Adjustable Model Compression Using Multiple Genetic Algorithm
IEEE Transactions on Multimedia(2023)
摘要
Generative Adversarial Networks (GAN) is a popular machine learning method that possesses powerful image generation ability, which is useful for different multimedia applications (e.g., photographic filters, image editing). However, typical GAN models have a large memory footprint that limits their practical applications for resource-constrained devices (e.g., smartphones). To deploy GAN models on devices with various hardware constraints, we propose our method, AdjustableGAN, which can compress a pretrained GAN model to different compression ratios. Our method compresses GAN by performing filter-wise pruning that follows these objectives: (1) deactivate convolutional filters for minimal performance decrease, (2) reactivate convolutional filters for maximal performance increase. We implement multiple Genetic Algorithms (GA) to perform each of these objectives- Downsize GA for best filter deactivations, while Upsize GA searches for best filter reactivations. By selective utilization of Upsize/Downsize GA, we could explicitly control the compression ratio of the model. For finalization, we fine-tune the compressed output model using the training dataset of the original input model. Our experimental results show that our method can reliably compress generative networks with minimal accuracy drop compared to other state-of-the-art compression algorithms.
更多查看译文
关键词
Genetic algorithms, Generative adversarial networks, Image coding, Statistics, Sociology, Computational modeling, Training, Evolutionary pruning, genetic algorithm, model compression
AI 理解论文
溯源树
样例
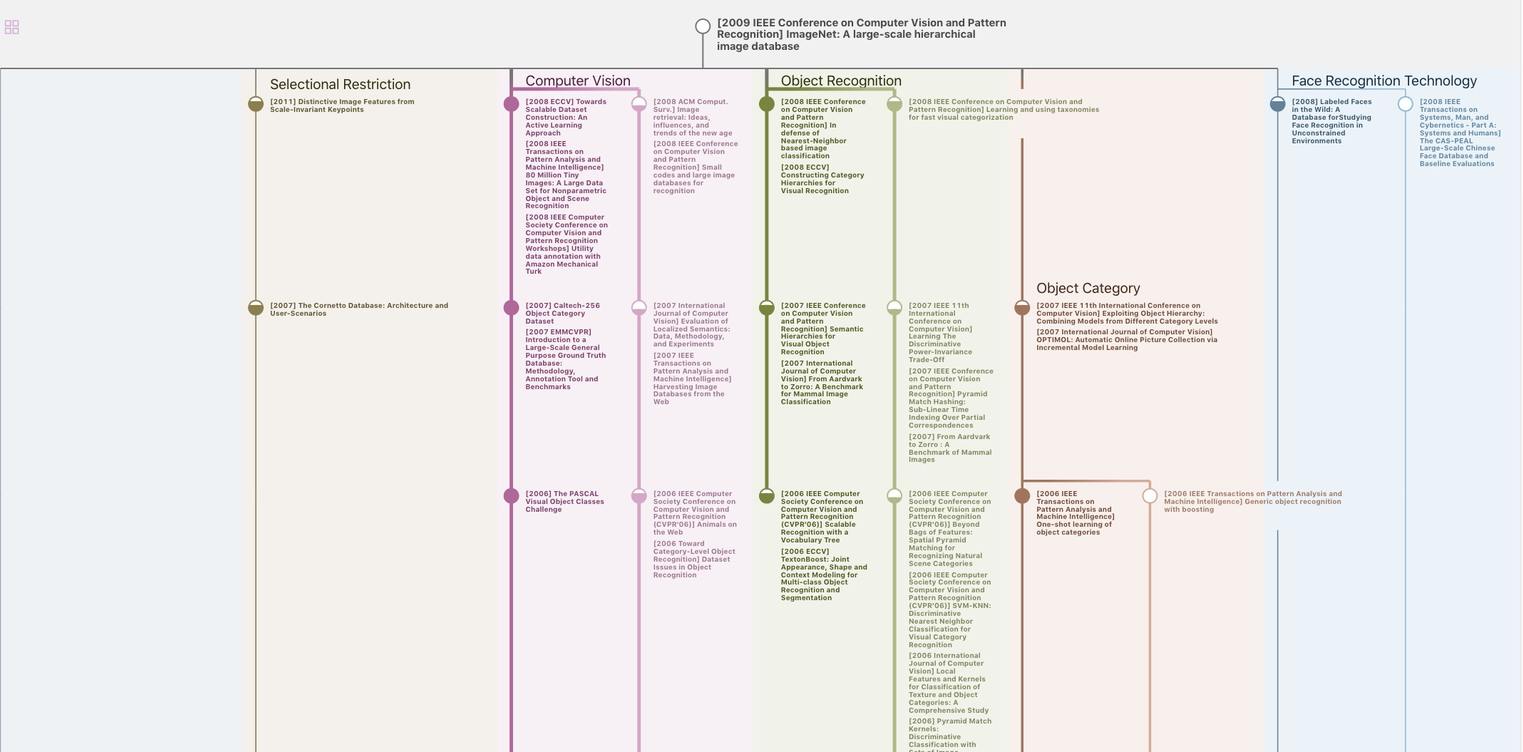
生成溯源树,研究论文发展脉络
Chat Paper
正在生成论文摘要