Learning from Randomly Initialized Neural Network Features
arxiv(2022)
摘要
We present the surprising result that randomly initialized neural networks are good feature extractors in expectation. These random features correspond to finite-sample realizations of what we call Neural Network Prior Kernel (NNPK), which is inherently infinite-dimensional. We conduct ablations across multiple architectures of varying sizes as well as initializations and activation functions. Our analysis suggests that certain structures that manifest in a trained model are already present at initialization. Therefore, NNPK may provide further insight into why neural networks are so effective in learning such structures.
更多查看译文
关键词
learning,randomly,features,network
AI 理解论文
溯源树
样例
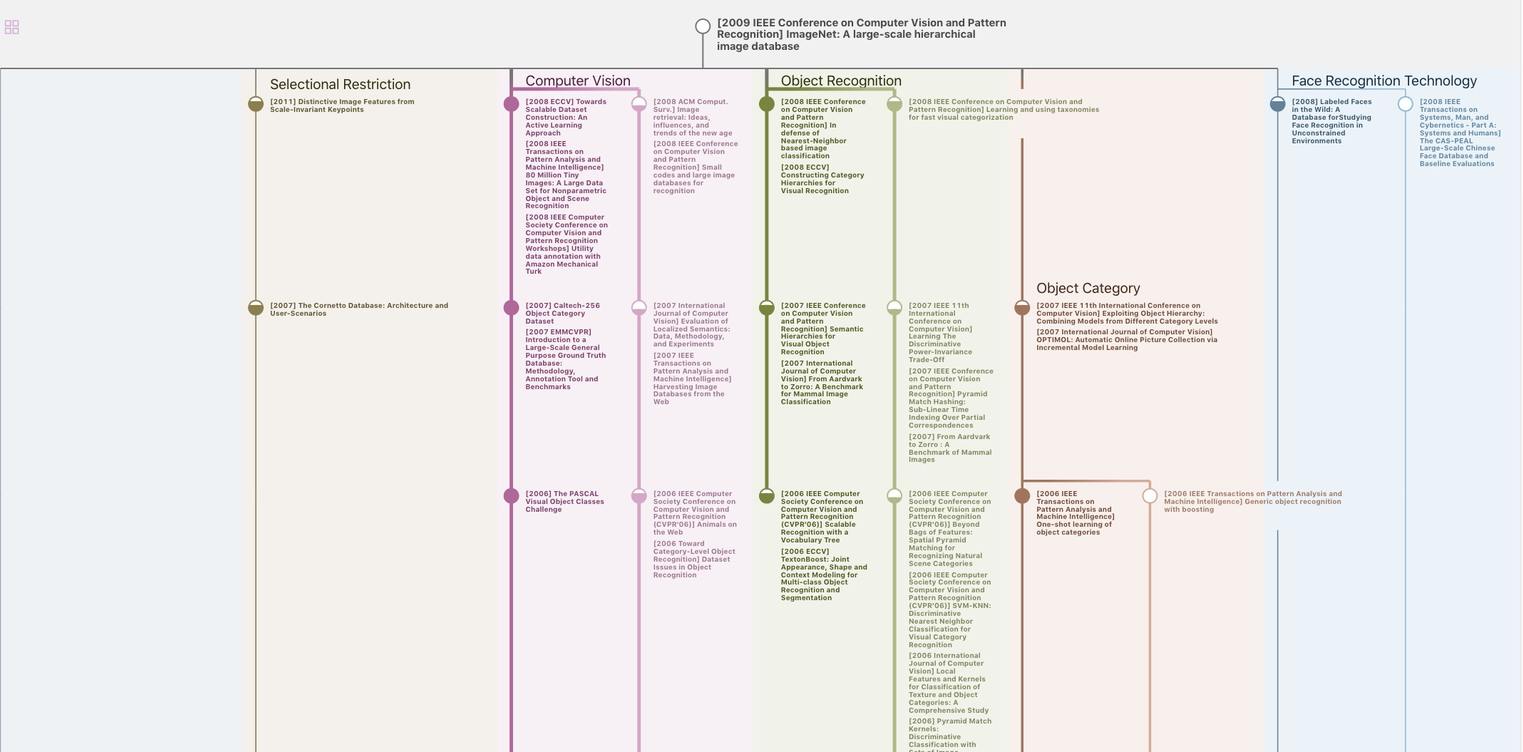
生成溯源树,研究论文发展脉络
Chat Paper
正在生成论文摘要