Materials Structure–property Factorization for Identification of Synergistic Phase Interactions in Complex Solar Fuels Photoanodes
npj Computational Materials(2022)
关键词
Computational methods,Electrocatalysis,Materials Science,general,Characterization and Evaluation of Materials,Mathematical and Computational Engineering,Theoretical,Mathematical and Computational Physics,Computational Intelligence,Mathematical Modeling and Industrial Mathematics
AI 理解论文
溯源树
样例
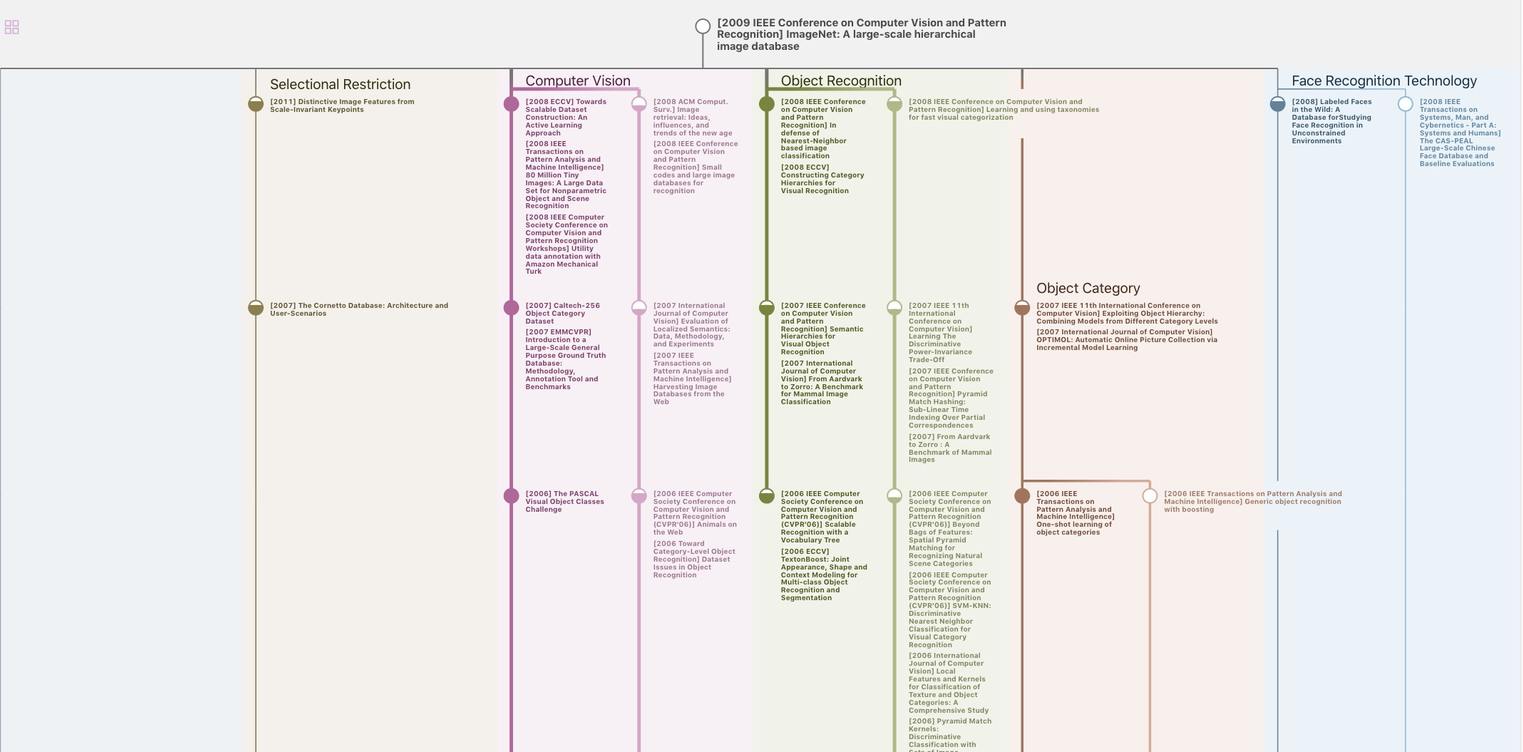
生成溯源树,研究论文发展脉络
Chat Paper
正在生成论文摘要