NonLoss: a Novel Analytical Method for Differential Biological Module Identification from Single-Cell Transcriptome
Annals of Translational Medicine(2021)
关键词
Single-cell RNA sequencing (scRNA-seq),biological module,blood,colorectal cancer,python package
AI 理解论文
溯源树
样例
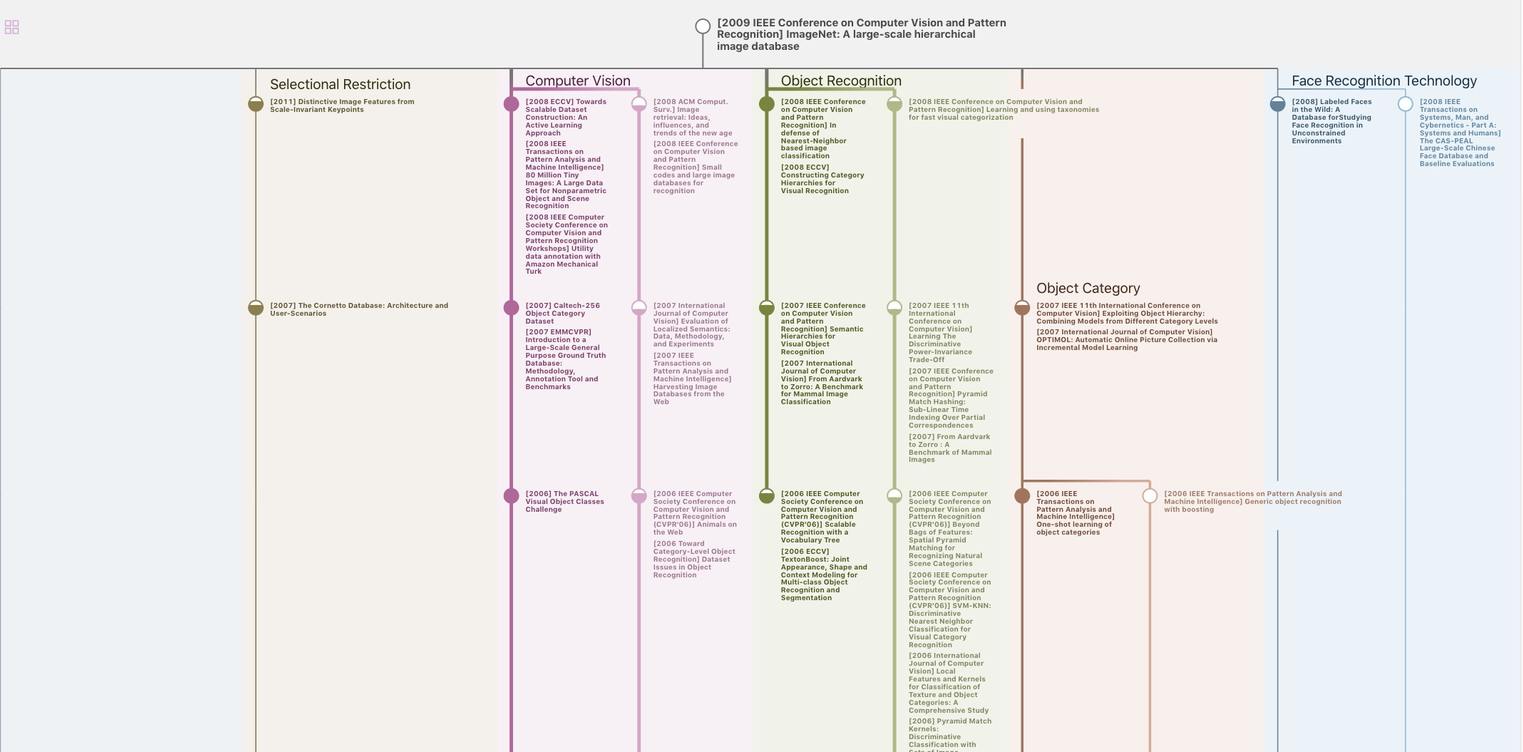
生成溯源树,研究论文发展脉络
Chat Paper
正在生成论文摘要