Micro-Genetic algorithm with fuzzy selection of operators for multi-Objective optimization: μ FAME
Swarm and Evolutionary Computation(2021)
摘要
We propose a new accurate Micro Genetic Algorithm (μGA) for multi-objective optimization problems that we call Micro-FAME (or μFAME). The distinctive feature of μFAME with respect to the other existing multi-objective algorithms in the literature is its high elitism and fast convergence, produced by the application of the evolution directly on the Pareto front approximation. This means that it has a bounded population of variable size, determined by the number of non-dominated solutions found so far. In μFAME (and generally in μGAs) the premature convergence problem acquires especial relevance, and generating and maintaining solutions diversity is of extreme importance to deal with it. This is especially significant in the multi-objective field, where the algorithm looks for a wide and diverse set of non-dominated solutions. In μFAME, the diversity loss that high elitism usually produces is overcome by the decisions driven by the Fuzzy Inference System (FIS), that chooses the most appropriate operators to apply during the evolution to promote both diversity and accuracy of solutions. The algorithm arises as a suitable solution for problems with a computationally heavy fitness function, because it is able to quickly achieve good quality local optimal solutions, but it is also highly competitive in long runs. Experiments reveal a great performance of μFAME according to several convergence related metrics, when comparing against seven state-of-the-art algorithms on a large set of benchmark problems, as well as on a real-world engineering one with an expensive fitness function.
更多查看译文
关键词
fuzzy selection,optimization,algorithm,xmlnsmml=http//wwww3org/1998/math/mathml,micro-genetic,multi-objective
AI 理解论文
溯源树
样例
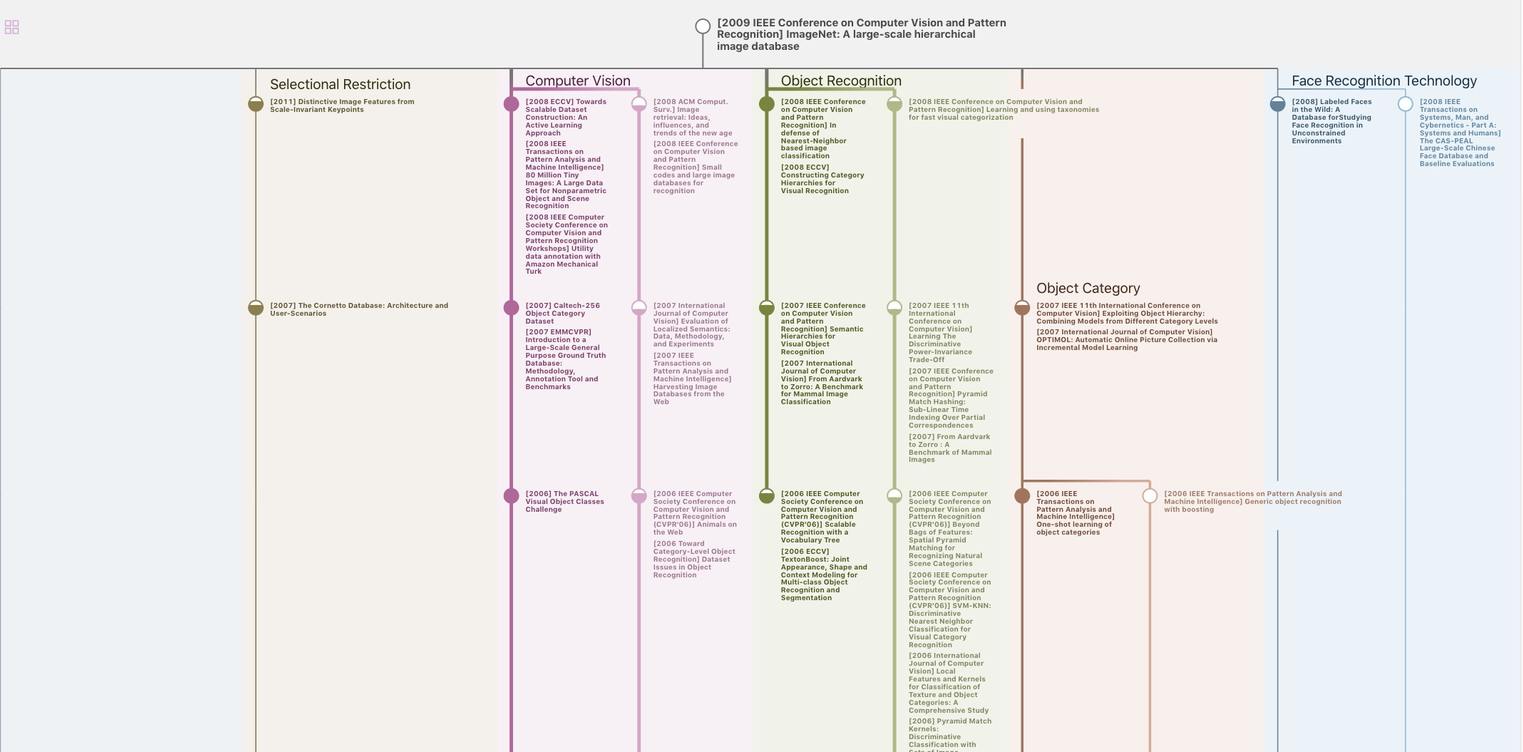
生成溯源树,研究论文发展脉络
Chat Paper
正在生成论文摘要