Lossy Compression with Gaussian Diffusion
ICLR 2023(2022)
摘要
We consider a novel lossy compression approach based on unconditional diffusion generative models, which we call DiffC. Unlike modern compression schemes which rely on transform coding and quantization to restrict the transmitted information, DiffC relies on the efficient communication of pixels corrupted by Gaussian noise. We implement a proof of concept and find that it works surprisingly well despite the lack of an encoder transform, outperforming the state-of-the-art generative compression method HiFiC on ImageNet 64x64. DiffC only uses a single model to encode and denoise corrupted pixels at arbitrary bitrates. The approach further provides support for progressive coding, that is, decoding from partial bit streams. We perform a rate-distortion analysis to gain a deeper understanding of its performance, providing analytical results for multivariate Gaussian data as well as theoretic bounds for general distributions. Furthermore, we prove that a flow-based reconstruction achieves a 3 dB gain over ancestral sampling at high bitrates.
更多查看译文
关键词
diffusion,compression,information theory
AI 理解论文
溯源树
样例
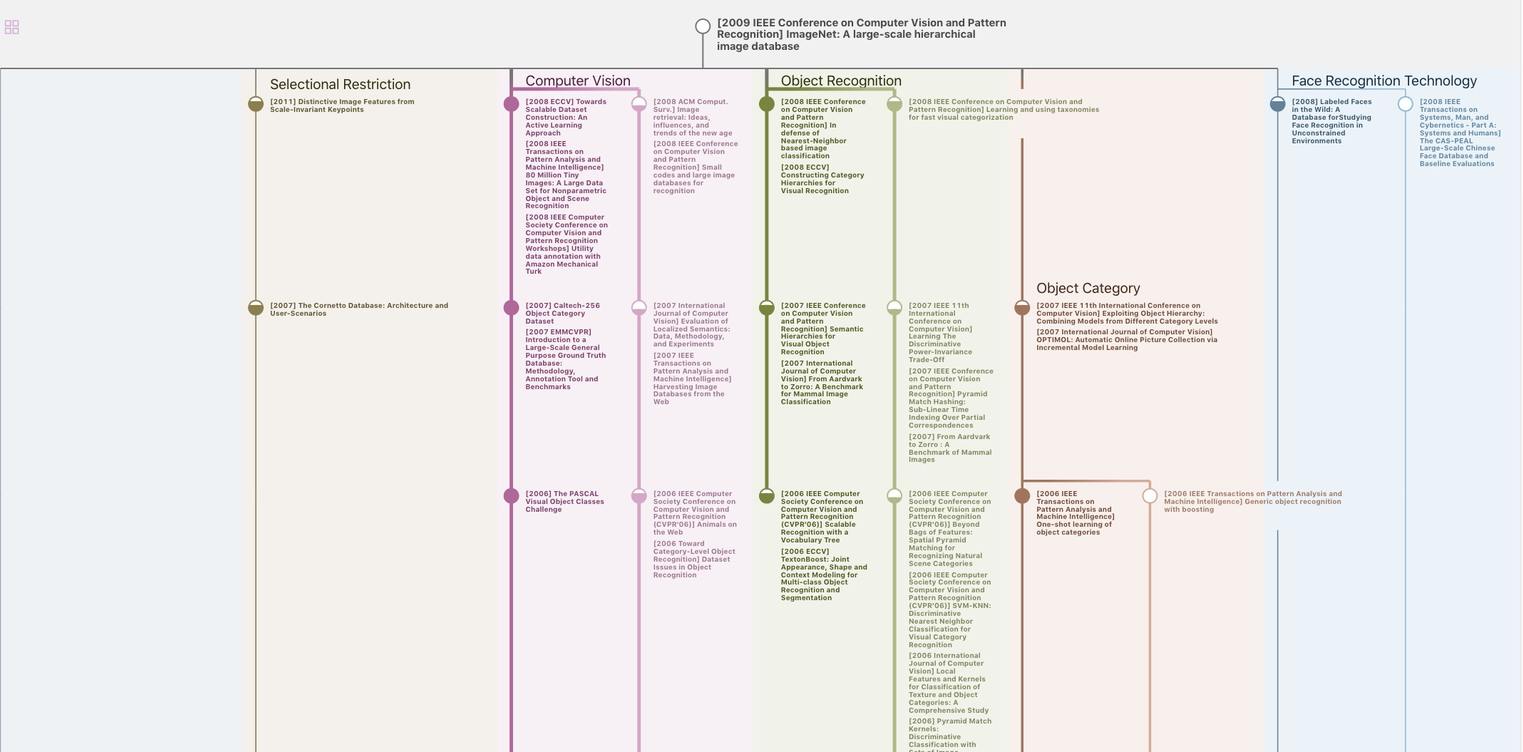
生成溯源树,研究论文发展脉络
Chat Paper
正在生成论文摘要