LED: Latent Variable-based Estimation of Density
CoRR(2022)
摘要
Modern generative models are roughly divided into two main categories: (1) models that can produce high-quality random samples, but cannot estimate the exact density of new data points and (2) those that provide exact density estimation, at the expense of sample quality and compactness of the latent space. In this work we propose LED, a new generative model closely related to GANs, that allows not only efficient sampling but also efficient density estimation. By maximizing log-likelihood on the output of the discriminator, we arrive at an alternative adversarial optimization objective that encourages generated data diversity. This formulation provides insights into the relationships between several popular generative models. Additionally, we construct a flow-based generator that can compute exact probabilities for generated samples, while allowing low-dimensional latent variables as input. Our experimental results, on various datasets, show that our density estimator produces accurate estimates, while retaining good quality in the generated samples.
更多查看译文
关键词
density,estimation,variable-based
AI 理解论文
溯源树
样例
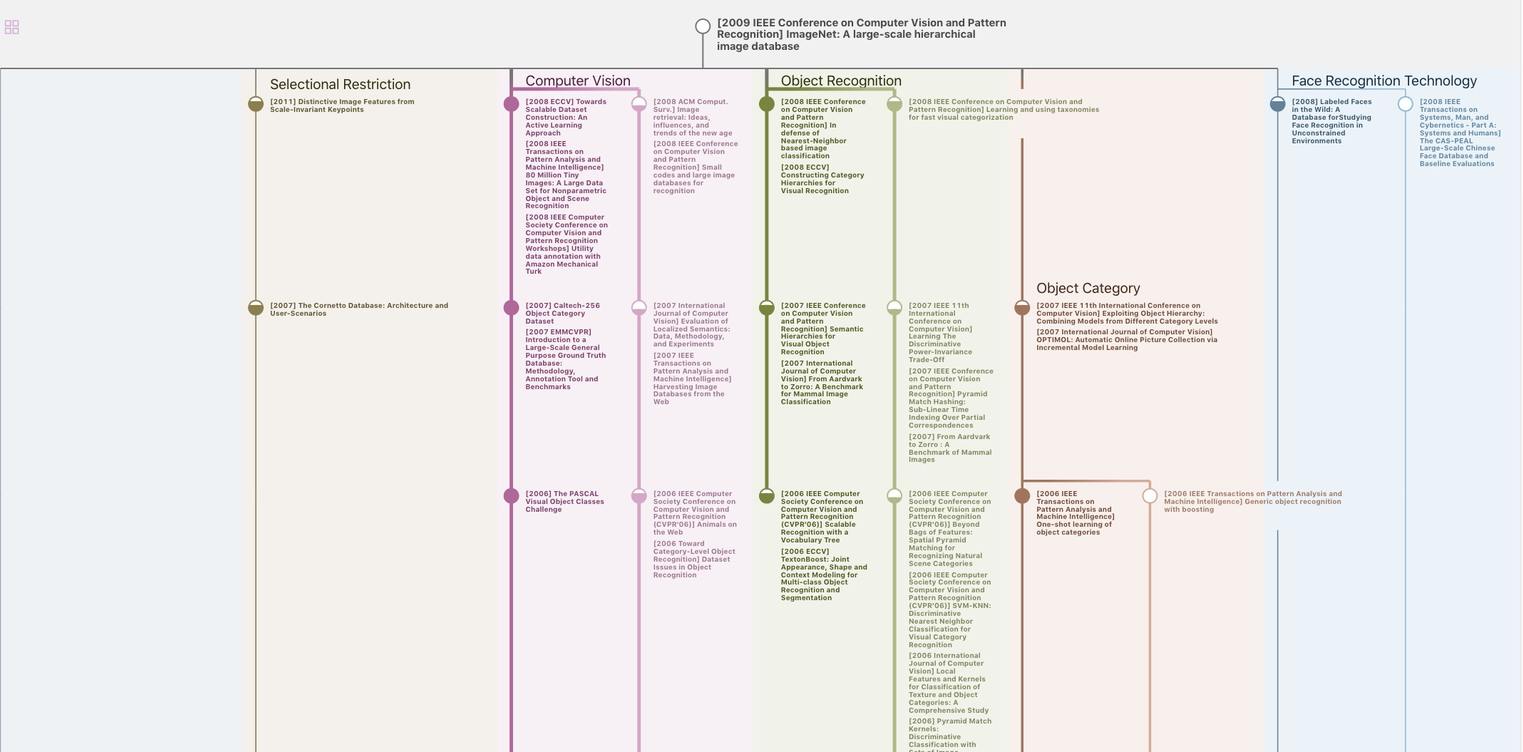
生成溯源树,研究论文发展脉络
Chat Paper
正在生成论文摘要