Variety classification of coated maize seeds based on Raman hyperspectral imaging.
Spectrochimica acta. Part A, Molecular and biomolecular spectroscopy(2021)
摘要
As an essential factor in quality assessment of maize seeds, variety purity profoundly impacts final yield and farmers' economic benefits. In this study, a novel method based on Raman hyperspectral imaging system was applied to achieve variety classification of coated maize seeds. A total of 760 maize seeds including 4 different varieties were evaluated. Raman spectral data of 400-1800 cm-1 were extracted and preprocessed. Variable selection methods involved were modified competitive adaptive reweighted sampling (MCARS), successive projections algorithm (SPA), and their combination. In addition, MCARS was proposed for the first time in this paper as a stable search technology. The performance of support vector machine (SVM) models optimized by genetic algorithm (GA) was analyzed and compared with models based on random forest (RF) and back-propagation neural network (BPNN). Same models based on Vis-NIR spectral data were also established for comparison. Results showed that the MCARS-GA-SVM model based on Raman spectral data obtained the best performance with calibration accuracy of 99.29% and prediction accuracy of 100%, which were stable and easily replicated. In addition, the accuracy on the independent validation set was 96.88%, which proved that the model can be applied in practice. A more simplified MCARS-SPA-GA-SVM model, which contained only 3 variables, had more than 95% accuracy on each data set. This procedure can help to develop a real-time detection system to classify coated seed varieties with high accuracy, which is of great significance for assessing variety purity and increasing crop yield.
更多查看译文
AI 理解论文
溯源树
样例
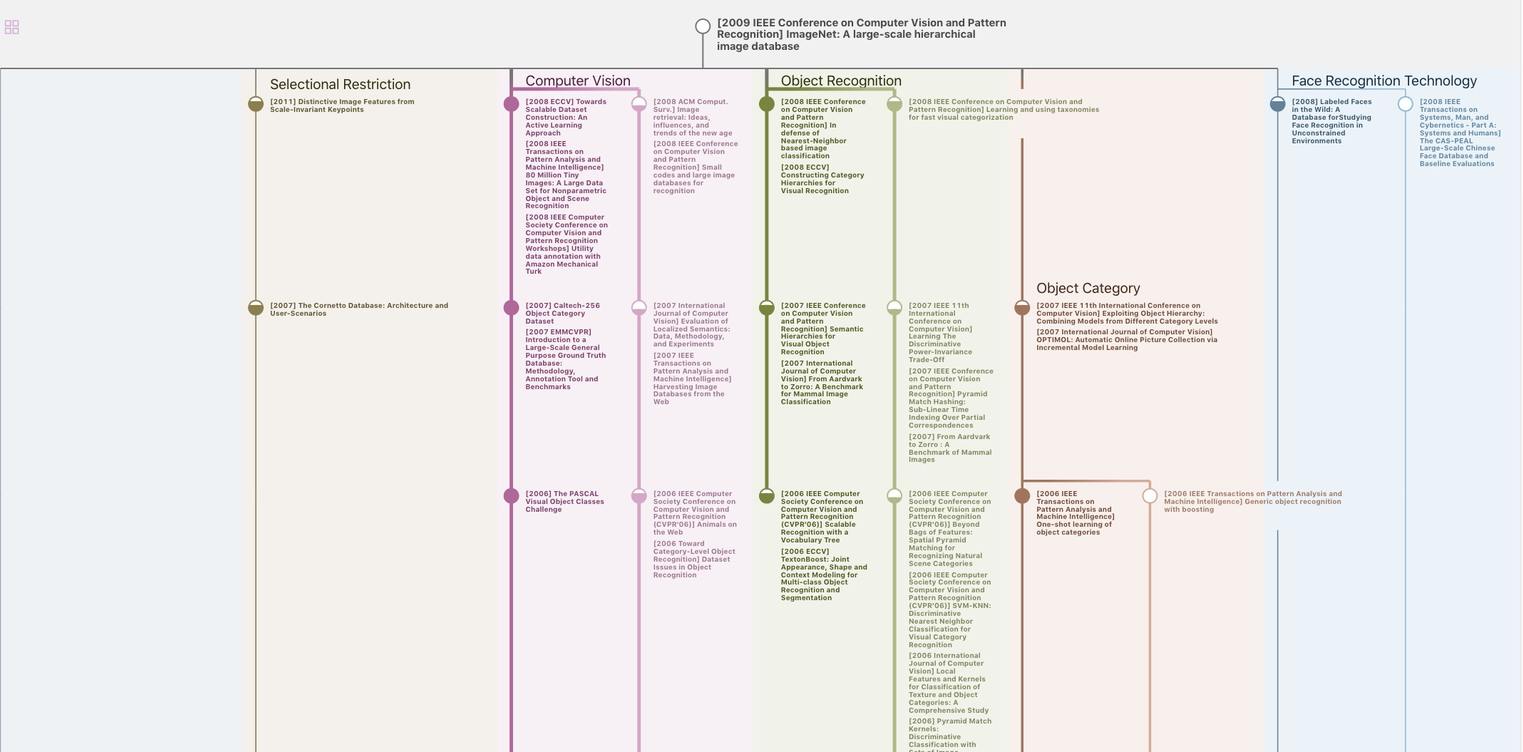
生成溯源树,研究论文发展脉络
Chat Paper
正在生成论文摘要