A Probabilistic Neural Network for Uncertainty Prediction with Applications to Manufacturing Process Monitoring.
Applied Soft Computing(2022)
关键词
Neural networks,Uncertainty quantification,Gaussian mixture model,Manufacturing process monitoring
AI 理解论文
溯源树
样例
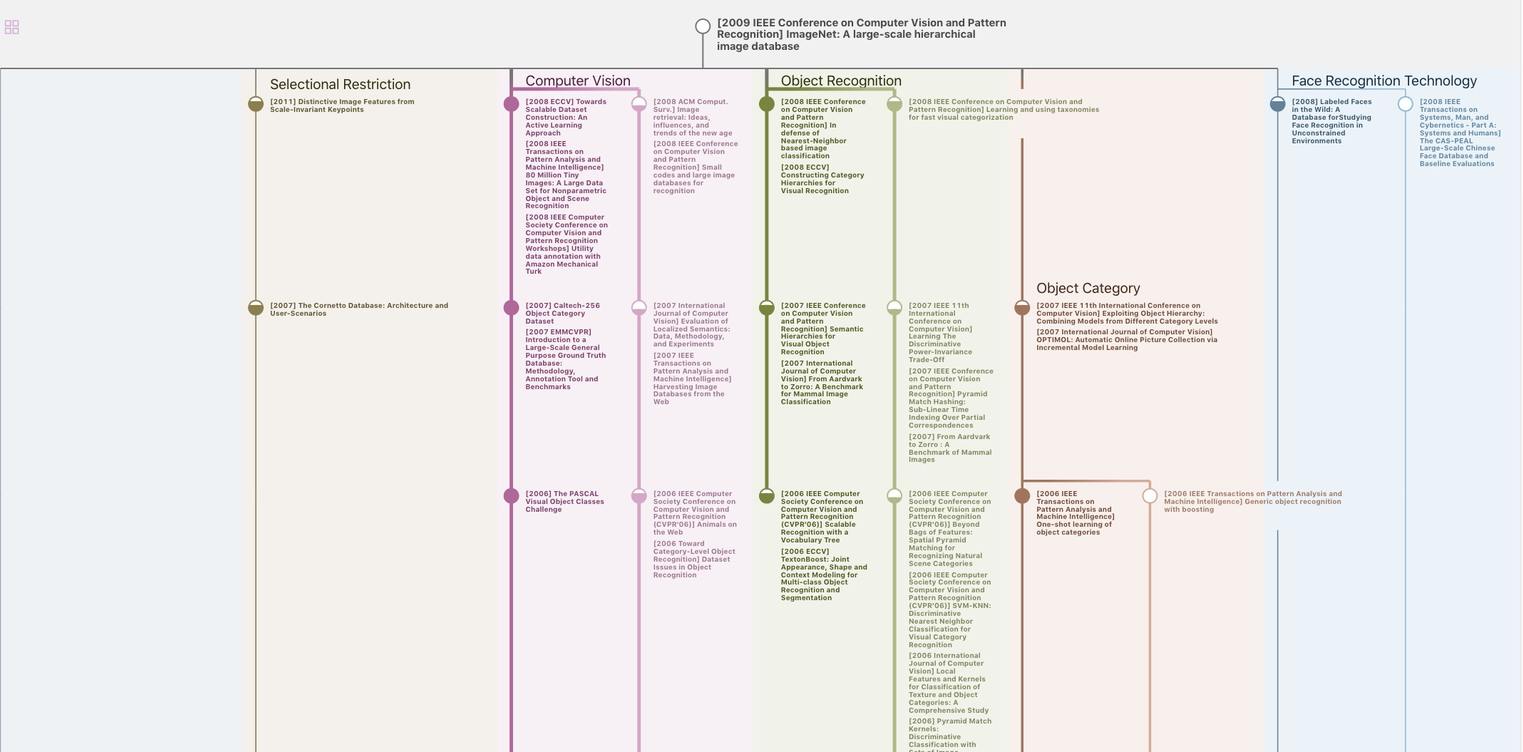
生成溯源树,研究论文发展脉络
Chat Paper
正在生成论文摘要