Time Series Anomaly Detection with Adversarial Reconstruction Networks
IEEE TRANSACTIONS ON KNOWLEDGE AND DATA ENGINEERING(2023)
关键词
Time series,adversarial reconstruction networks,anomaly detection,data augmentation
AI 理解论文
溯源树
样例
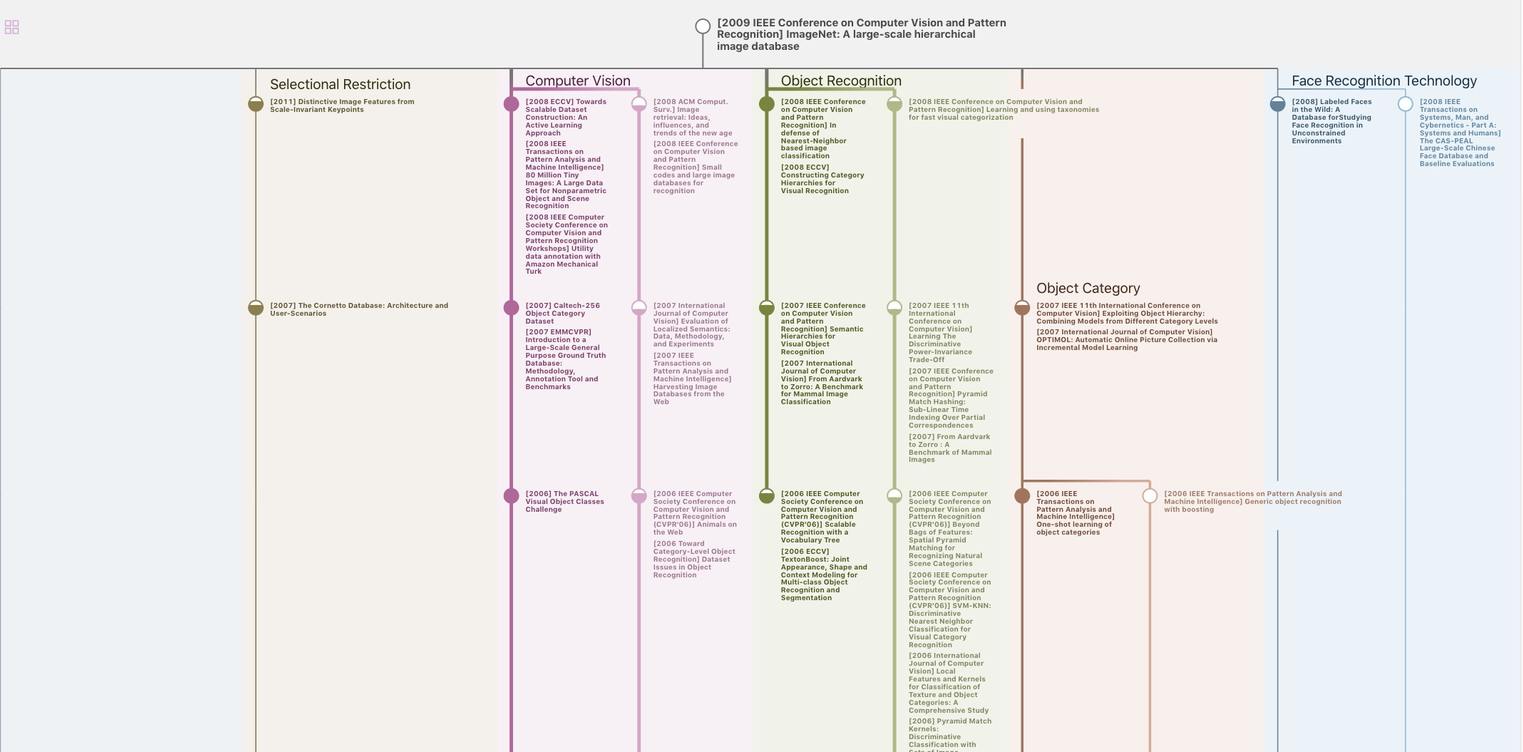
生成溯源树,研究论文发展脉络
Chat Paper
正在生成论文摘要