Transformer Memory as a Differentiable Search Index
NeurIPS 2022(2022)
摘要
In this paper, we demonstrate that information retrieval can be accomplished with a single Transformer, in which all information about the corpus is encoded in the parameters of the model. To this end, we introduce the Differentiable Search Index (DSI), a new paradigm that learns a text-to-text model that maps string queries directly to relevant docids; in other words, a DSI model answers queries directly using only its parameters, dramatically simplifying the whole retrieval process. We study variations in how documents and their identifiers are represented, variations in training procedures, and the interplay between models and corpus sizes. Experiments demonstrate that given appropriate design choices, DSI significantly outperforms strong baselines such as dual encoder models. Moreover, DSI demonstrates strong generalization capabilities, outperforming a BM25 baseline in a zero-shot setup.
更多查看译文
关键词
transformer,memory,index,search
AI 理解论文
溯源树
样例
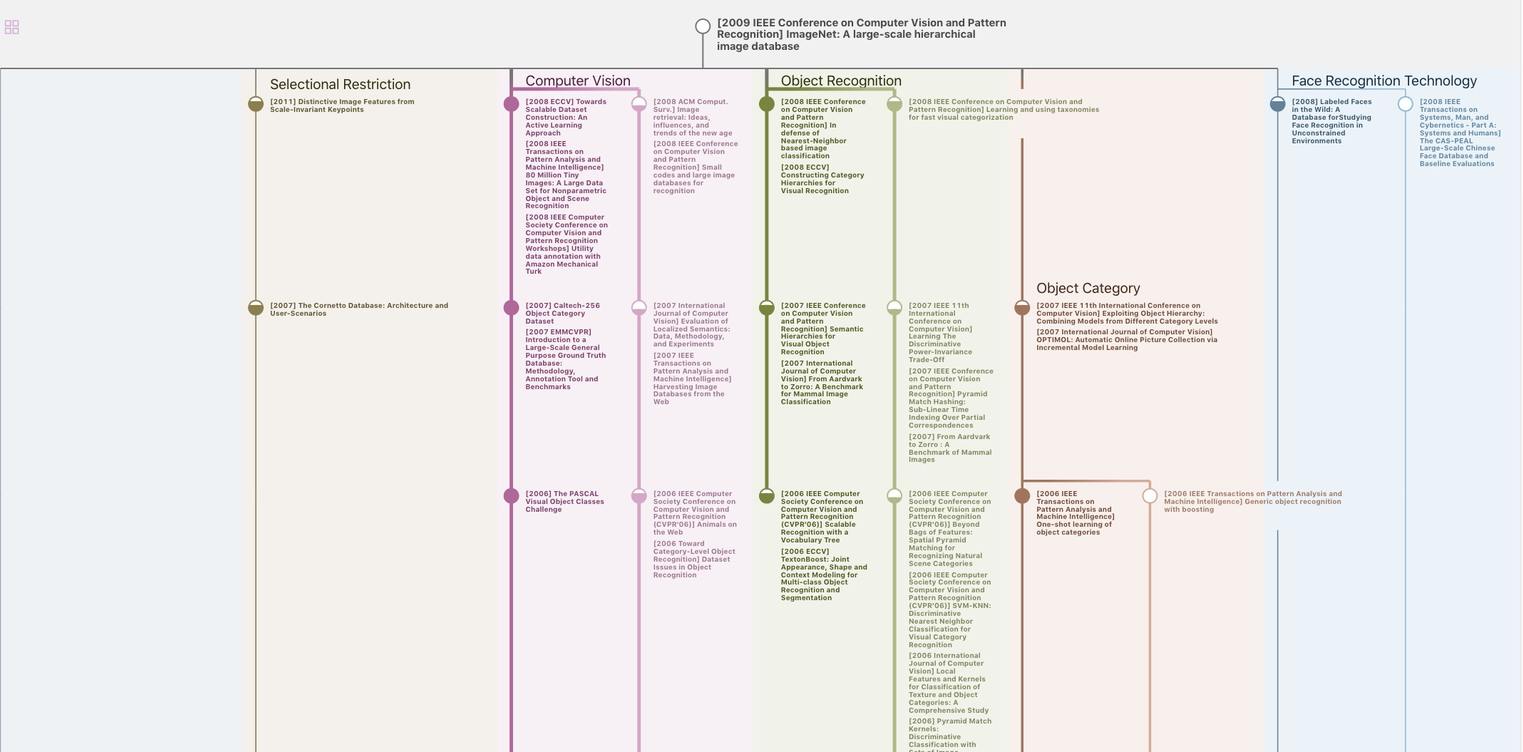
生成溯源树,研究论文发展脉络
Chat Paper
正在生成论文摘要