Complex Locomotion Skill Learning via Differentiable Physics
arxiv(2022)
摘要
Differentiable physics enables efficient gradient-based optimizations of neural network (NN) controllers. However, existing work typically only delivers NN controllers with limited capability and generalizability. We present a practical learning framework that outputs unified NN controllers capable of tasks with significantly improved complexity and diversity. To systematically improve training robustness and efficiency, we investigated a suite of improvements over the baseline approach, including periodic activation functions, and tailored loss functions. In addition, we find our adoption of batching and an Adam optimizer effective in training complex locomotion tasks. We evaluate our framework on differentiable mass-spring and material point method (MPM) simulations, with challenging locomotion tasks and multiple robot designs. Experiments show that our learning framework, based on differentiable physics, delivers better results than reinforcement learning and converges much faster. We demonstrate that users can interactively control soft robot locomotion and switch among multiple goals with specified velocity, height, and direction instructions using a unified NN controller trained in our system.
更多查看译文
关键词
learning,physics,skill,complex
AI 理解论文
溯源树
样例
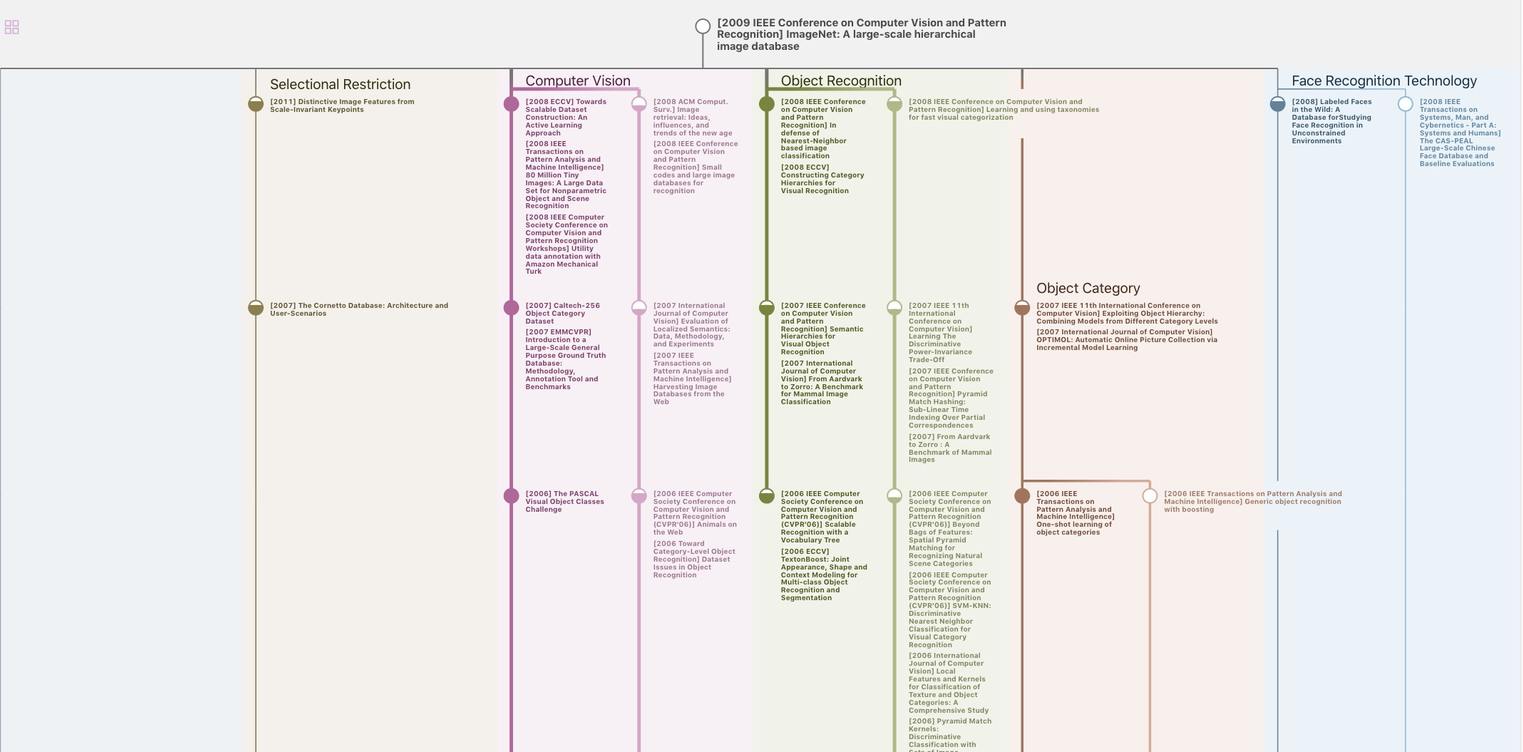
生成溯源树,研究论文发展脉络
Chat Paper
正在生成论文摘要