Multiple instance learning to predict immune checkpoint blockade efficacy using neoantigen candidates
biorxiv(2023)
摘要
Previous studies showed that the neoantigen candidate load is an imperfect predictor of immune checkpoint blockade (ICB) efficacy. Further studies provided evidence that the response to ICB is also affected by the qualitative properties of a few or even single candidates, limiting the predictive power based on candidate quantity alone. Here, we predict ICB efficacy based on neoantigen candidates and their neoantigen features in the context of the mutation type, using Multiple-Instance Learning via Embedded Instance Selection (MILES). Multiple instance learning is a type of supervised machine learning that classifies labeled bags that are formed by a set of unlabeled instances. MILES performed better compared with neoantigen candidate load alone for low-abundant fusion genes in renal cell carcinoma. Our findings suggest that MILES is an appropriate method to predict the efficacy of ICB therapy based on neoantigen candidates without requiring direct T cell response information.
更多查看译文
关键词
immune checkpoint blockade efficacy,multiple instance,learning
AI 理解论文
溯源树
样例
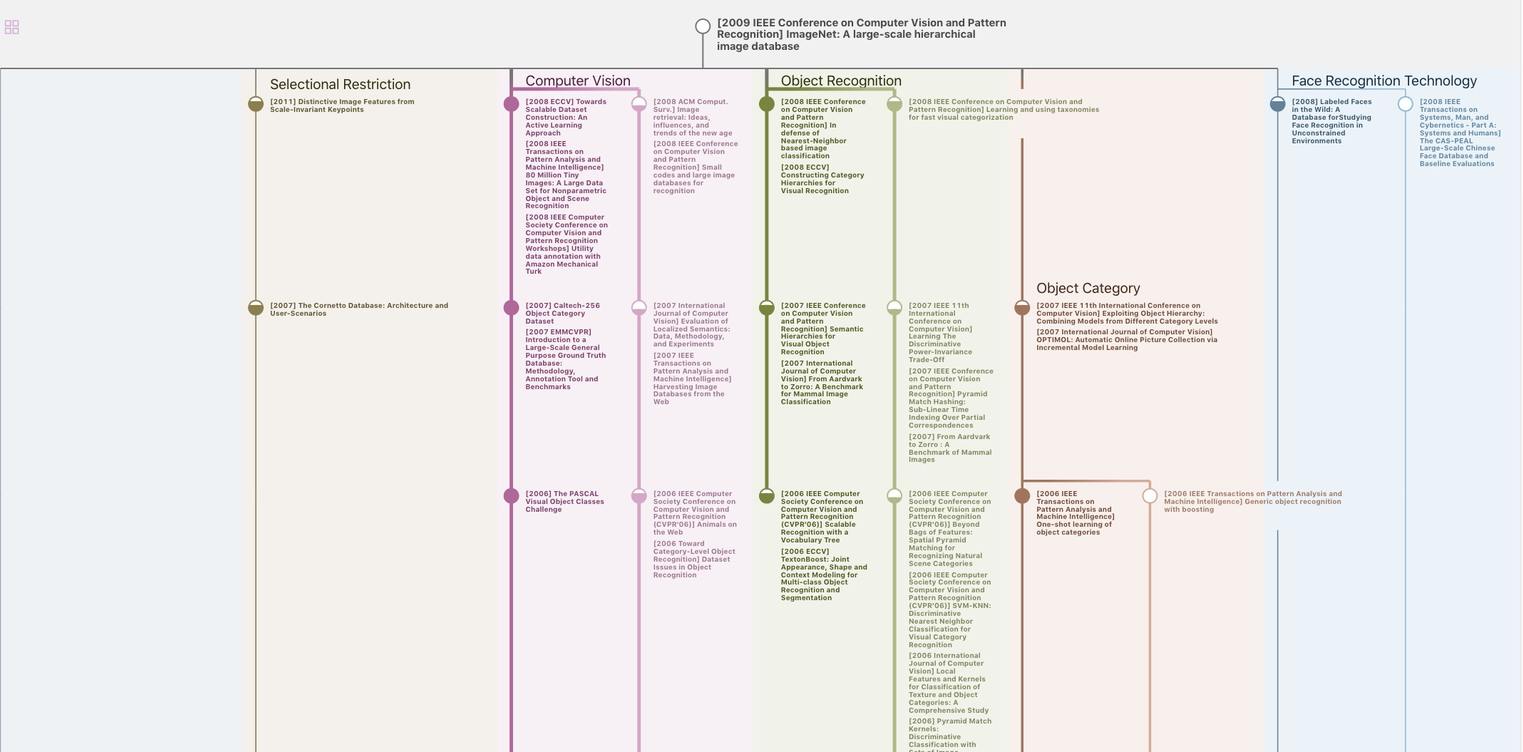
生成溯源树,研究论文发展脉络
Chat Paper
正在生成论文摘要