Multilingual, Code-switching and Low-Resource NLP and ASR
semanticscholar(2021)
AI Read Science
Must-Reading Tree
Example
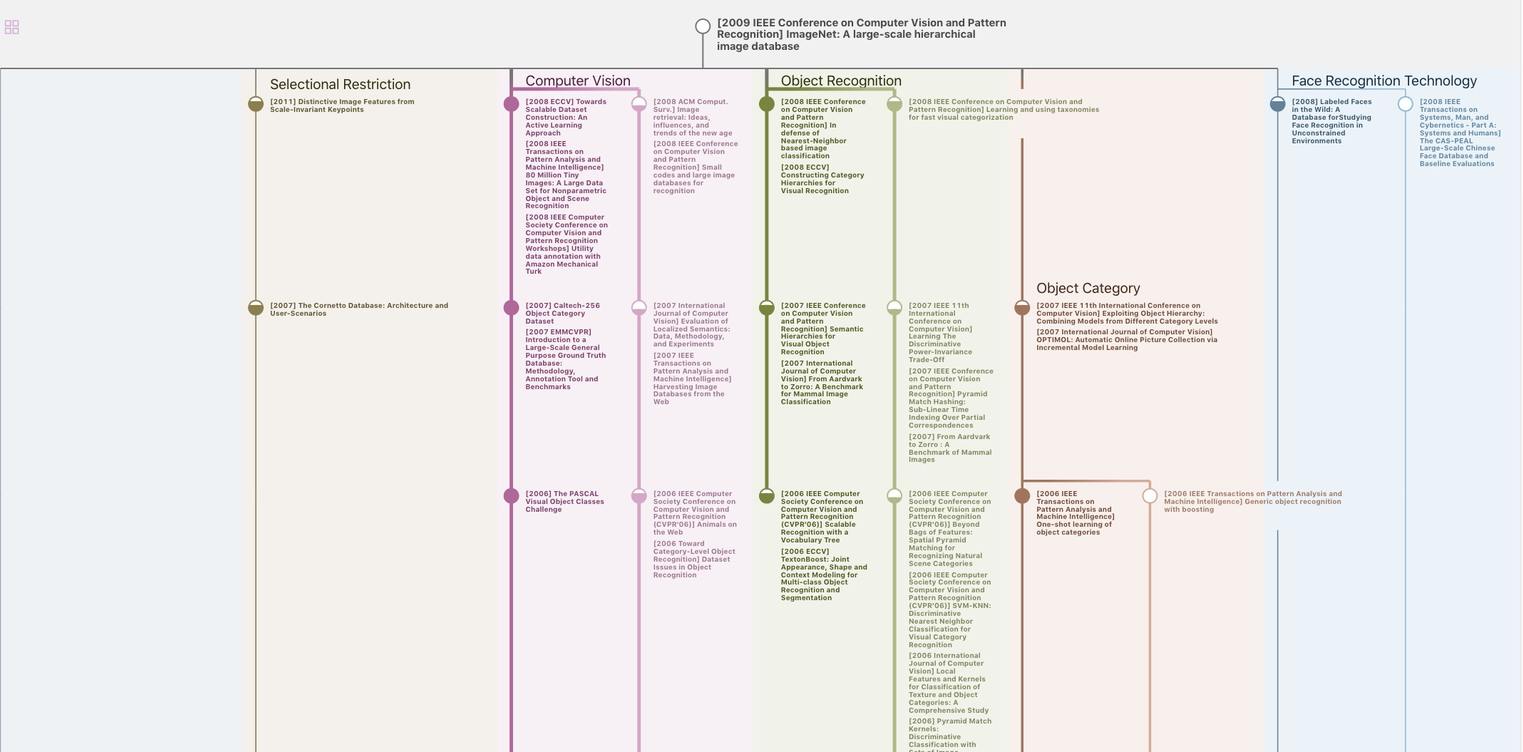
Generate MRT to find the research sequence of this paper
Chat Paper
Summary is being generated by the instructions you defined
semanticscholar(2021)