Gram Regularization for Sparse and Disentangled Representation
Pattern Analysis and Applications(2022)
关键词
Regularization,Sparse representation,Disentangled representation,Decision margin
AI 理解论文
溯源树
样例
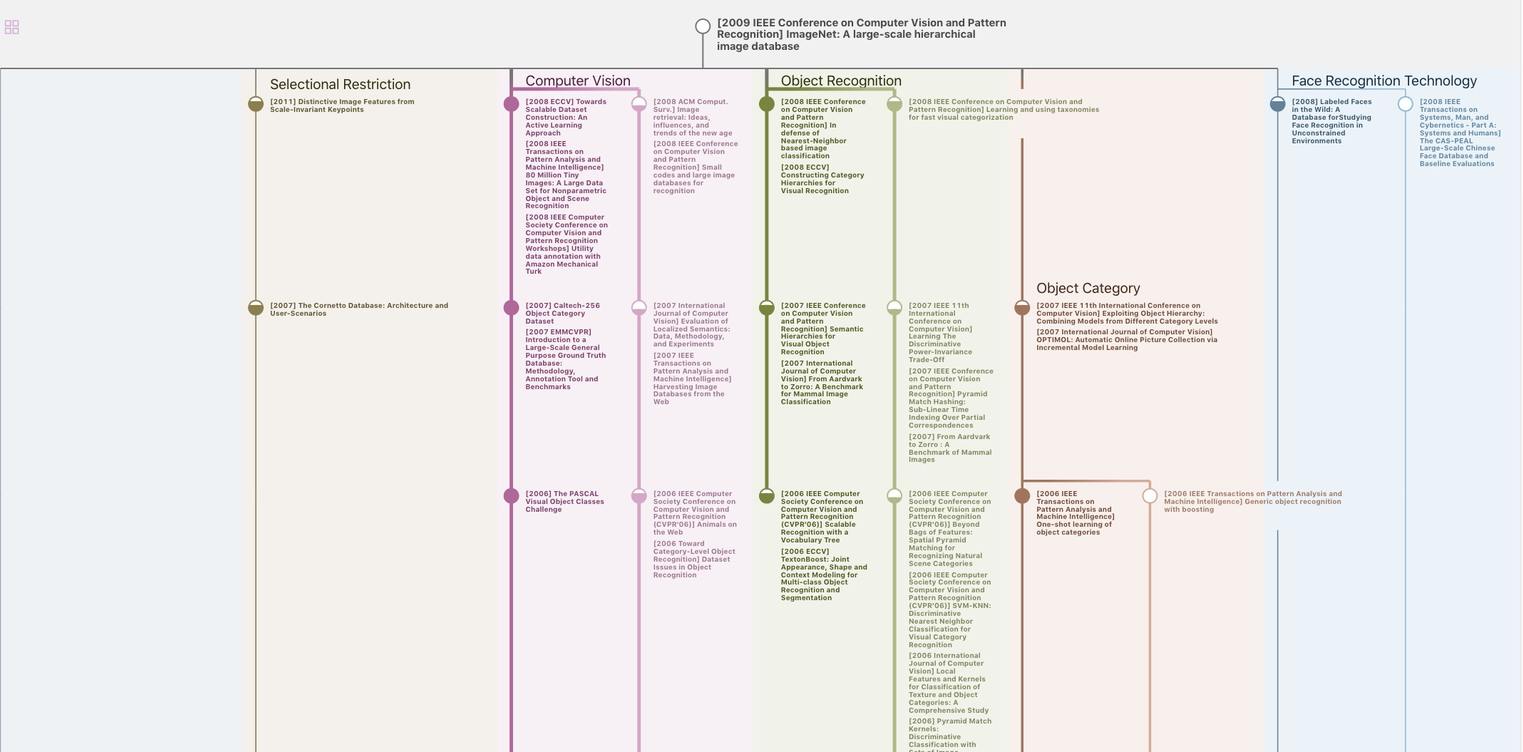
生成溯源树,研究论文发展脉络
Chat Paper
正在生成论文摘要
Pattern Analysis and Applications(2022)