Contrastive Graph Representations for Logical Formulas Embedding
IEEE TRANSACTIONS ON KNOWLEDGE AND DATA ENGINEERING(2023)
关键词
Syntactics,Semantics,Task analysis,Visualization,Transforms,Recurrent neural networks,Deep learning,Formulas embedding,graph representation,contrastive learning,graph convolutional network
AI 理解论文
溯源树
样例
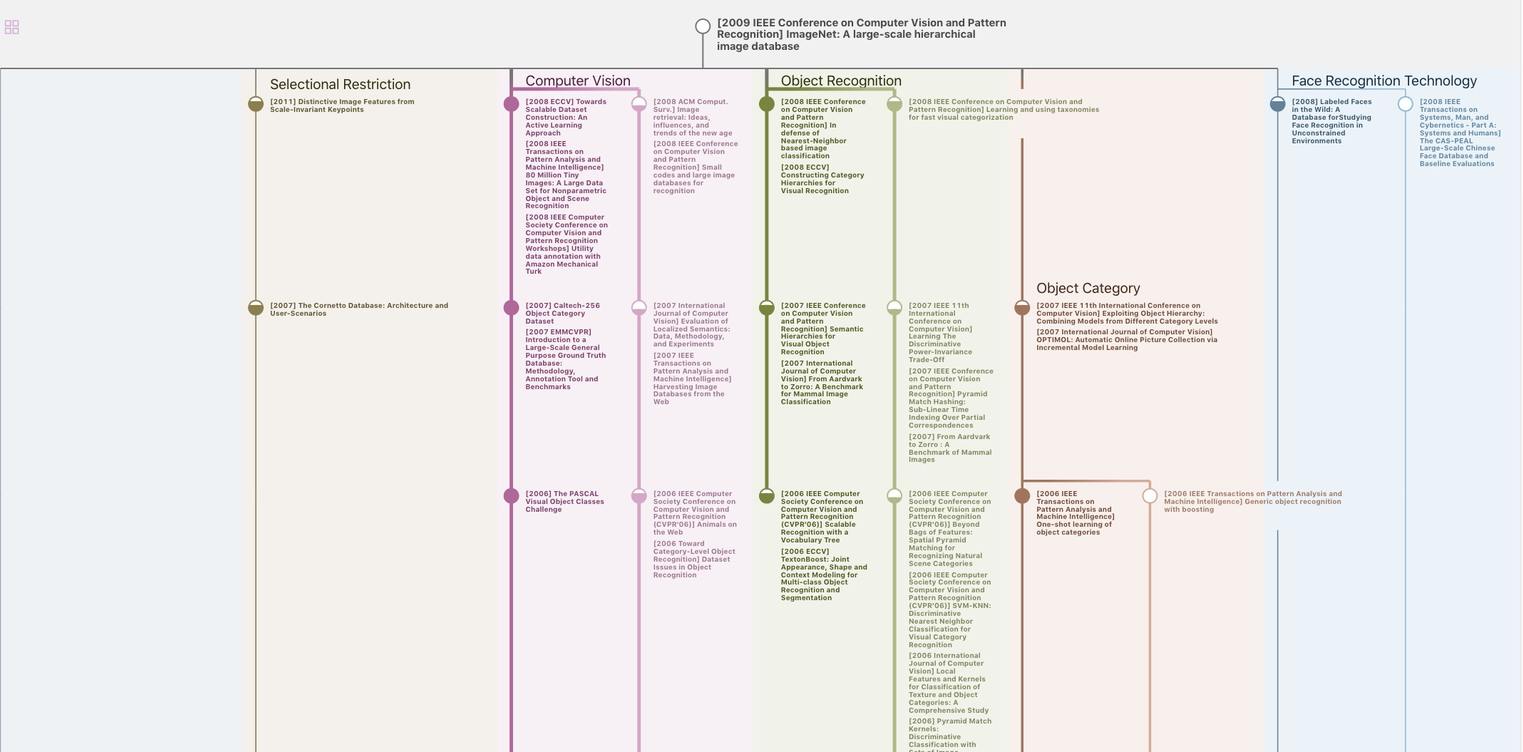
生成溯源树,研究论文发展脉络
Chat Paper
正在生成论文摘要