Learning Performance Graphs from Demonstrations Via Task-Based Evaluations
IEEE Robotics and Automation Letters(2022)
Univ Southern Calif
Abstract
In the paradigm of robot learning-from-demonstra tions (LfD), understanding and evaluating the demonstrated behaviors plays a critical role in extracting control policies for robots. Without this knowledge, a robot may infer incorrect reward functions that lead to undesirable or unsafe control policies. Prior work has used temporal logic specifications, manually ranked by human experts based on their importance, to learn reward functions from imperfect/suboptimal demonstrations. To overcome reliance on expert rankings, we propose a novel algorithm that learns from demonstrations, a partial ordering of provided specifications in the form of a performance graph. Through various experiments, including simulation of industrial mobile robots, we show that extracting reward functions with the learned graph results in robot policies similar to those generated with the manually specified orderings. We also show in a user study that the learned orderings match the orderings or rankings by participants for demonstrations in a simulated driving domain. These results show that we can accurately evaluate demonstrations with respect to provided task specifications from a small set of imperfect data with minimal expert input.
MoreTranslated text
Key words
Formal methods in robotics and automation,learning from demonstration,reinforcement learning
PDF
View via Publisher
AI Read Science
Must-Reading Tree
Example
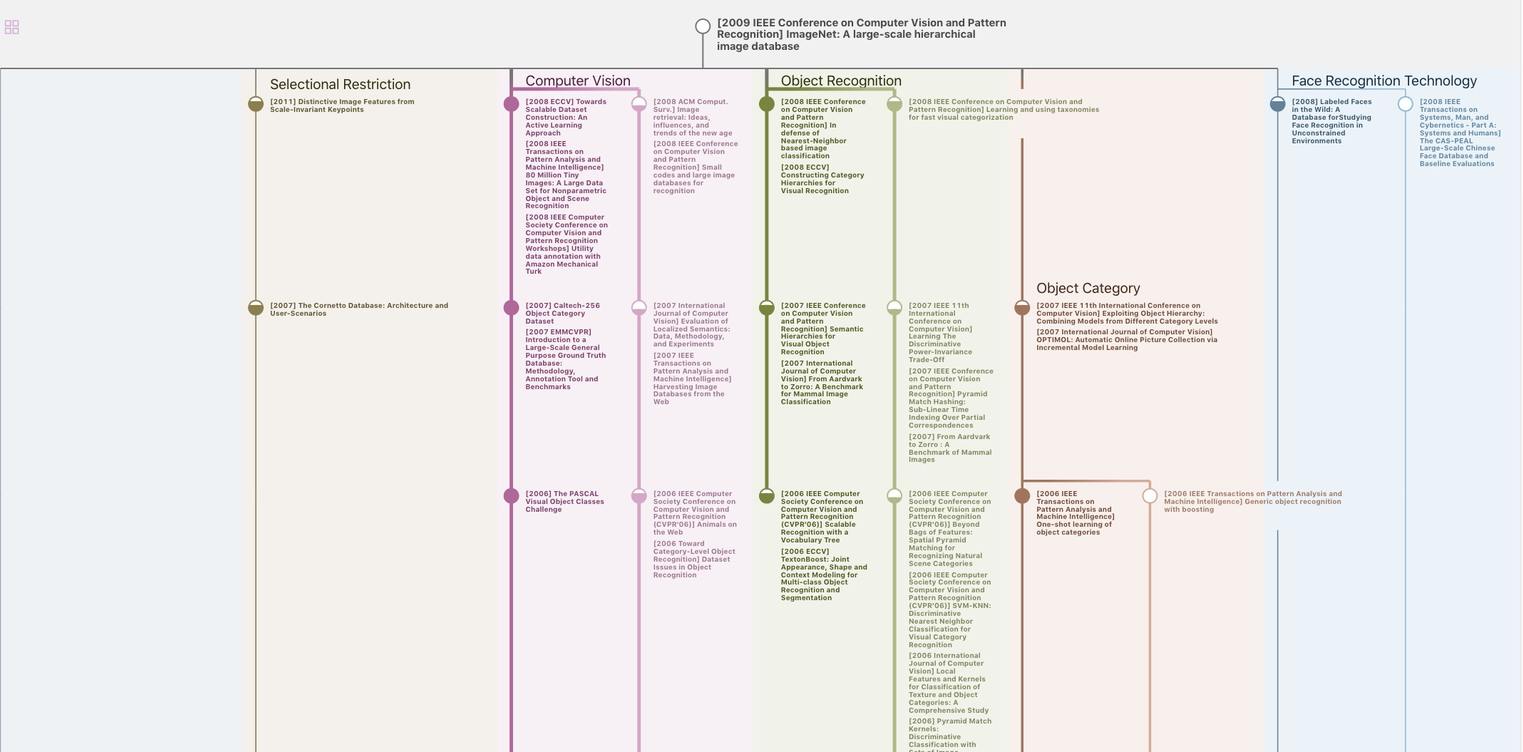
Generate MRT to find the research sequence of this paper
Data Disclaimer
The page data are from open Internet sources, cooperative publishers and automatic analysis results through AI technology. We do not make any commitments and guarantees for the validity, accuracy, correctness, reliability, completeness and timeliness of the page data. If you have any questions, please contact us by email: report@aminer.cn
Chat Paper
Summary is being generated by the instructions you defined