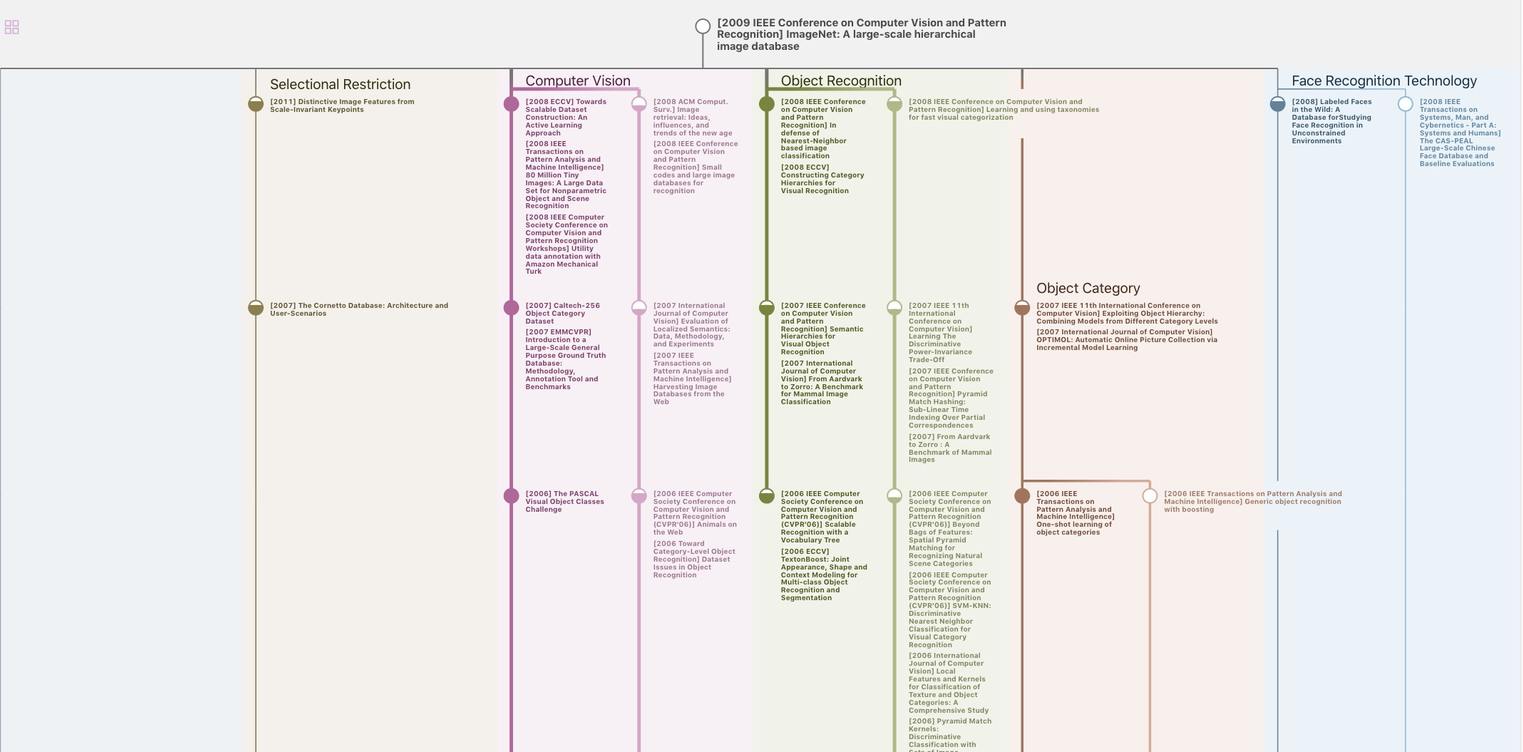
生成溯源树,研究论文发展脉络
Chat Paper
正在生成论文摘要