Exploring How Anomalous Model Input and Output Alerts Affect Decision-Making in Healthcare
arxiv(2022)
摘要
An important goal in the field of human-AI interaction is to help users more appropriately trust AI systems' decisions. A situation in which the user may particularly benefit from more appropriate trust is when the AI receives anomalous input or provides anomalous output. To the best of our knowledge, this is the first work towards understanding how anomaly alerts may contribute to appropriate trust of AI. In a formative mixed-methods study with 4 radiologists and 4 other physicians, we explore how AI alerts for anomalous input, very high and low confidence, and anomalous saliency-map explanations affect users' experience with mockups of an AI clinical decision support system (CDSS) for evaluating chest x-rays for pneumonia. We find evidence suggesting that the four anomaly alerts are desired by non-radiologists, and the high-confidence alerts are desired by both radiologists and non-radiologists. In a follow-up user study, we investigate how high- and low-confidence alerts affect the accuracy and thus appropriate trust of 33 radiologists working with AI CDSS mockups. We observe that these alerts do not improve users' accuracy or experience and discuss potential reasons why.
更多查看译文
关键词
output alerts affect
AI 理解论文
溯源树
样例
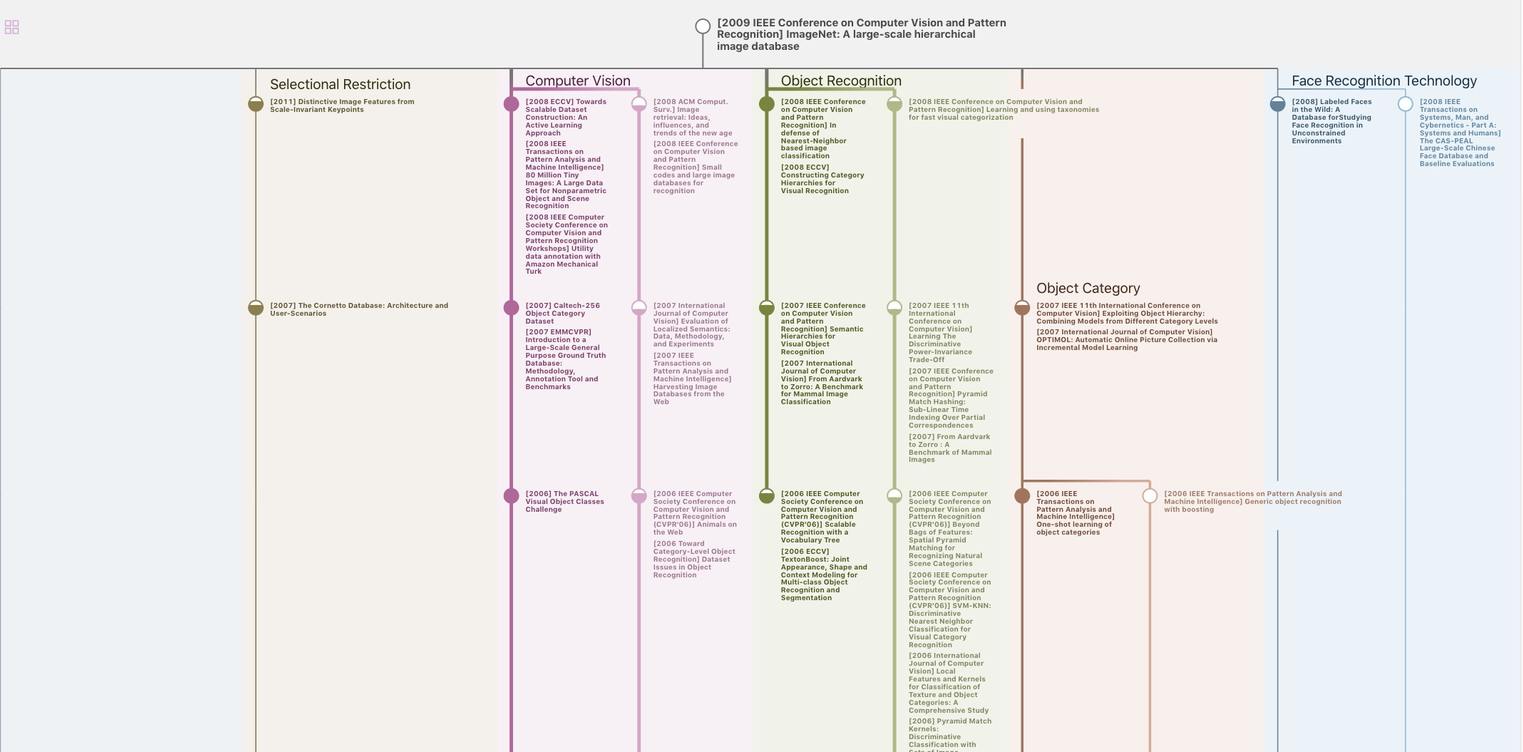
生成溯源树,研究论文发展脉络
Chat Paper
正在生成论文摘要