Detection of Anomalous Behavior of Smartphone Devices using Changepoint Analysis and Machine Learning Techniques
Digital Threats: Research and Practice(2021)
摘要
Detecting anomalous behavior on smartphones is challenging since malware evolution. Other methodologies detect malicious behavior by analyzing static features of the application code or dynamic data samples obtained from hardware or software. Static analysis is prone to code’s obfuscation while dynamic needs that malicious activities to cease to be dormant in the shortest possible time while data samples are collected. Triggering and capturing malicious behavior in data samples in dynamic analysis is challenging since we need to generate an efficient combination of user’s inputs to trigger these malicious activities. We propose a general model which uses a data collector and analyzer to unveil malicious behavior by analyzing the device’s power consumption since this summarizes the changes in software. The data collector uses an automated tool to generate user inputs. The data analyzer uses changepoint analysis to extract features from power consumption and machine learning techniques to train these features. The data analyzer stage contains two methodologies that extract features using parametric and non-parametric changepoint. Our methodologies are efficient in data collection time than a manual method and the data analyzer provides higher accuracy compared to other techniques, reaching over 94% F1-measure for emulated and real malware.
更多查看译文
AI 理解论文
溯源树
样例
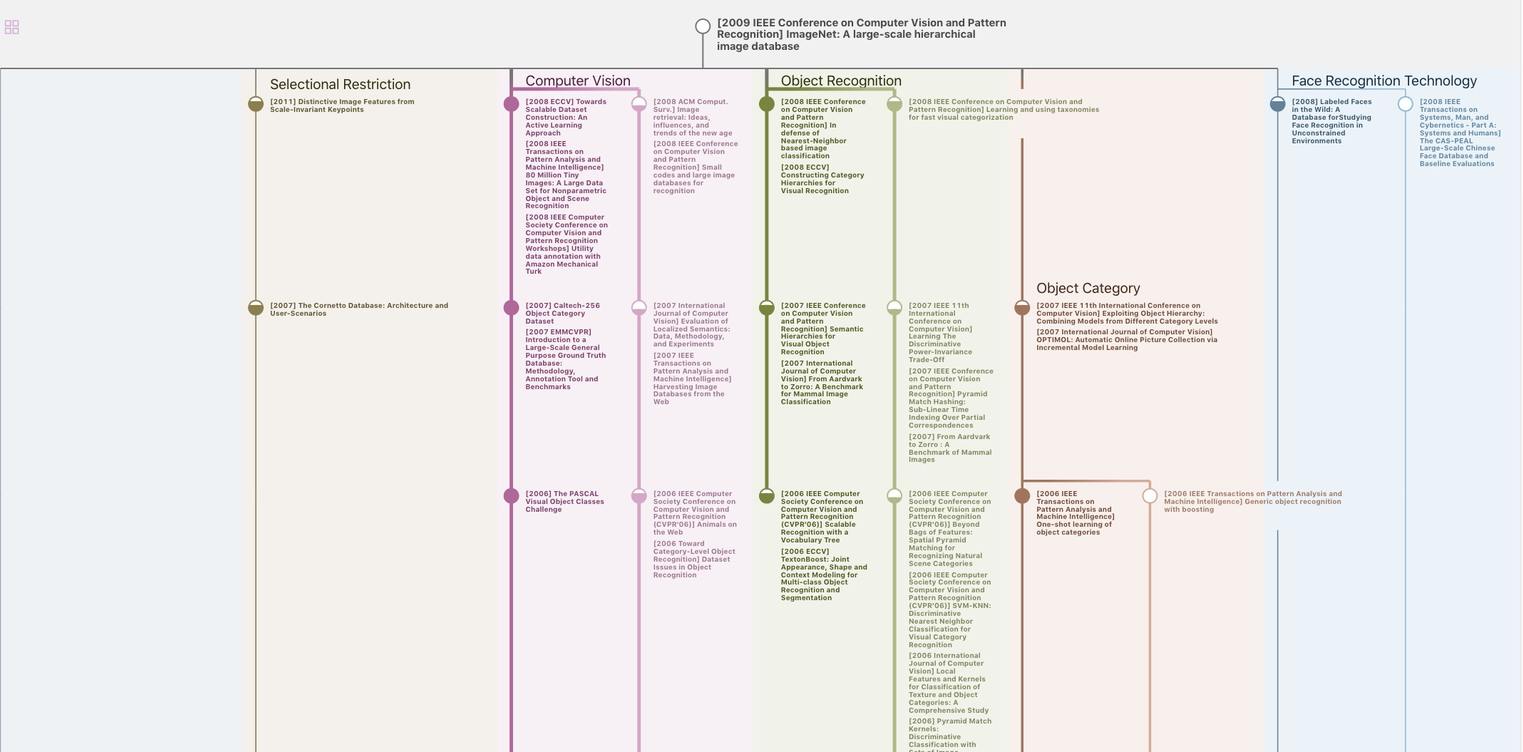
生成溯源树,研究论文发展脉络
Chat Paper
正在生成论文摘要