Abstract WP230: Interpretable Machine Learning Models for Predicting 30-Day All-Cause Readmission Following Carotid Endarterectomy among Acute Ischemic Stroke Cases: A NSQIP Study (2014 - 2017)
Stroke(2022)
AI 理解论文
溯源树
样例
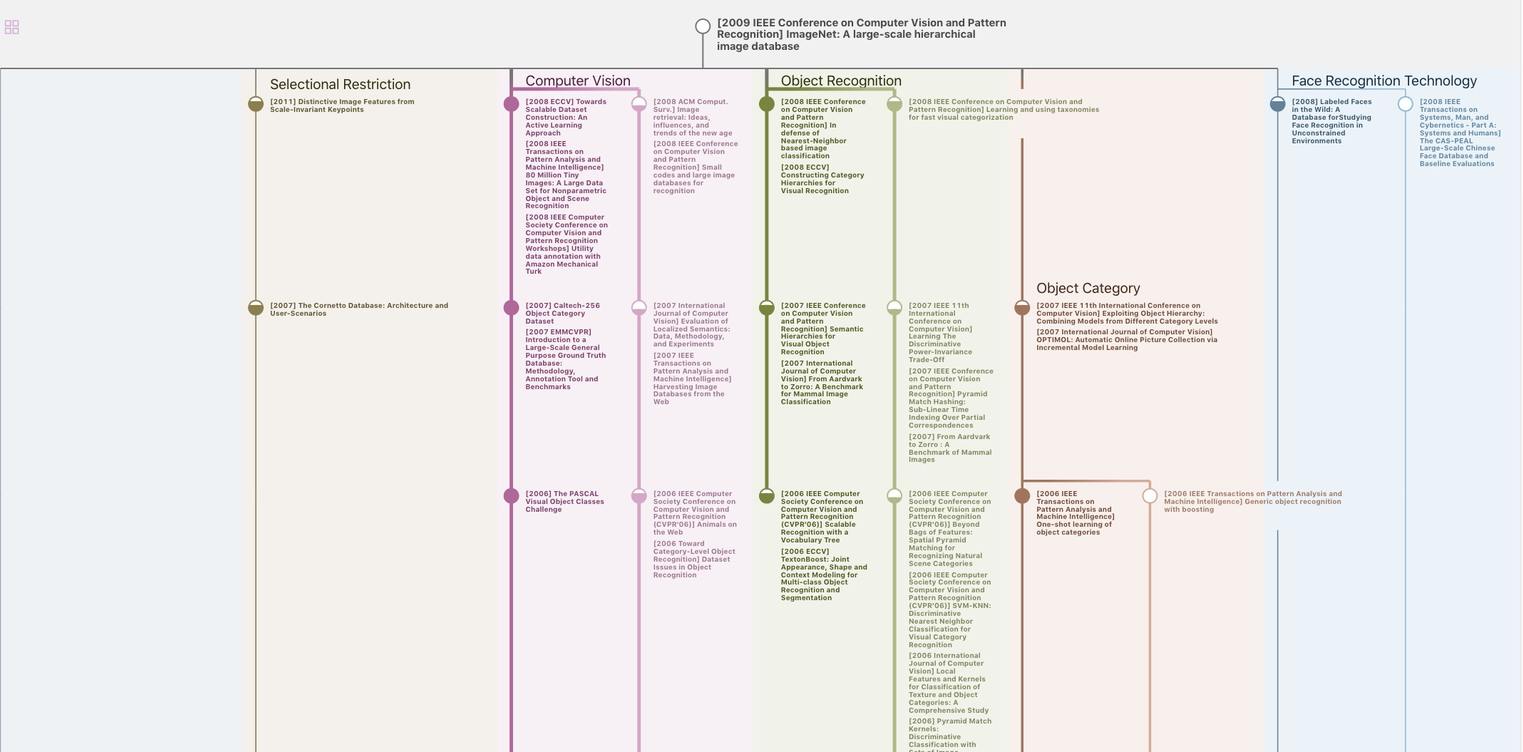
生成溯源树,研究论文发展脉络
Chat Paper
正在生成论文摘要