Real-Time Parameter Inference of Nonlinear Bluff-Body-Stabilized Flame Models Using Bayesian Neural Network Ensembles
PROCEEDINGS OF ASME TURBO EXPO 2023 TURBOMACHINERY TECHNICAL CONFERENCE AND EXPOSITION, GT2023, VOL 3A(2023)
Key words
Thermoacoustics,Neural Networks,Machine Learning,Data Assimilation,Flame Transfer Function
AI Read Science
Must-Reading Tree
Example
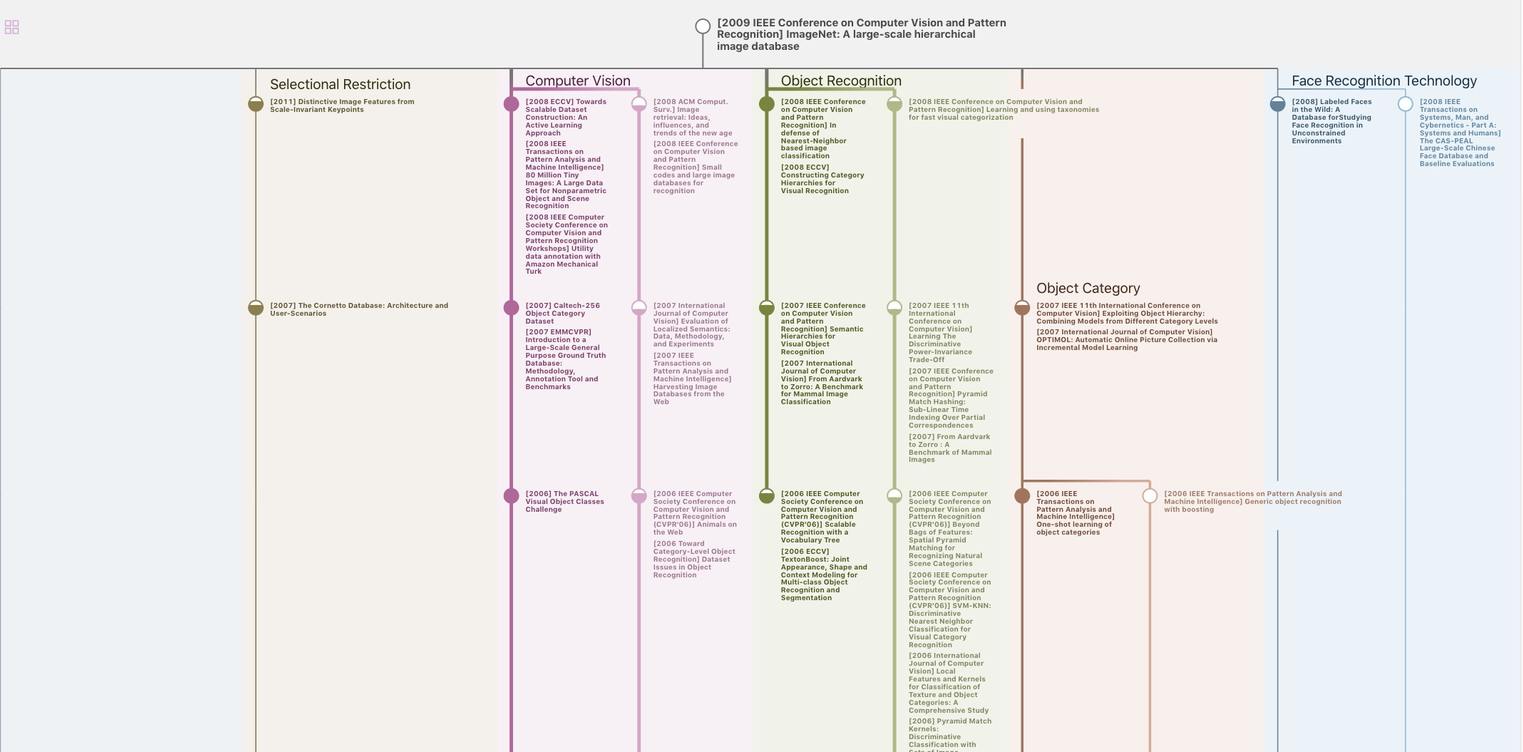
Generate MRT to find the research sequence of this paper
Chat Paper
Summary is being generated by the instructions you defined