Adversarially Robust Bayesian Optimization for Efficient Auto-Tuning of Generic Control Structures under Uncertainty
AIChE Journal(2022)
Key words
controller auto-tuning,data-driven optimization,model uncertainty,robust Bayesian optimization
AI Read Science
Must-Reading Tree
Example
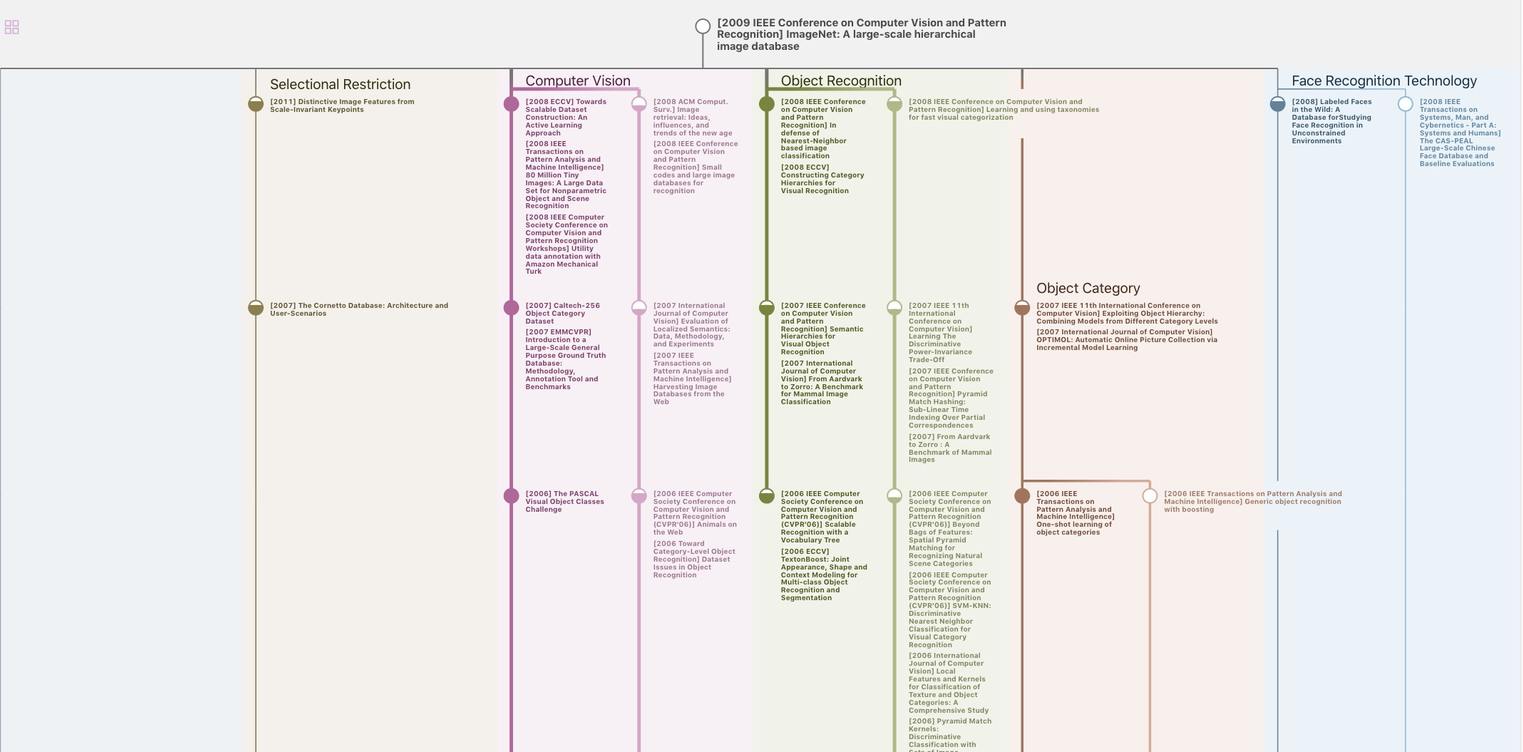
Generate MRT to find the research sequence of this paper
Chat Paper
Summary is being generated by the instructions you defined