A Classification System for Visualized Malware Based on Multiple Autoencoder Models
IEEE ACCESS(2021)
摘要
In this paper, we propose a classification system that uses multiple autoencoder models for identifying malware images. It is crucial to accurately classify malware before we can deploy appropriate countermeasures to prevent them from spreading. Rapid malware classification is the first step in preparing effective countermeasures. Typical approaches to this problem, which can be divided into static or dynamic methods, are not suitable for efficient malware classification because they require either fixed malware patterns or lots of time to investigate, respectively. If the malware analysts have enough time and resources, they can analyze any malware thoroughly. However, finite resources mean they always suffer from a lack of time due to the malware that needs analyzing increasing at a dramatic rate. In the real world, new malware and variants of existing malware are constantly emerging. To address this issue, many researchers have developed approaches using machine learning techniques. However, to date these systems have had difficulty responding appropriately to the rapidly changing malware environment and also suffer from data imbalance problems in the training data. The system proposed in this paper consists of multiple autoencoder models that classify malware that has been converted to an image. Each autoencoder model classifies only one type of malware and is trained using only samples from the corresponding family, this allows the system to update quickly and mitigates the data imbalance problem. We demonstrate our method's superior performance through various experiments compared to other state-of-the-art techniques using the Malimg dataset.
更多查看译文
关键词
Malware,Feature extraction,Deep learning,Machine learning,Machine learning algorithms,Data visualization,Task analysis,Autoencoder,deep learning,malware classification,malware visualization
AI 理解论文
溯源树
样例
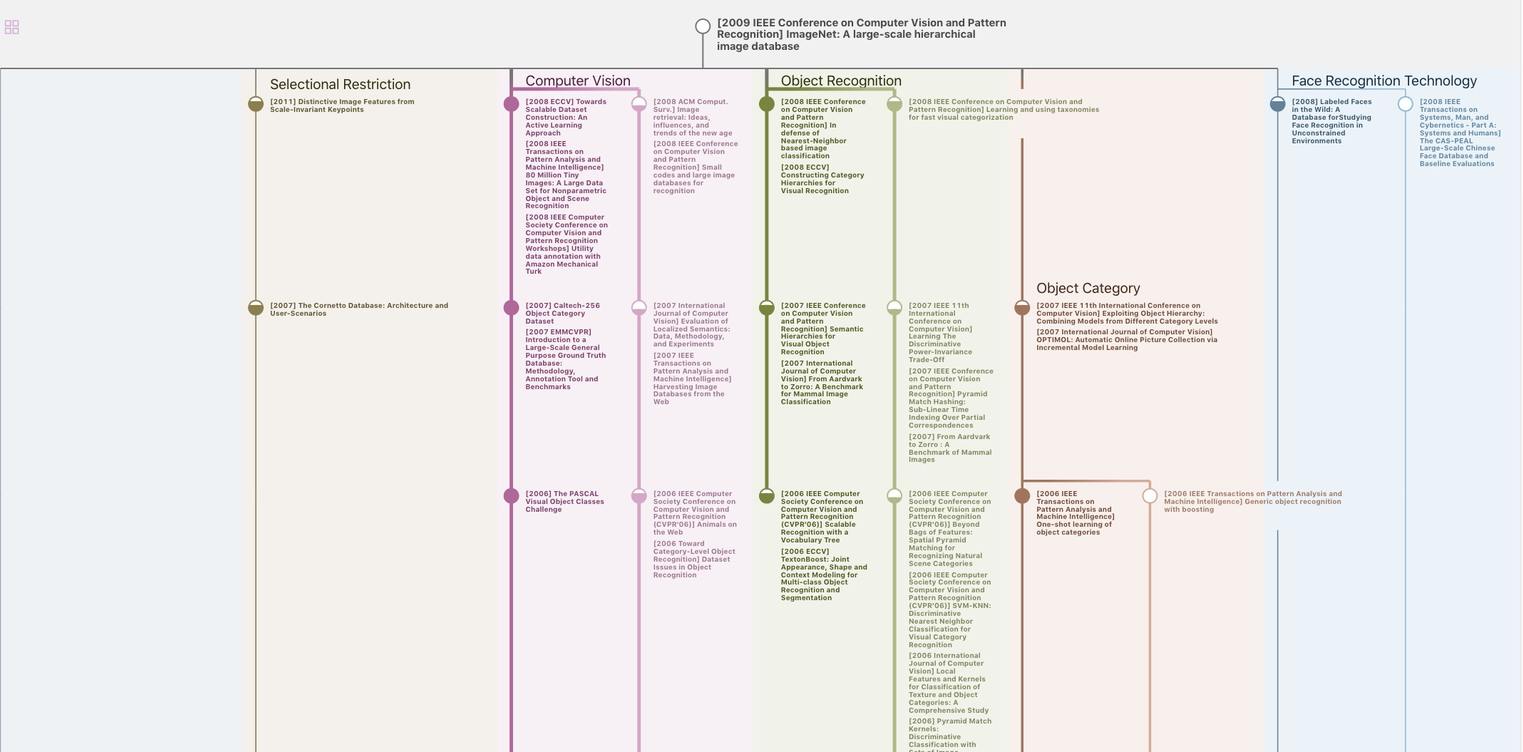
生成溯源树,研究论文发展脉络
Chat Paper
正在生成论文摘要