Remaining Useful Life Prediction for Turbofan Engine using SAE-TCN Model
chinese control conference(2021)
摘要
Turbofan engines are known as the heart of the aircraft, as important equipment of the aircraft, the health state of the engine determines the aircraft’s operational status. Therefore, the equipment monitoring and maintenance of the engine is an important part of ensuring the healthy and stable operation of the aircraft, and the remaining useful life (RUL) prediction of the engine is an important part of it. The monitoring data of turbofan engines have a high dimension and a long time span, which brings difficulties to predicting the remaining useful life of the engine. This paper proposes a residual life prediction model based on Autoencoder and temporal convolutional network (TCN). Among them, Autoencoder is used to reduce the dimension of the data and extract features from the engine monitoring data. The obtained low-dimensional data is trained in the TCN network to predict the remaining useful life. The model mentioned in this article is verified on the NASA public dataset(C-MAPSS) and compared with common machine learning methods and other deep neural networks. The experimental results show that the model proposed in this paper performs best in the evaluation methods, and this conclusion has important implications for engine health.
更多查看译文
关键词
Deep Learning,Turbofan Engine,Remaining Useful Life Prediction,Temporal Convolutional Network,Autoencoder,Target Generation
AI 理解论文
溯源树
样例
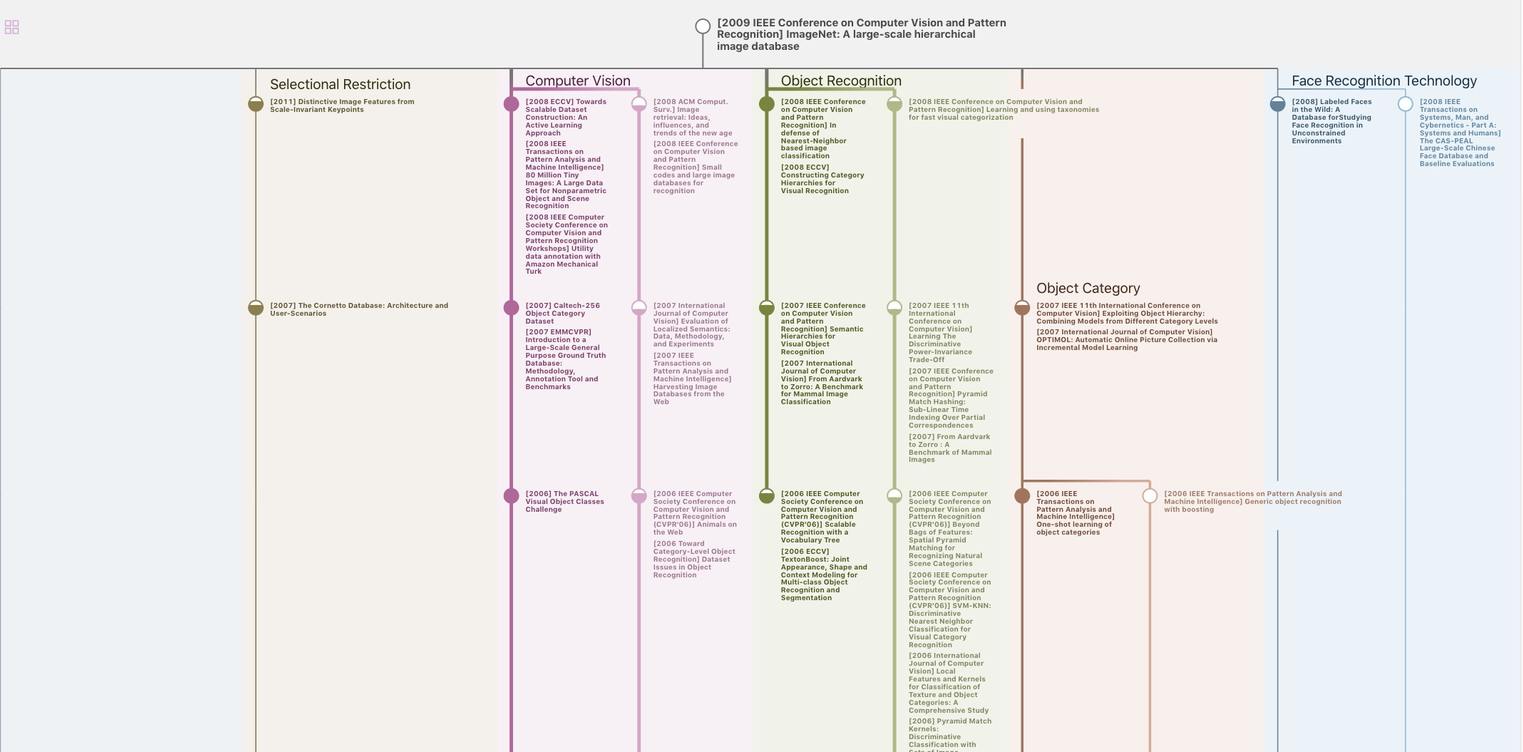
生成溯源树,研究论文发展脉络
Chat Paper
正在生成论文摘要